Data Labeling for Geospatial Mapping
Project Overview:
Objective
The “Data Labeling for Geospatial Mapping” project aims to create a dataset for training machine learning models to accurately label and annotate geospatial data, such as satellite imagery, maps, and aerial photographs. This dataset will support geospatial mapping applications, land use analysis, urban planning, and environmental monitoring.
Scope
This project involves collecting geospatial data from various sources, including satellite imagery providers, government agencies, and mapping platforms, and annotating them with relevant information, such as land cover types, infrastructure, and geographic features.
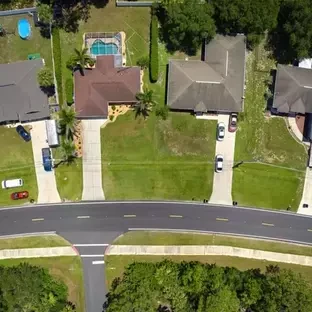
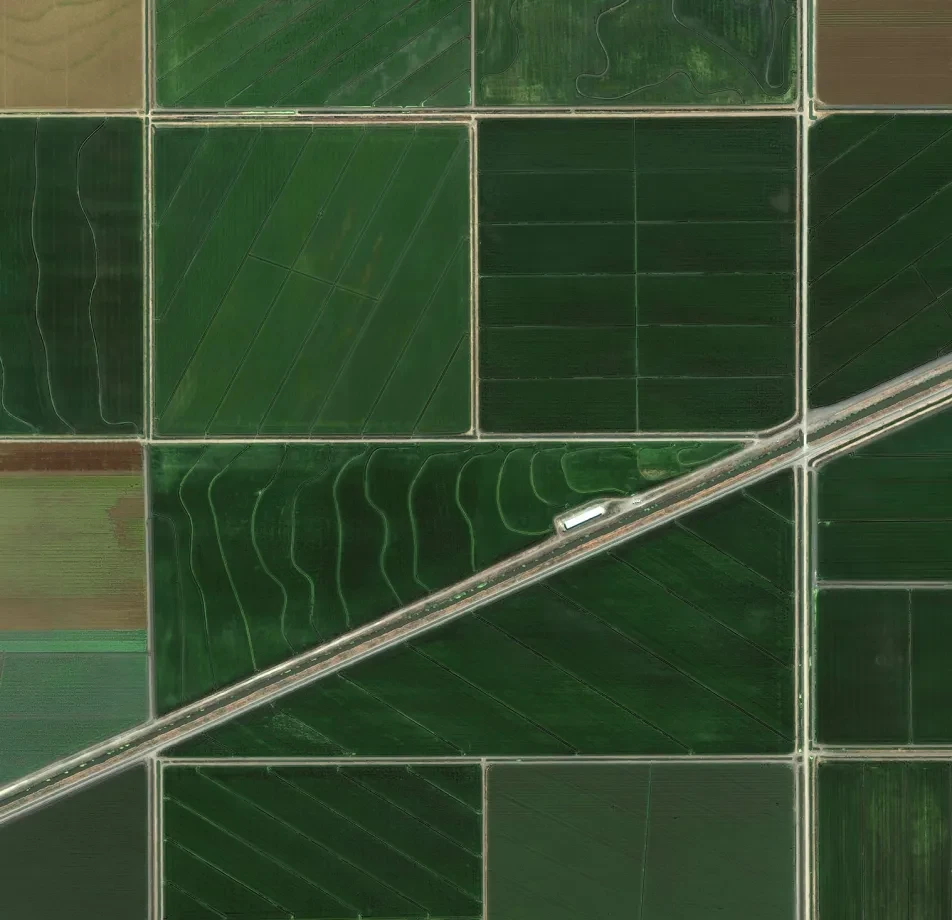
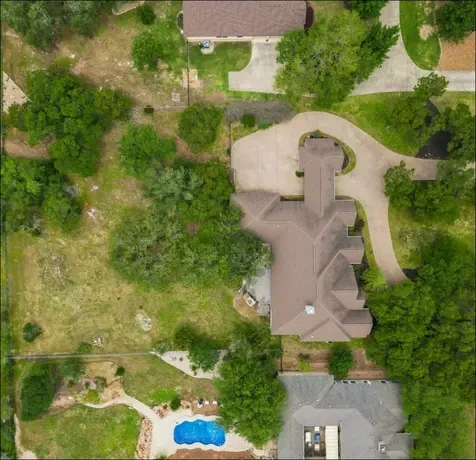
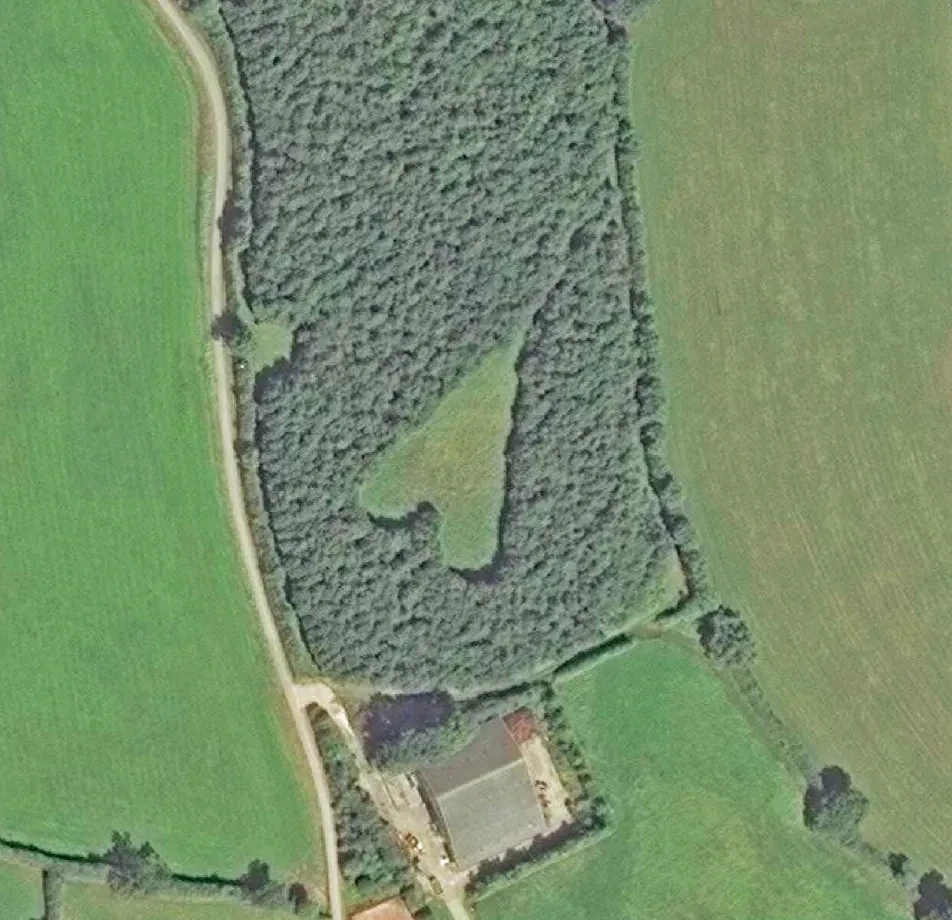
Sources
- Satellite Imagery: Gather high-resolution satellite imagery from commercial providers, research organizations, and government agencies.
- Aerial Photographs: Collect aerial photographs captured by drones or aircraft for specific regions of interest.
- Mapping Platforms: Access publicly available mapping platforms and contribute annotations to open mapping projects.
