Data Labeling for Autonomous Drone Navigation
Home » Case Study » Data Labeling for Autonomous Drone Navigation
Project Overview:
Objective
Data Labeling: Our goal was to furnish a comprehensive dataset, meticulously annotated to empower drones with the ability to navigate autonomously and efficiently. This project plays a critical role in sectors like agriculture, logistics, and surveillance, boosting operational effectiveness through the use of advanced drone technology.
Scope
Annotating data for aerial imagery, obstacle detection, and route planning is crucial. This labeled data is essential for enabling drones to operate autonomously in various applications.
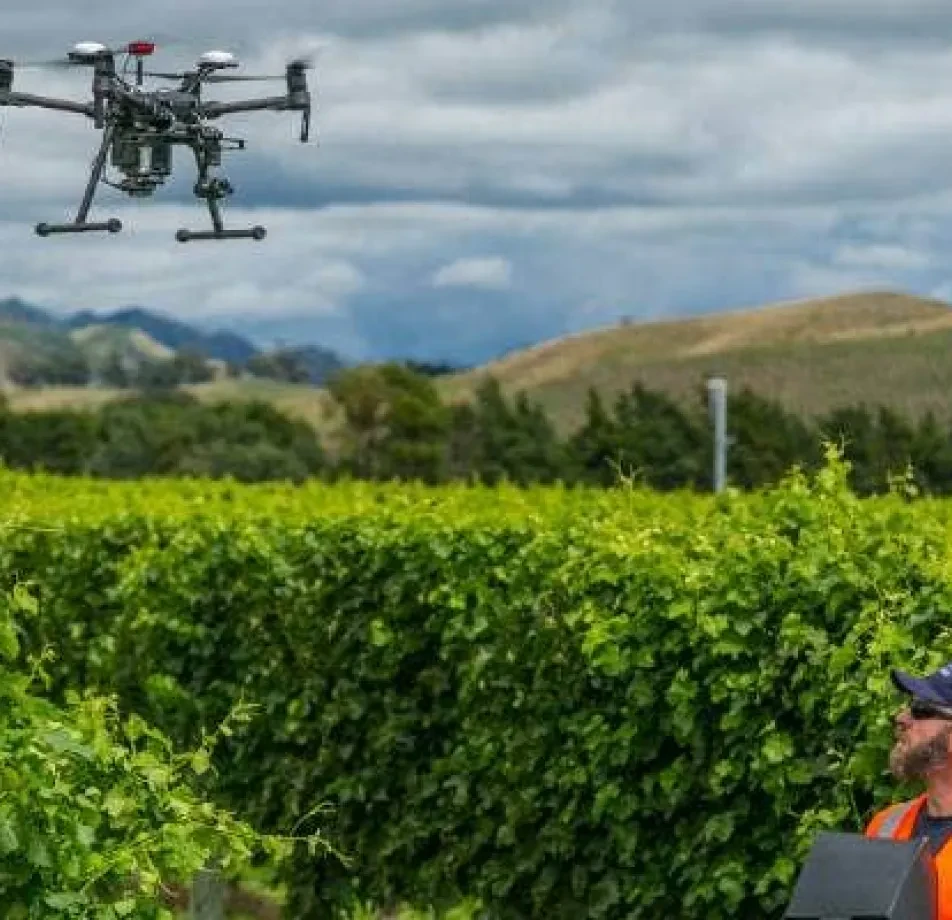
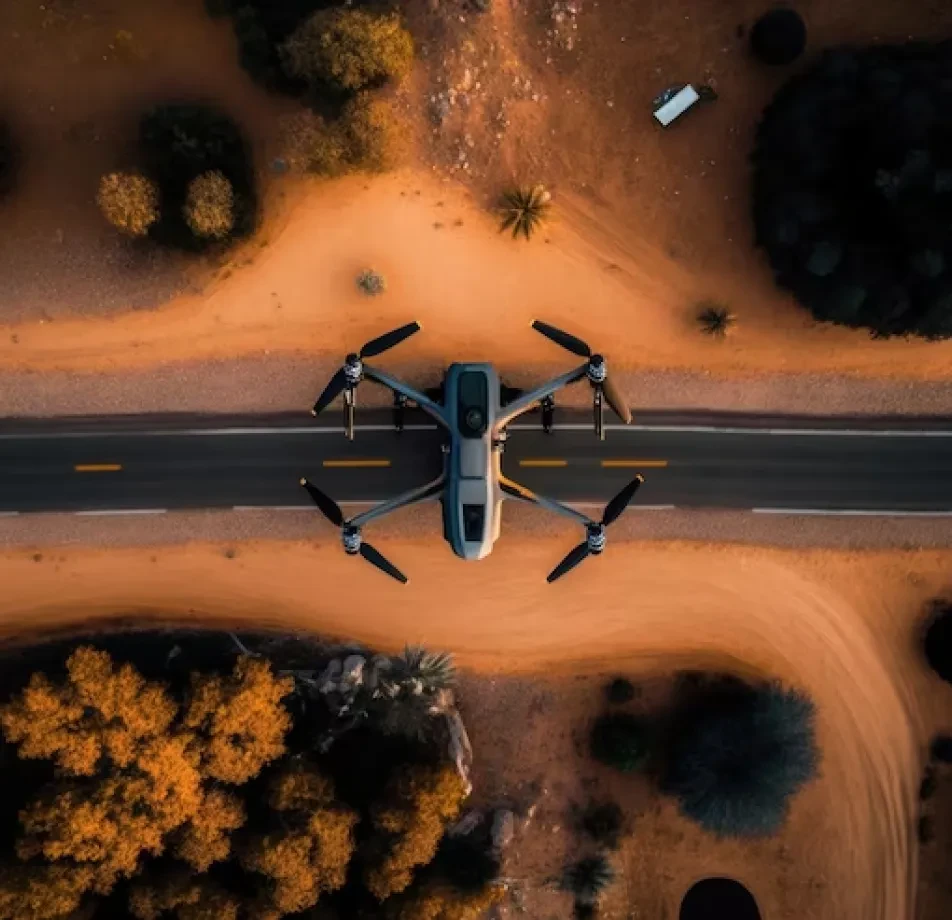
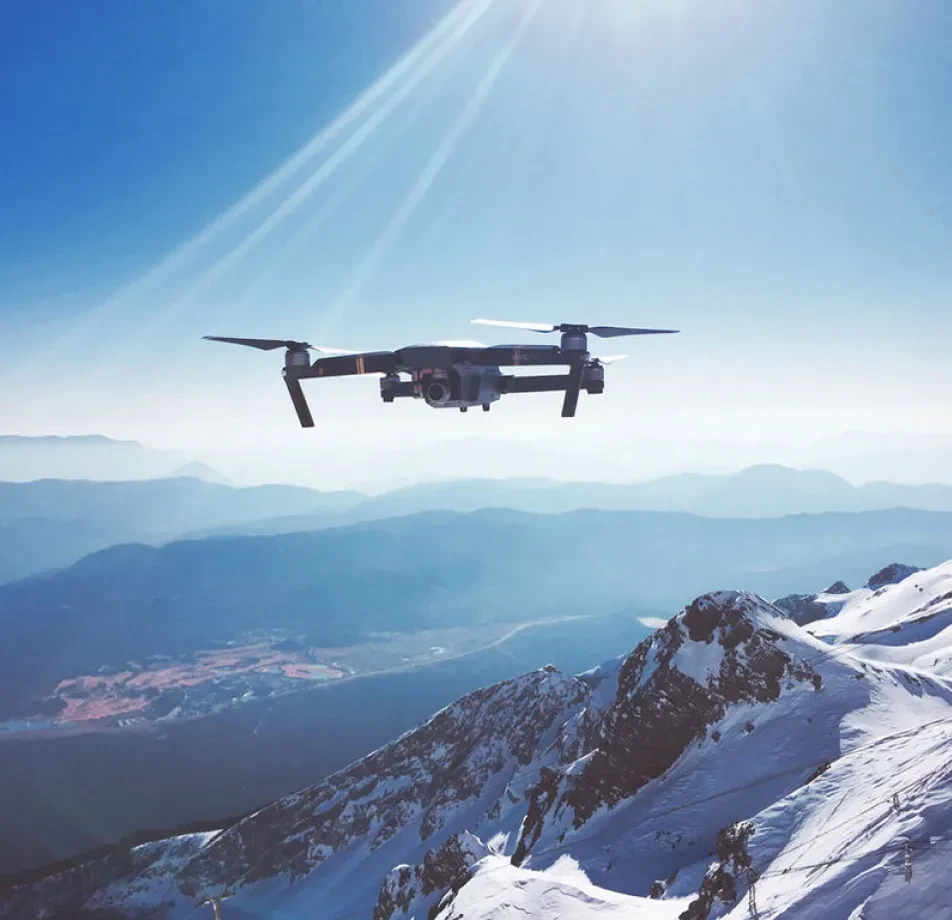
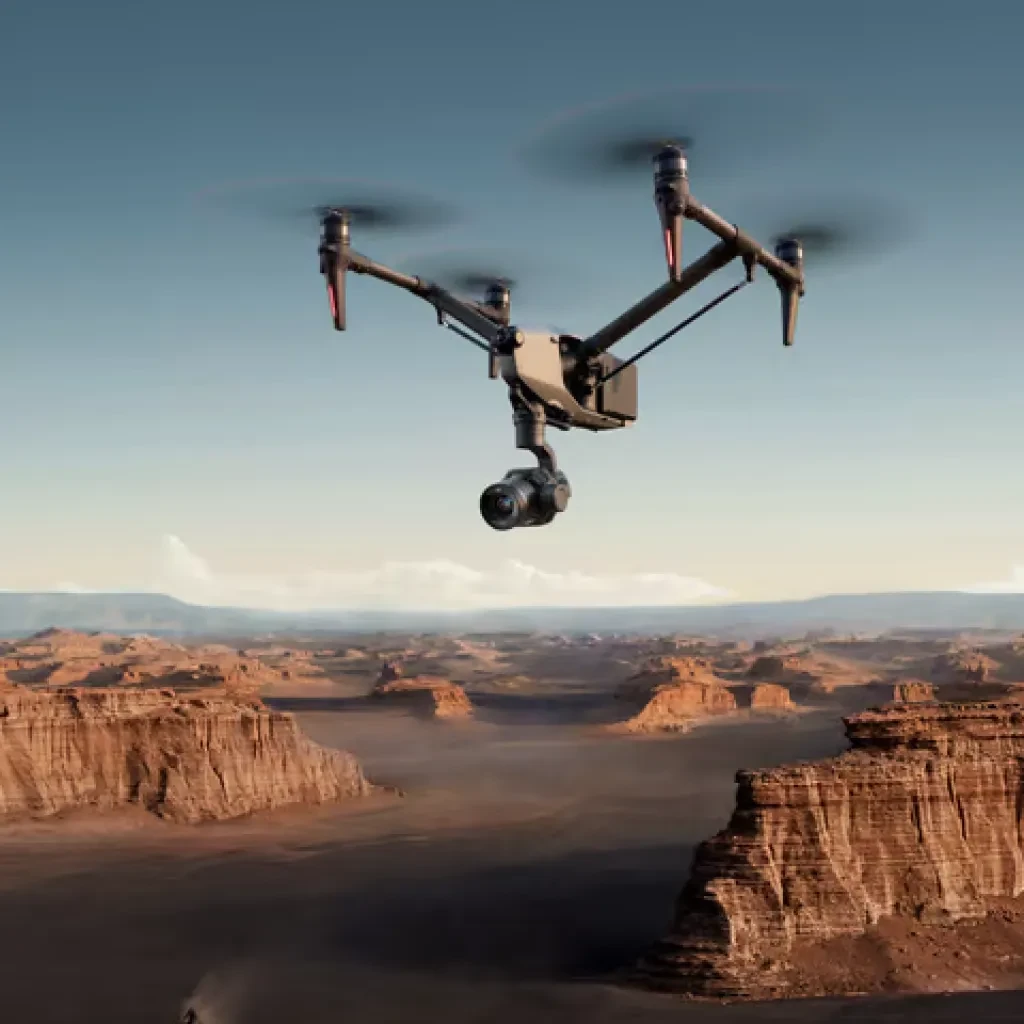
Sources
- By utilizing our state-of-the-art drones and satellite technology, we were able to capture high-resolution imagery, which forms the core of our visual database.
- Human Annotations: Additionally, our team of experienced annotators played a crucial role in identifying, labeling, and verifying objects and navigation paths in the imagery, thereby guaranteeing the data’s accuracy and usability.
