Damaged Board Parts Segmentation Dataset
Home » Case Study » Damaged Board Parts Segmentation Dataset
Project Overview:
Objective
To develop a comprehensive dataset for identifying damaged components on different types of boards, including circuit boards and wooden boards, our goal is to enhance automation in board inspection systems, damage assessment, and repair recommendations.
Scope
To gather images that display different types of boards under varied lighting conditions and from multiple angles, follow these guidelines. Ensure each image includes segmentation masks for both intact and damaged areas.
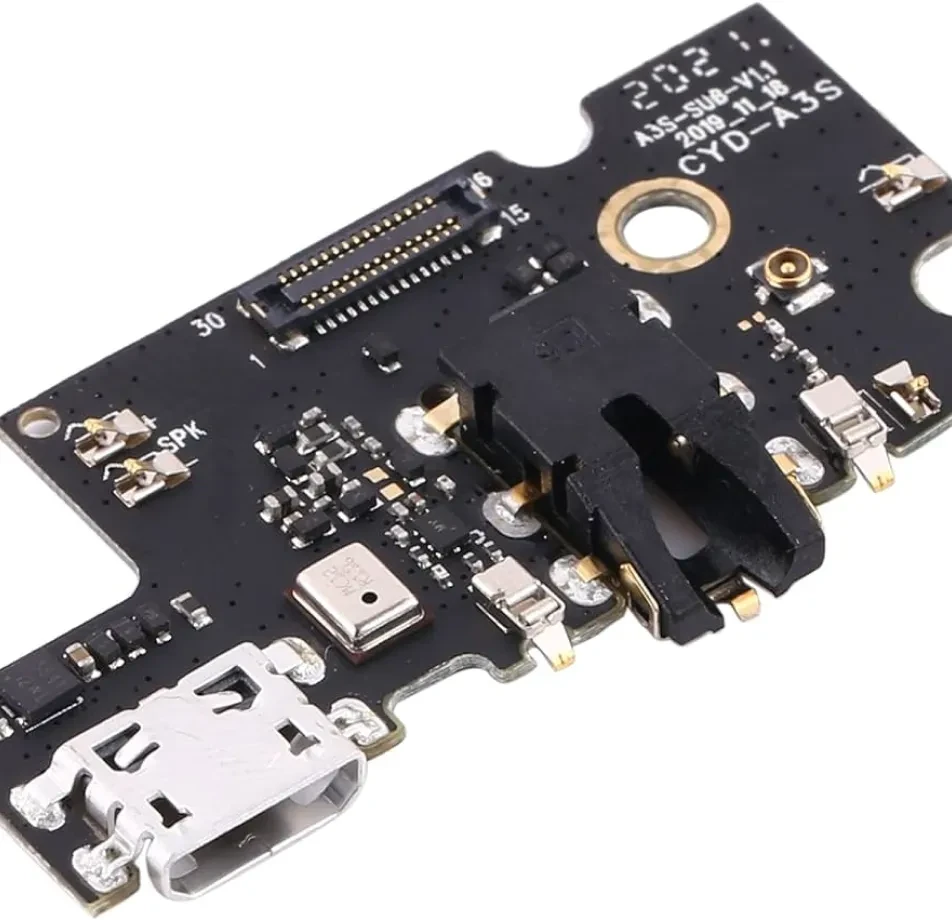
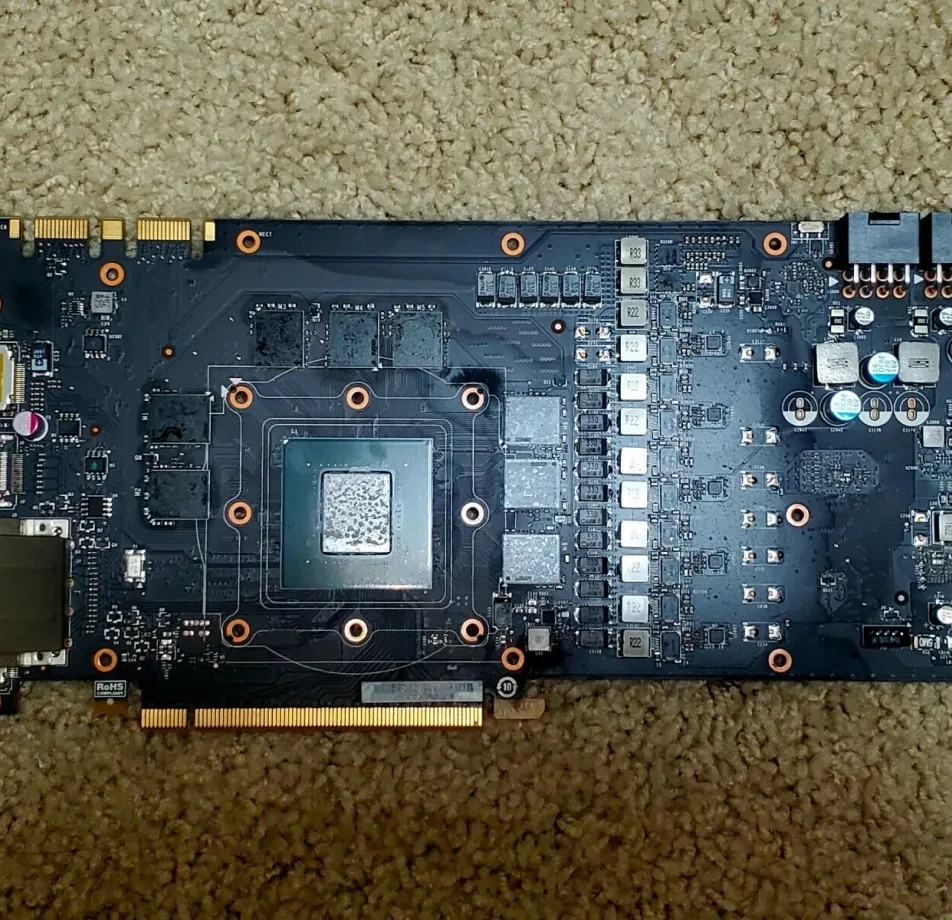
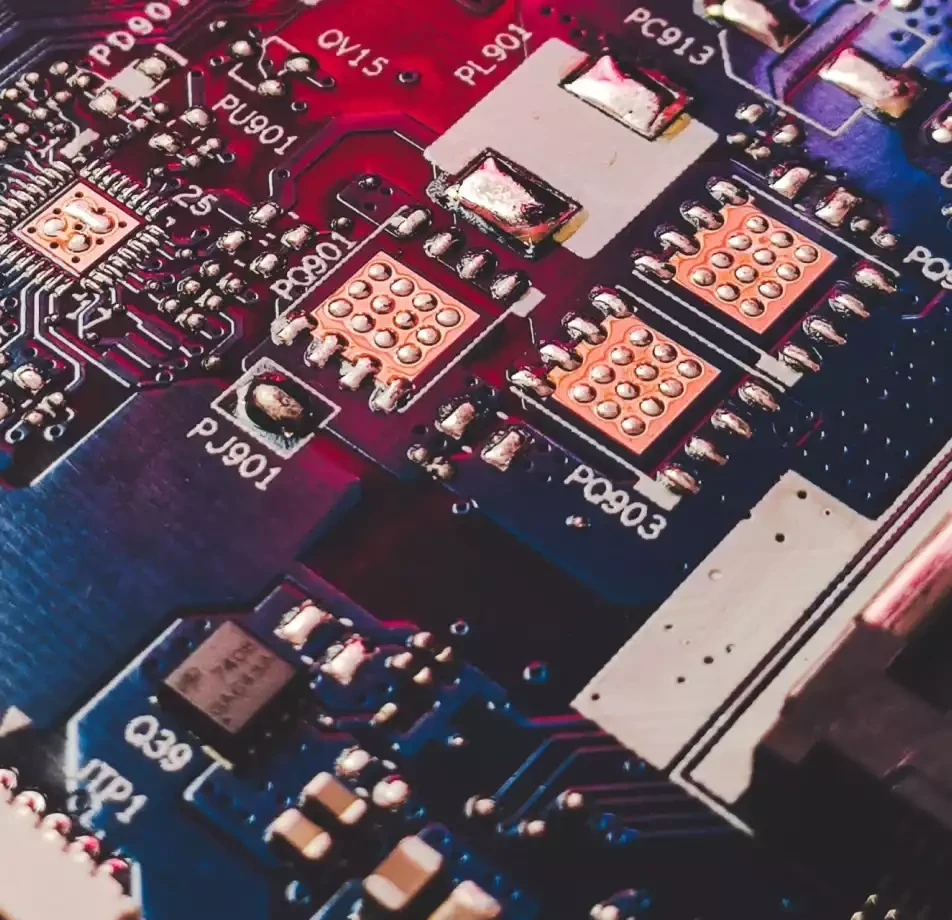
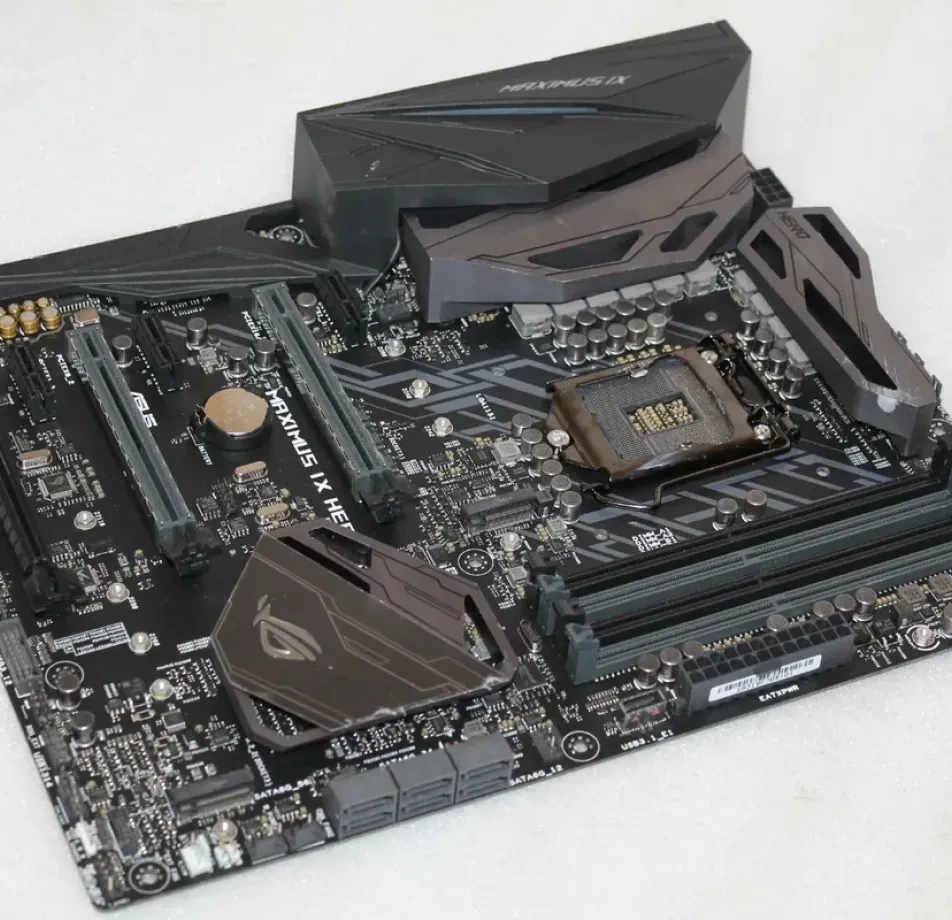
Sources
We engaged in collaborative initiatives with electronics manufacturing units to gain access to images of defective boards. Consequently, we carefully collected and successfully curated an assortment of visuals. This collaboration allowed us to ensure the quality and relevance of the collected images.
We have formed partnerships with carpentry workshops and lumber mills to gather samples of damaged wooden boards. This has resulted in a carefully curated dataset.
Crowdsourced submissions from DIY enthusiasts and hobbyists have been meticulously collected and successfully curated for a diverse compilation.