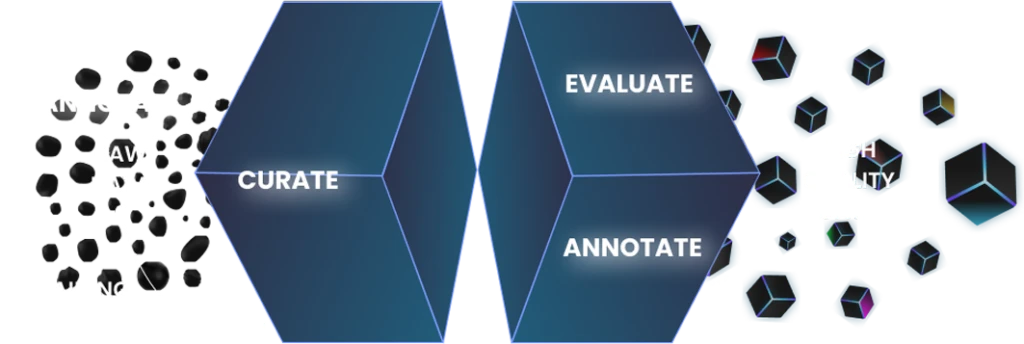
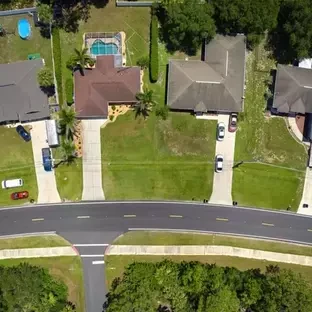
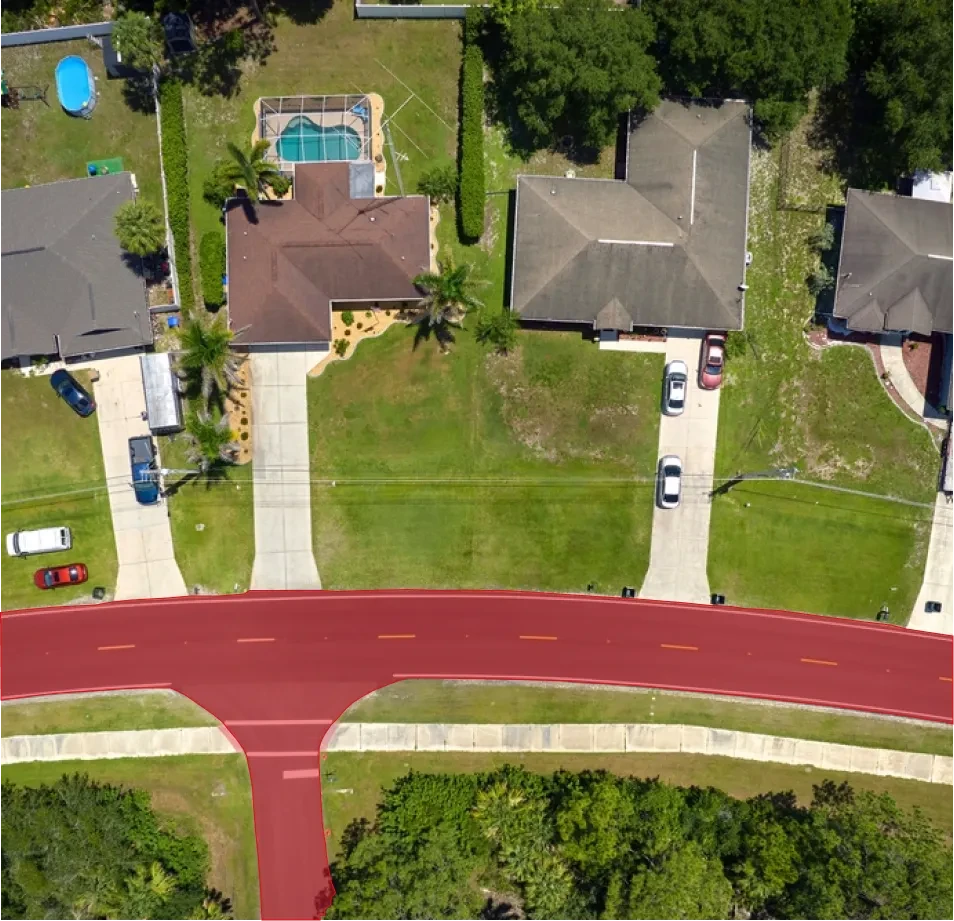
Data Collection Metrics
- Total Geospatial Data Entries: 50,000 entries
- Satellite Imagery: 30,000
- Aerial Photographs: 10,000
- Mapping Platforms: 10,000
Annotation Process
Stages
- Land Cover Annotation: Annotate each geospatial data entry with land cover labels, indicating the types of land cover present in the image, such as forests, urban areas, water bodies, and agriculture.