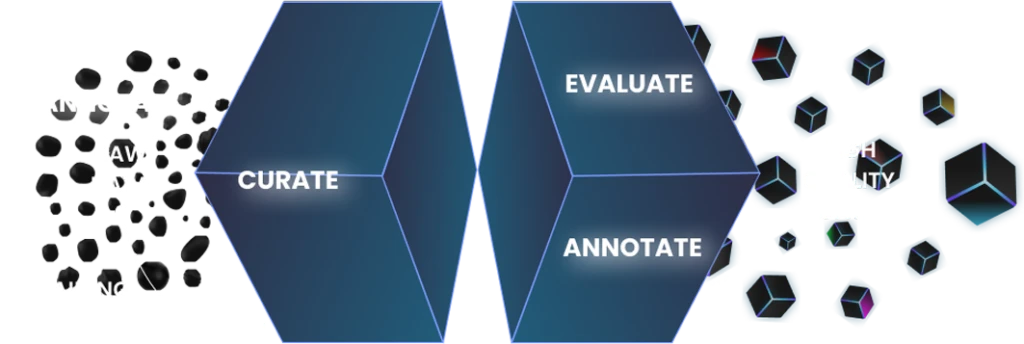
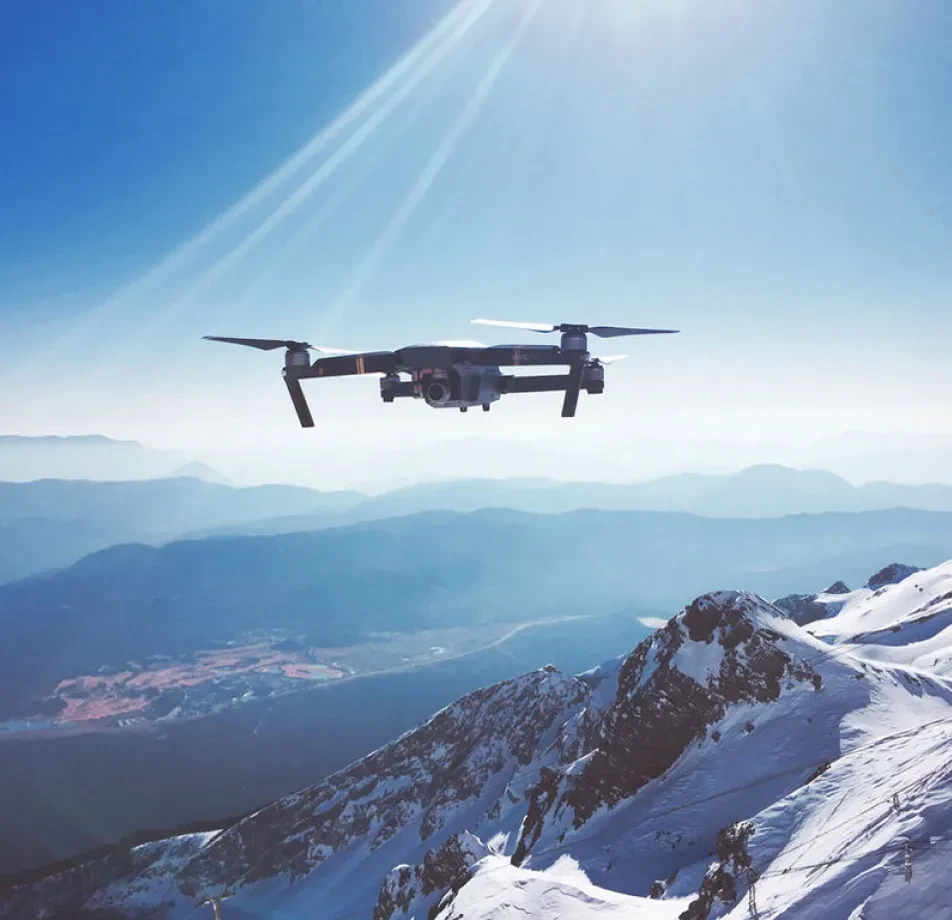
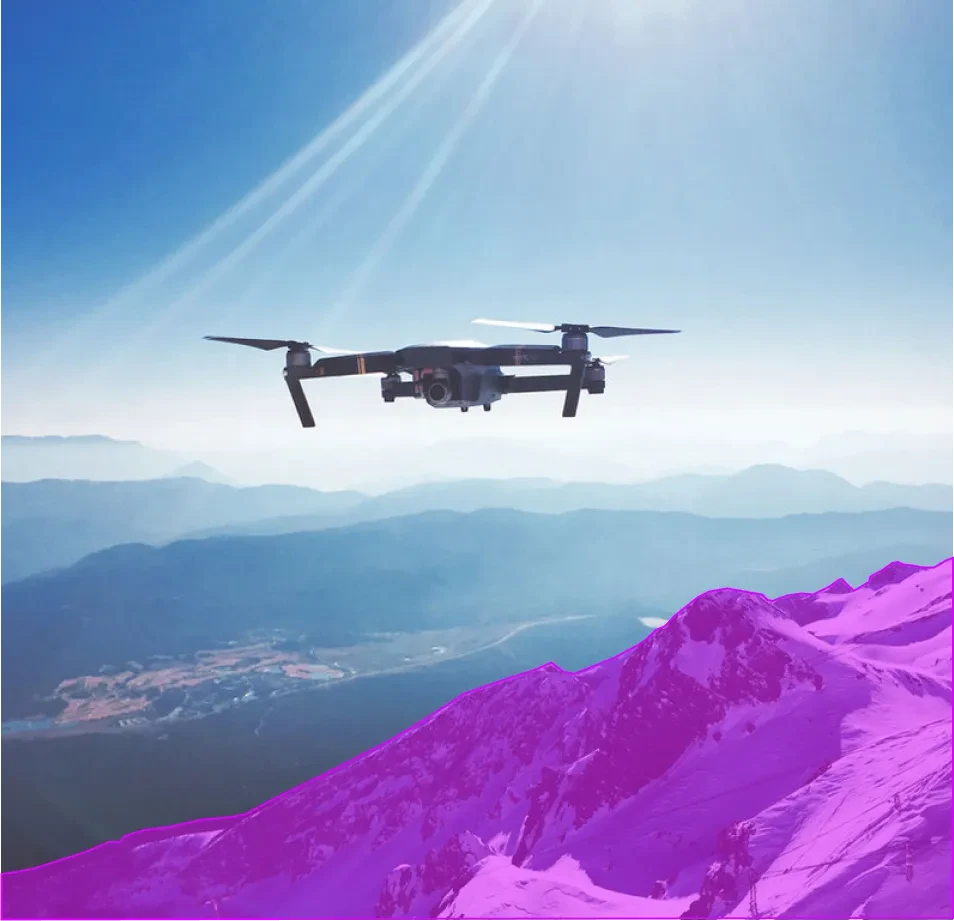
Data Collection Metrics
- Volume: Of 10 million data points, and furthermore, 8 million of these data points were annotated.
- Completeness: To ensure completeness, we focused on covering all essential data aspects for comprehensive navigation.
- Accuracy: Each data point was validated for correctness, thereby maintaining high accuracy standards.
- Timeliness: Additionally, our efficient data collection process catered to real-time application needs, ensuring timeliness.Â
Annotation Process
Stages
- Planning: Setting clear objectives and choosing appropriate data sources and collection methods is essential.
- Data Gathering: Subsequently, efficiently acquiring data from the selected sources is the next step.
- Validation: Furthermore, rigorous checks to ensure data accuracy and integrity must be conducted.
- Analysis: Processing and categorizing data for actionable insights.
- Reporting: After validation, processing and categorizing data for actionable insights follows. Reporting: Finally, presenting findings and interpretations from the data analysis completes the process.Â
Annotation Metrics
- Inter-Rater Agreement: Meanwhile, the F1 Score combines precision and recall to assess annotation accuracy.
- F1 Score: Combines precision and recall to assess annotation accuracy.
- Cohen’s Kappa: evaluates agreement between annotators while accounting for chance agreement, which is important for assessing reliability in data annotation.