- Conducted controlled photography sessions for capturing specific types of damage and wear, resulting in a successfully collected and professionally curated set of images.
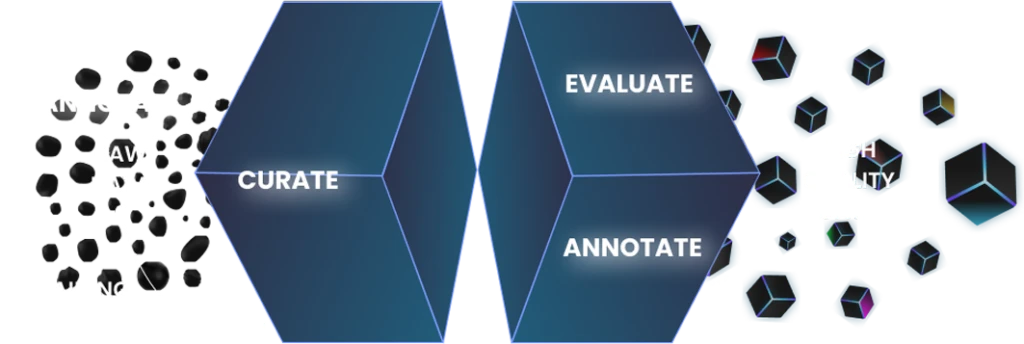
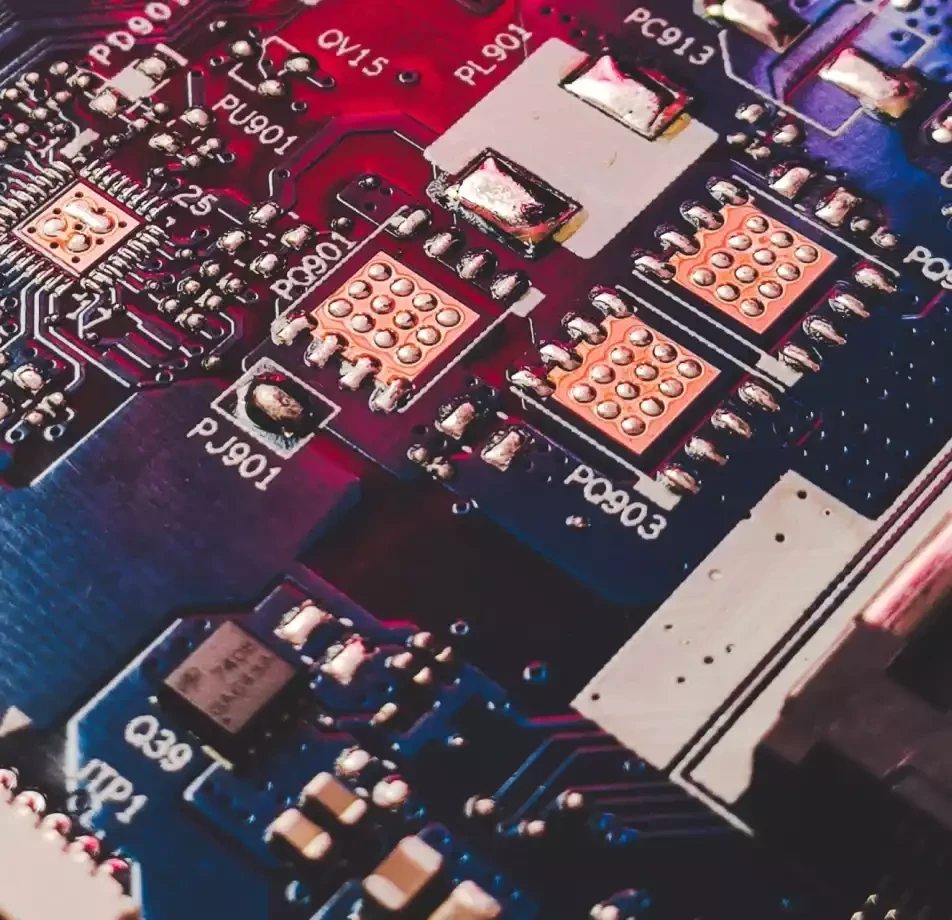
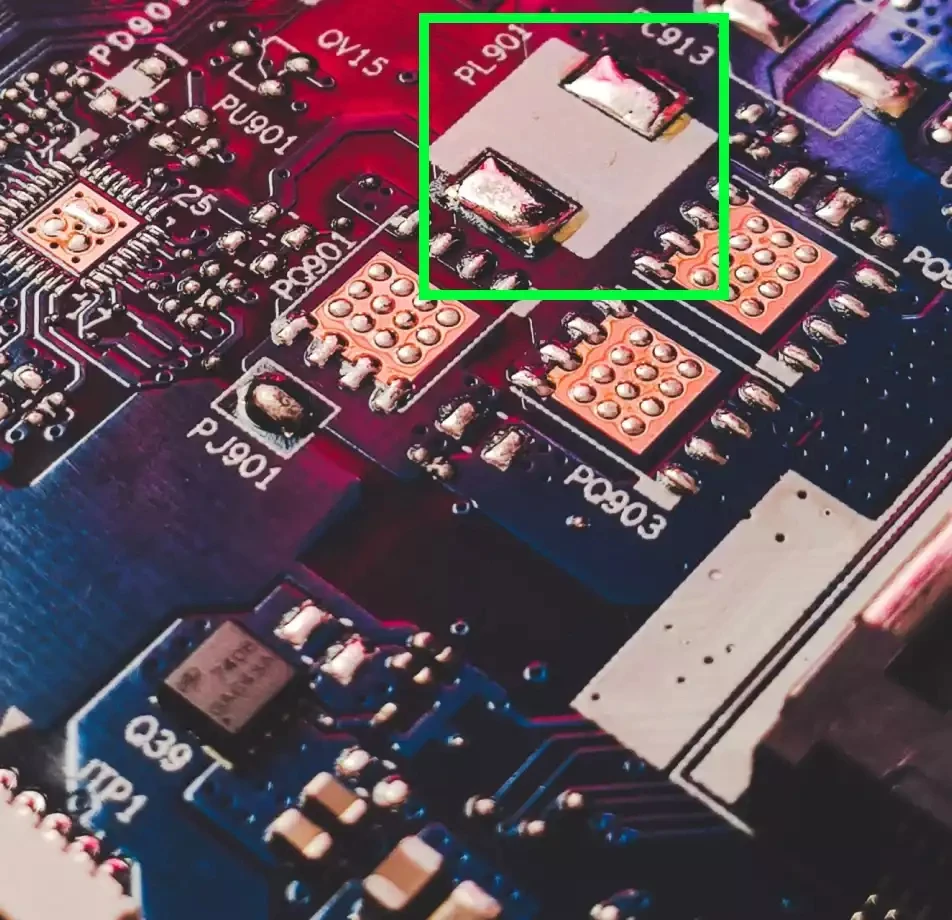
Data Collection Metrics
- Total Images: 50,000
- Electronics Circuit Boards: 25,000
- Wooden Boards: 20,000
- Other Board Materials (Metal, Plastic, etc.): 5,000
Annotation Process
Stages
- Image Pre-processing: First, we equalize the images for resolution and adjust any color imbalances. This ensures consistent quality across all images. Furthermore, we enhance image clarity and uniformity, which is essential for the next steps.
- Segmentation Annotation: Next, skilled annotators segment each component, clearly distinguishing between damaged and undamaged areas. This step is crucial for accurate analysis. Additionally, precise segmentation allows for better data interpretation and modeling.
- Validation: Finally, industry experts review the segmented images for accuracy, ensuring the highest standards are met. They also provide feedback to ensure continuous improvement in the annotation process. As a result, this step guarantees the reliability of the data.