- Infrastructure Annotation: Label infrastructure features, such as roads, buildings, bridges, and power lines.
- Geographic Features: Annotate geographic features, such as rivers, mountains, and landmarks.
- Metadata Logging: Log metadata, including the source of the data, geographic coordinates, image acquisition date, and any relevant contextual information.
Annotation Metrics
- Geospatial Data Entries with Annotations: 50,000
- Land Cover Annotations: Varies by entry
- Infrastructure Annotations: Varies by entry
- Geographic Features Annotations: Varies by entry
- Metadata Logging: 50,000
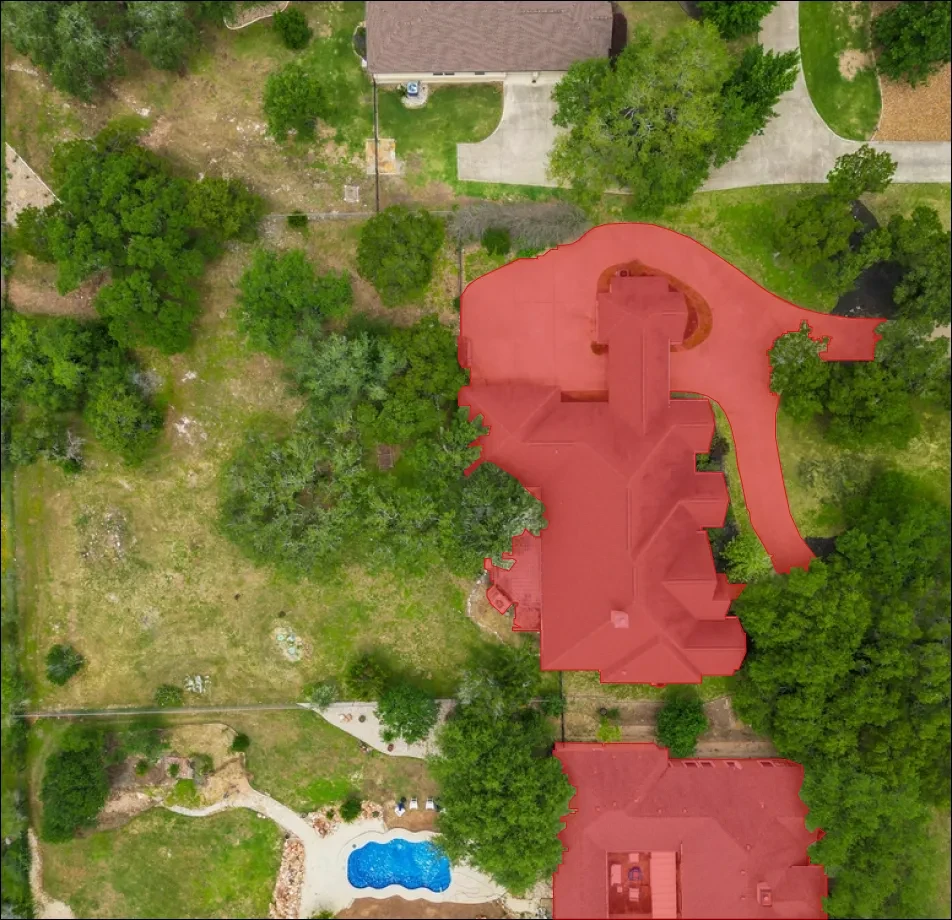
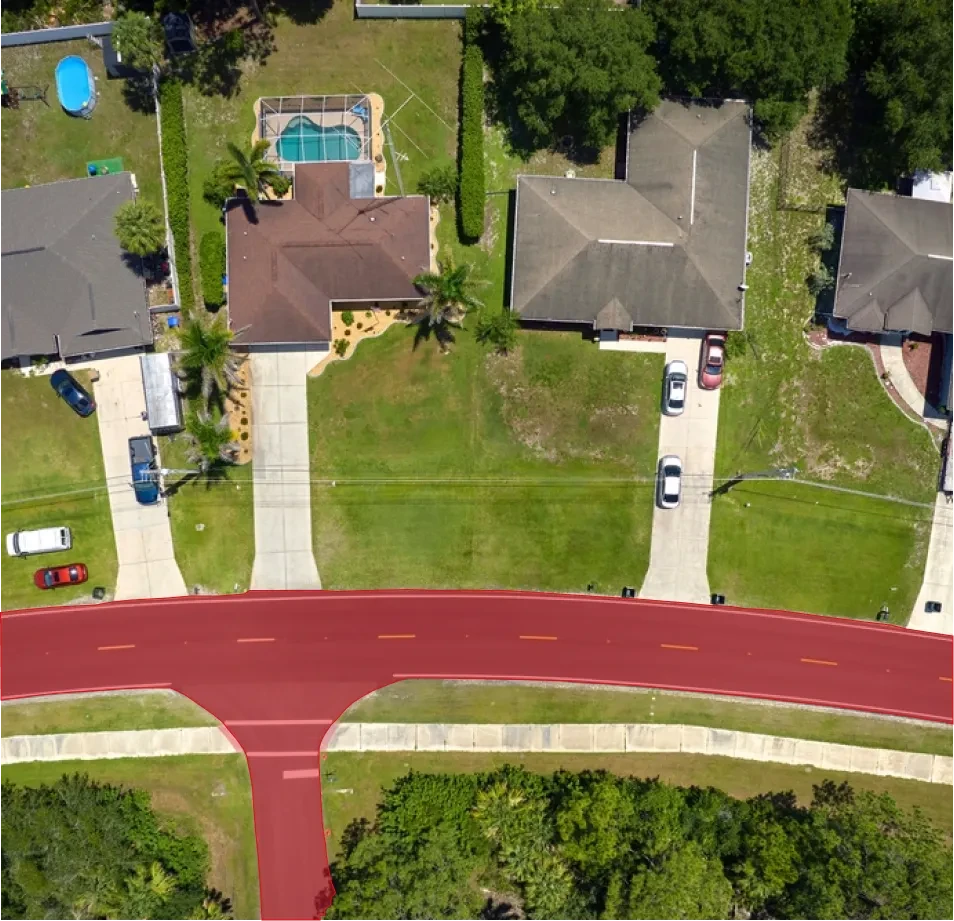
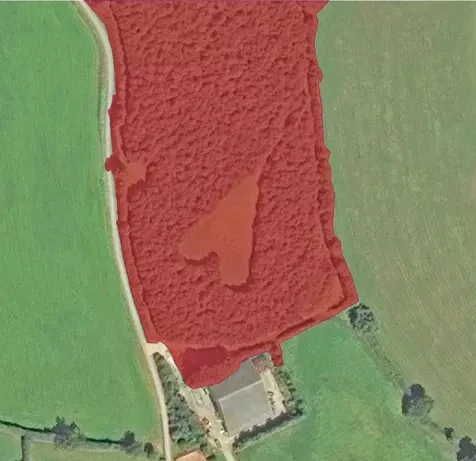