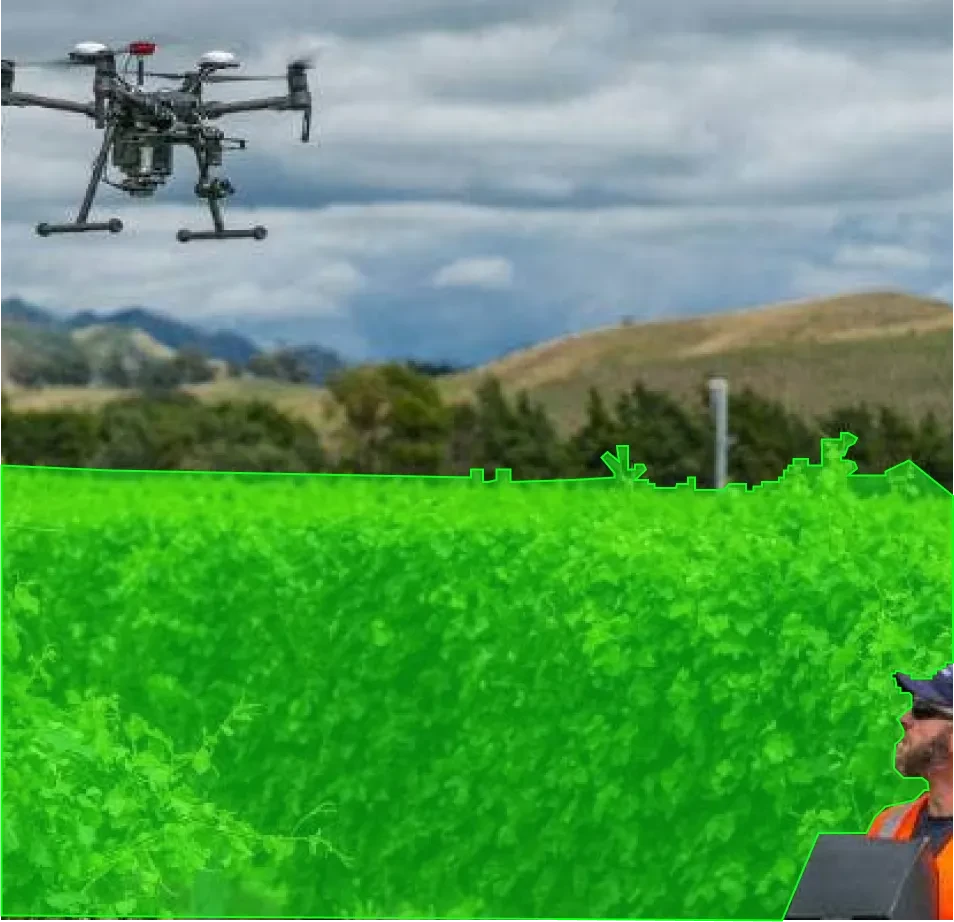
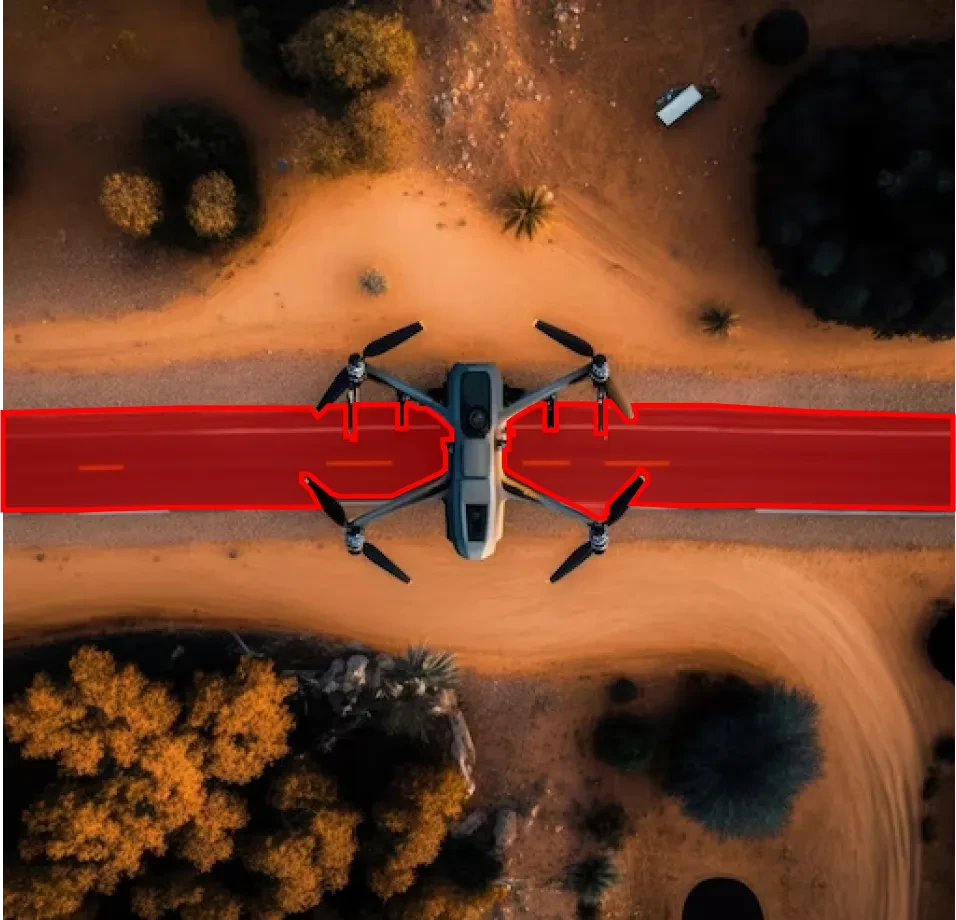
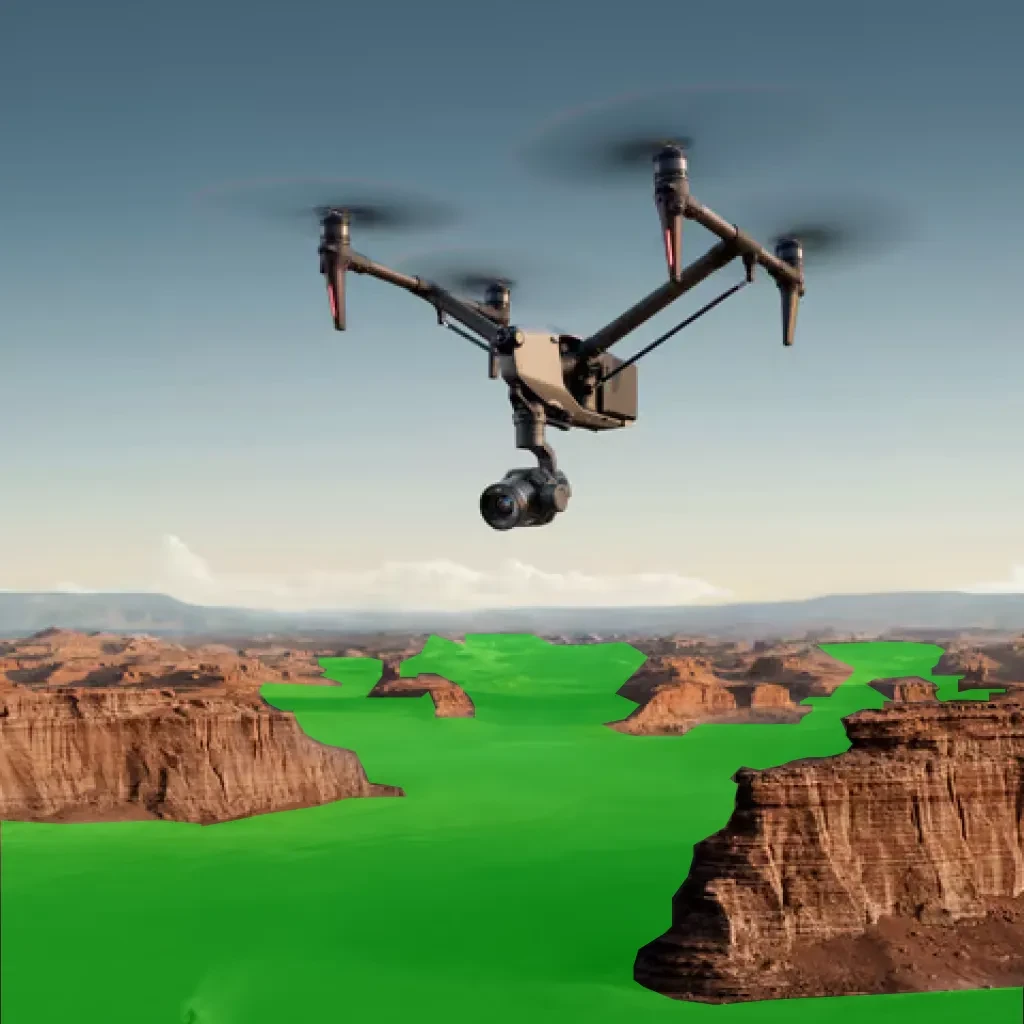
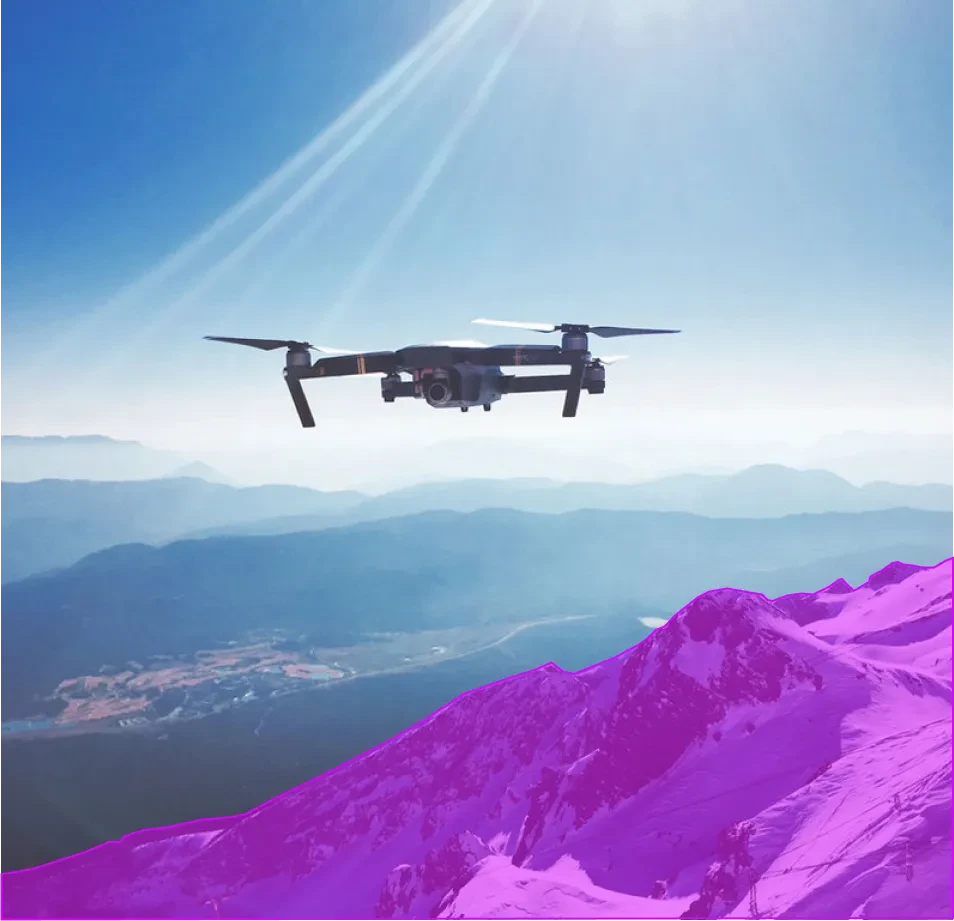
Quality Assurance
Stages
Transcription Verification: Ensuring the accuracy of transcribed content by comparing it to the original source is crucial for data reliability. This process is commonly used in journalism and data entry.
Privacy Compliance: Protecting personal data is essential to build trust and avoid legal problems, especially in a data privacy-regulated environment.
Data Security: Safeguarding data from unauthorized access and breaches through encryption and access controls ensures confidentiality and integrity, which is critical for protection.
QA Metrics
- Defect Rate: This metric measures the number of defects or errors in a product or process, thereby reflecting its quality.
- Customer Satisfaction: This evaluates how well a product or service meets customer expectations, making it a vital quality metric.
Conclusion
Data labeling is a foundational component in the development of autonomous drone navigation systems. Consequently, accurate and detailed labeled data enable drones to perceive and navigate their environment safely and efficiently. Moreover, the use of machine learning and computer vision techniques for data labeling has significantly improved the capabilities of autonomous drones. This advancement, in turn, is paving the way for applications in various industries, such as agriculture, logistics, and surveillance.

Quality Data Creation

Guaranteed TAT

ISO 9001:2015, ISO/IEC 27001:2013 Certified

HIPAA Compliance

GDPR Compliance

Compliance and Security
Let's Discuss your Data collection Requirement With Us
To get a detailed estimation of requirements please reach us.