Annotation Metrics
- Total Segmentation Masks: 175,000 (Considering multiple components per image)
- Average Annotation Time per Image: 15 minutes (Considering the intricate nature of board components)
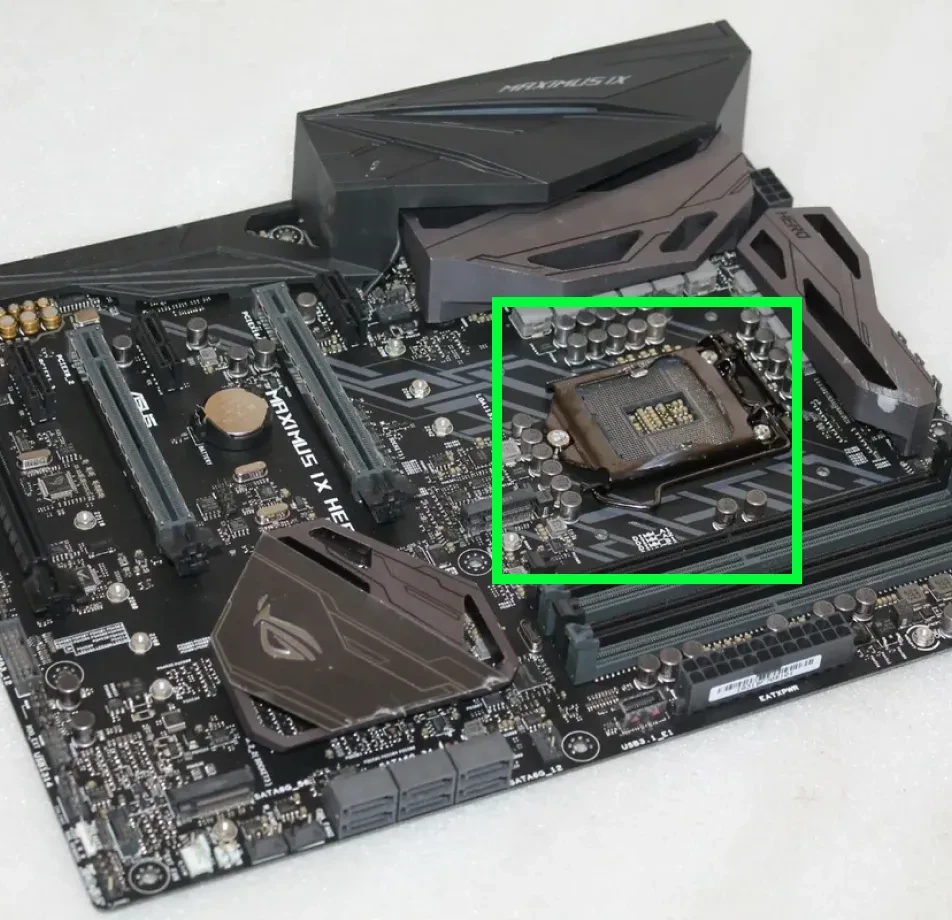
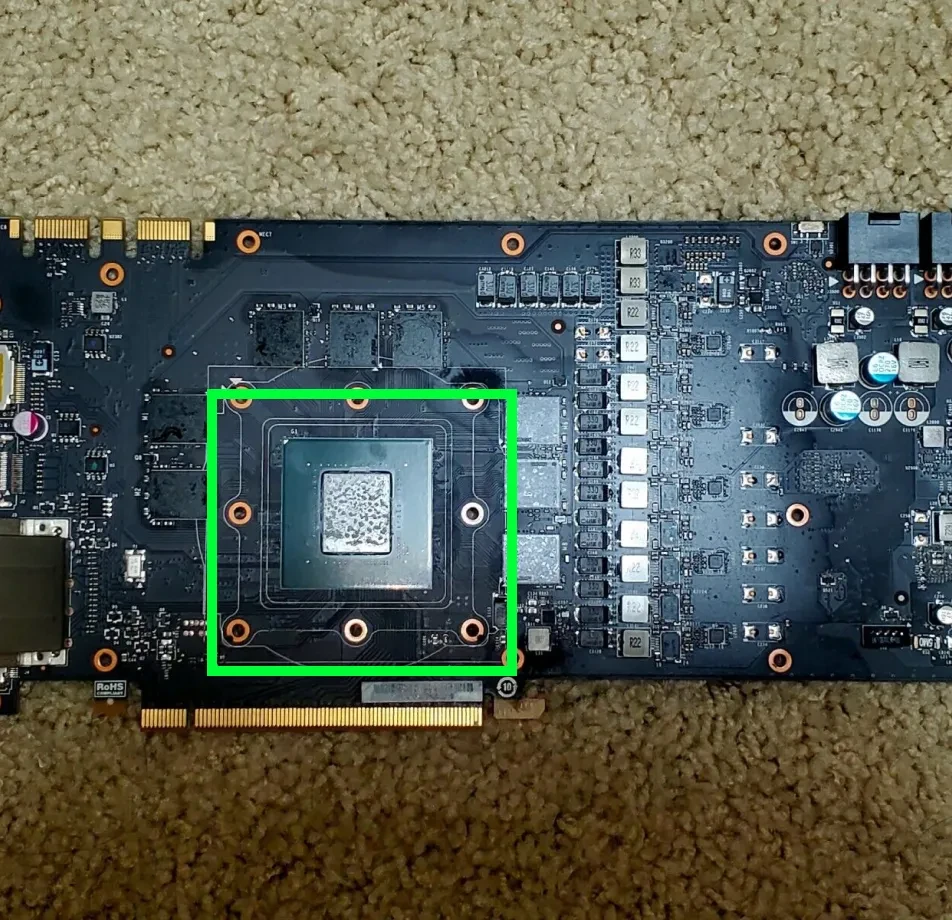
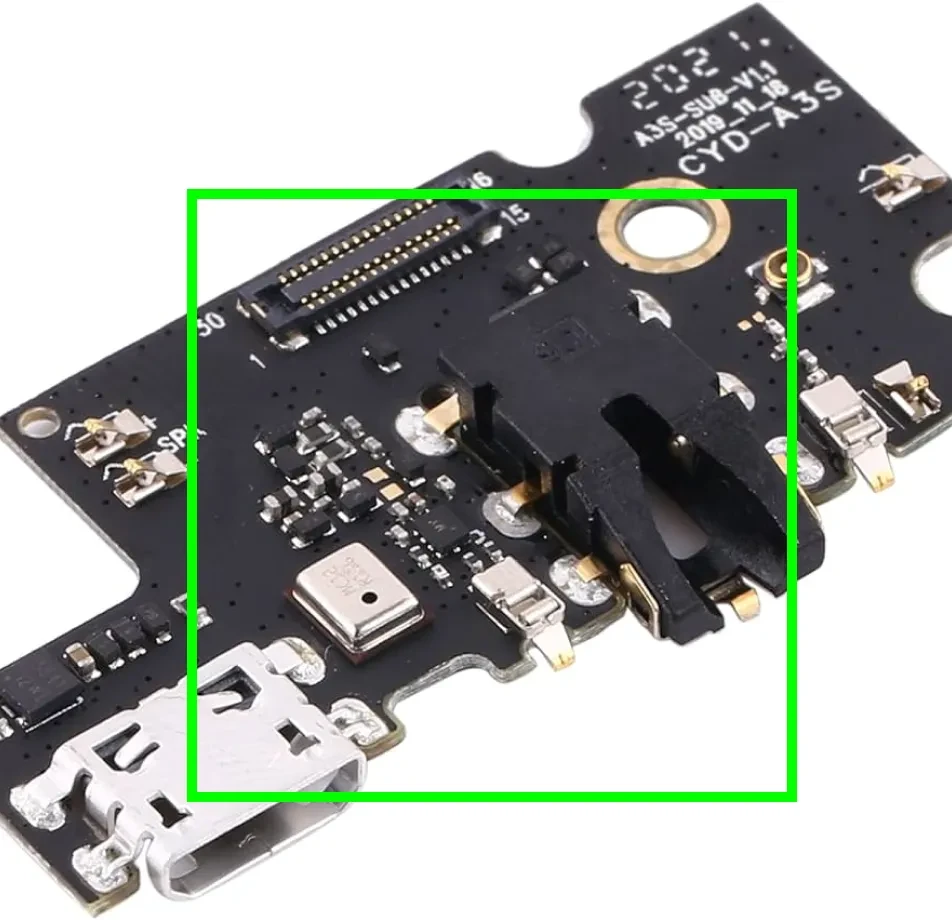
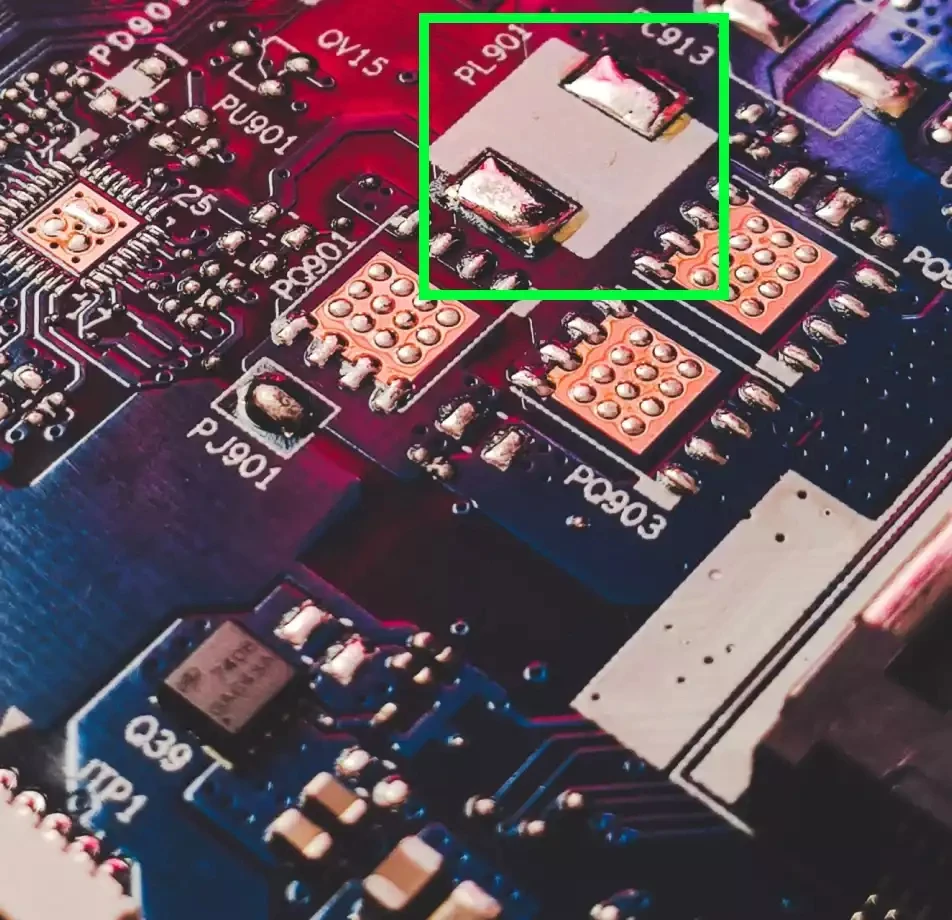
Quality Assurance
Stages
Automated Verification: First, we implement early-stage segmentation models. Then, we compare their outputs with human annotations to identify any possible mismatches.
Dual Review System: Next, each segmented image is reviewed by another expert. This step ensures both consistency and accuracy.
Inter-Annotator Agreement: For ambiguous or complex images, several annotators review them to reach a consensus on damage delineation.
QA Metrics
- Segmentations Verified using Automated Checks: 25,000 (50% of total images)
- Dual-reviewed Segmentations: 15,000 (30% of total images)
- Detected and Corrected Inconsistencies: 750 (1.5% of total images)
Conclusion
The Damaged Board Parts Segmentation Dataset is a valuable resource for industries aiming to improve quality control and board assessment processes. By offering precise segmentation of damaged components, it paves the way for better inspection systems and more accurate damage evaluations. Therefore, industries can rely on this dataset to enhance their quality control measures.

Quality Data Creation

Guaranteed TAT

ISO 9001:2015, ISO/IEC 27001:2013 Certified

HIPAA Compliance

GDPR Compliance

Compliance and Security
Let's Discuss your Data collection Requirement With Us
To get a detailed estimation of requirements please reach us.