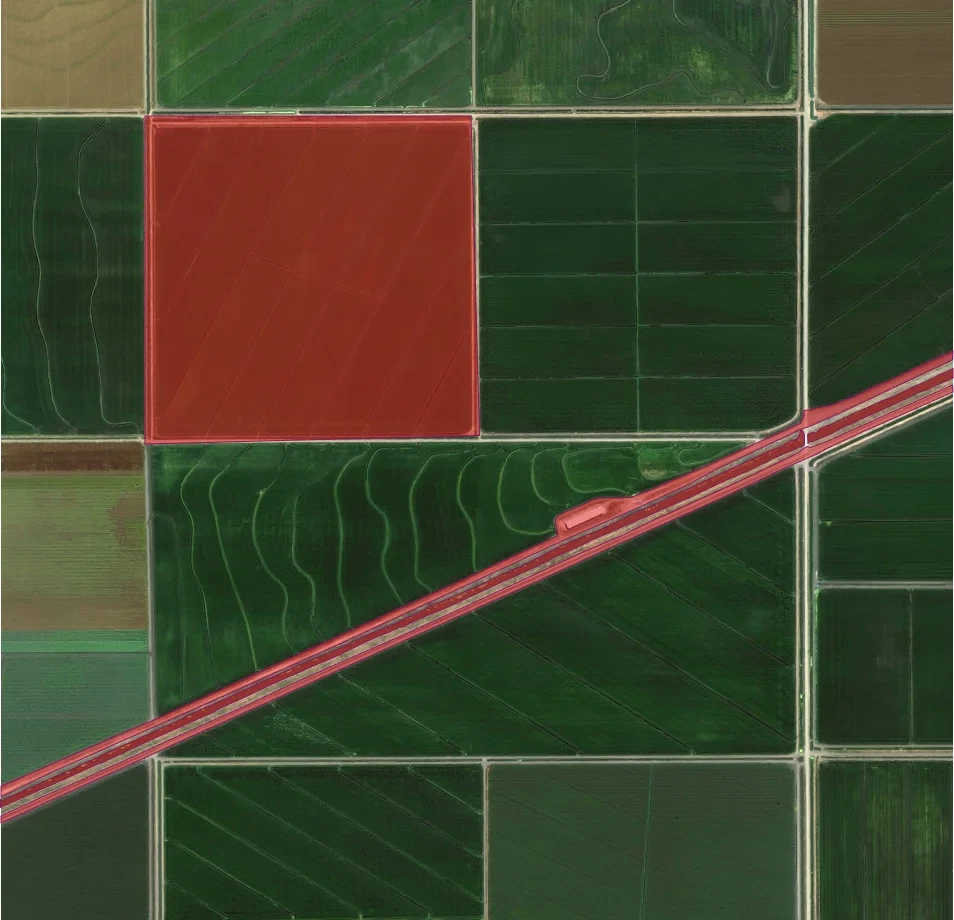
Quality Assurance
Stages
Annotation Verification: Implement a validation process involving geospatial experts to review and verify the accuracy of land cover labels, infrastructure annotations, and geographic features.
Data Quality Control: Ensure the removal of low-quality or noisy data entries and annotations that do not align with the project’s goals.
Data Security: Protect sensitive geographic information and adhere to data privacy regulations
QA Metrics
- Annotation Validation Cases: 5,000 (10% of total)
- Data Cleansing: Remove low-quality or irrelevant data entries and annotations
Conclusion
The “Data Labeling for Geospatial Mapping” dataset is a crucial resource for geospatial mapping, environmental monitoring, and land use analysis. With accurately annotated geospatial data and comprehensive metadata, this dataset empowers the development of advanced machine learning models and tools that can assist in automated land cover classification, infrastructure mapping, and geographic feature recognition. It contributes to improved mapping accuracy, urban planning, and decision-making in various fields, including agriculture, forestry, and environmental conservation.

Quality Data Creation

Guaranteed TAT

ISO 9001:2015, ISO/IEC 27001:2013 Certified

HIPAA Compliance

GDPR Compliance

Compliance and Security
Let's Discuss your Data collection Requirement With Us
To get a detailed estimation of requirements please reach us.