Conversational Context Understanding for LLM-based Chatbots
Project Overview:
Objective
The goal was to develop a dataset that would train and evaluate LLMs in understanding and retaining conversational context over long interactions, thereby enhancing the naturalness and engagement of chatbot responses.
Scope
The dataset includes synthetic multi-turn conversations across a range of scenarios, such as customer support, casual chatting, and information retrieval. Each conversation is annotate to highlight key context points and conversational shifts, aiding the LLM in maintaining context.
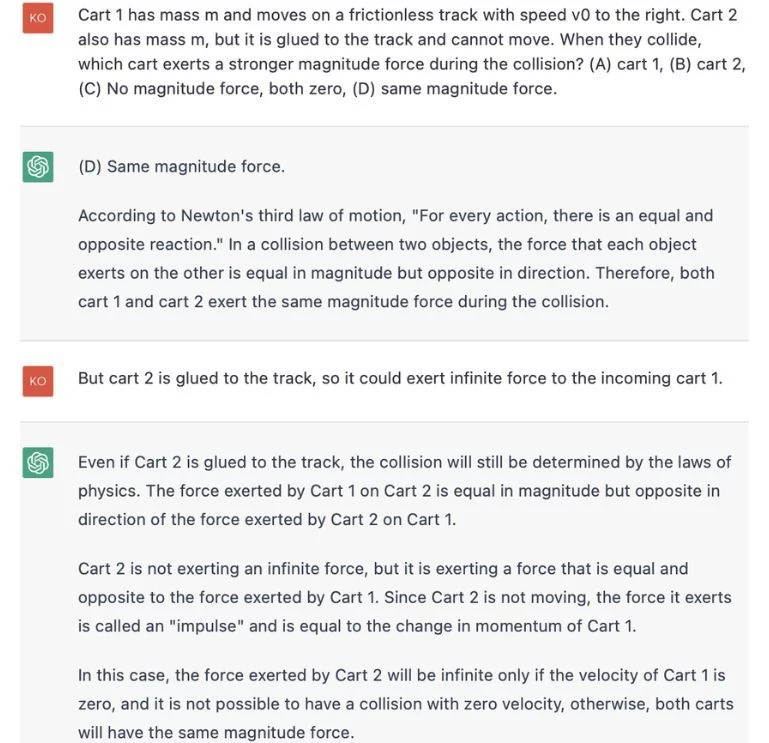
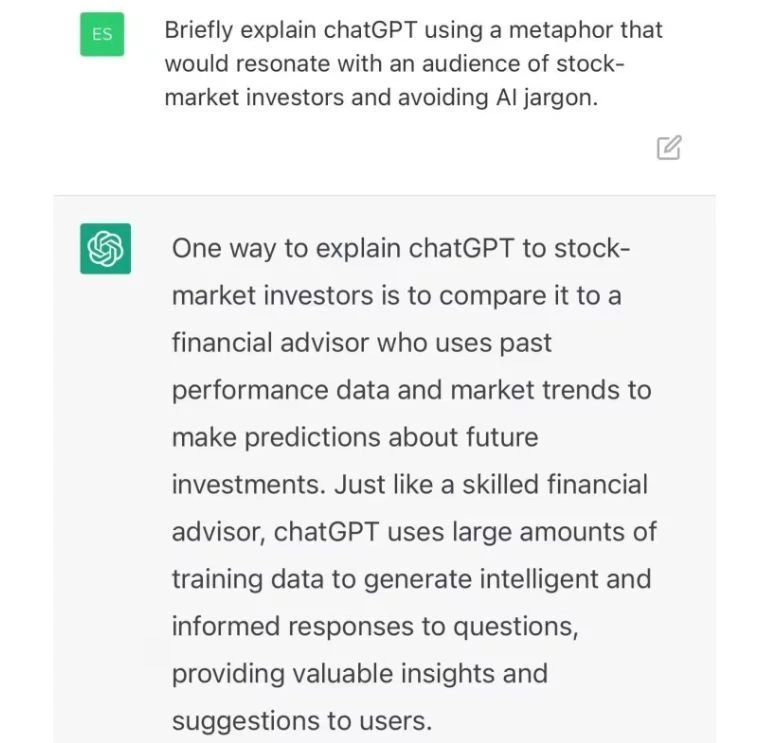
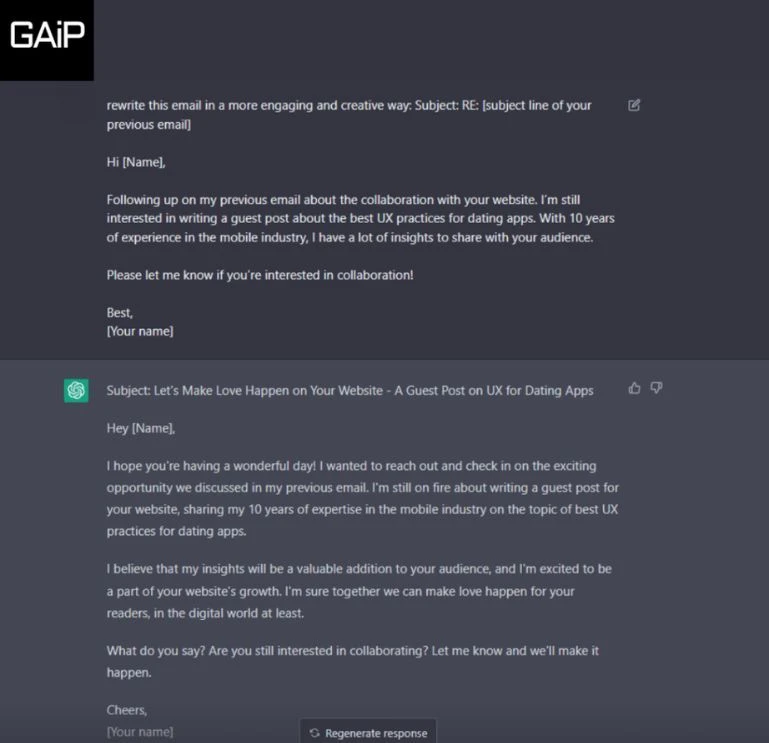
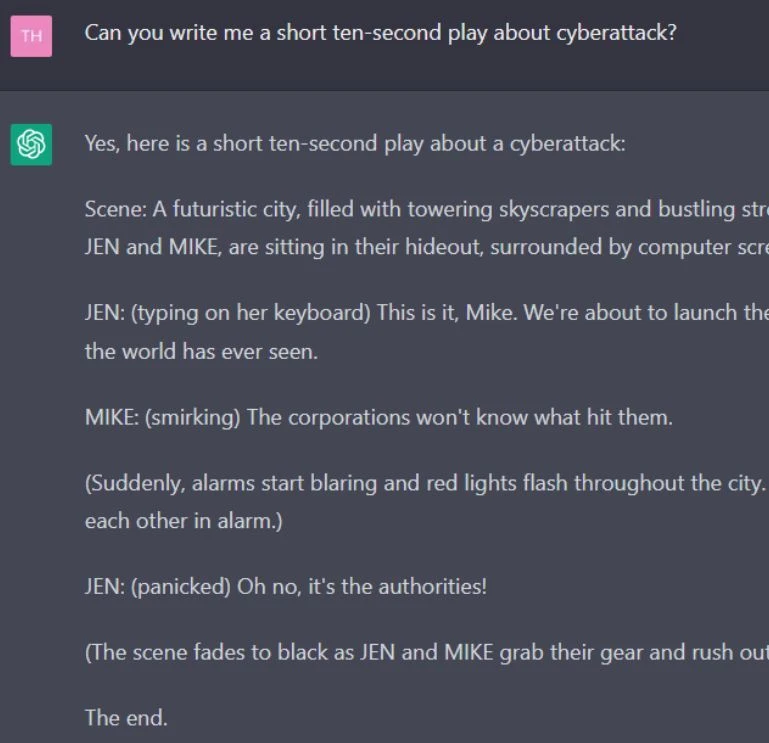
Sources
- Synthetic Data Generation: The data was generated through 50,000 synthetic conversations, crafted by conversational AI experts to simulate real-world interactions.
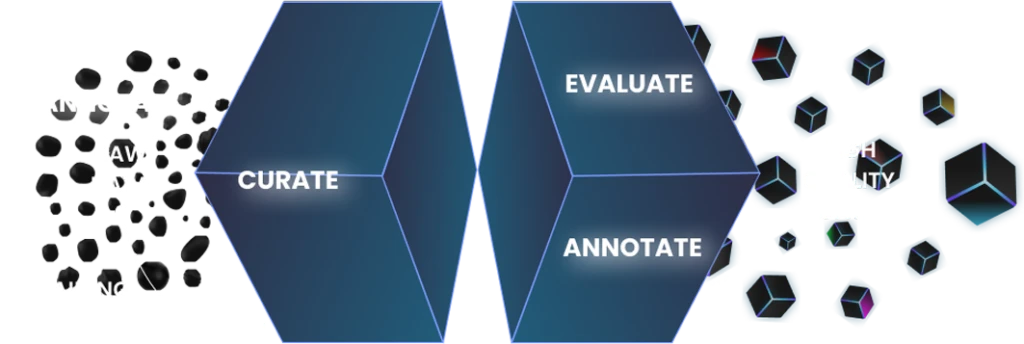
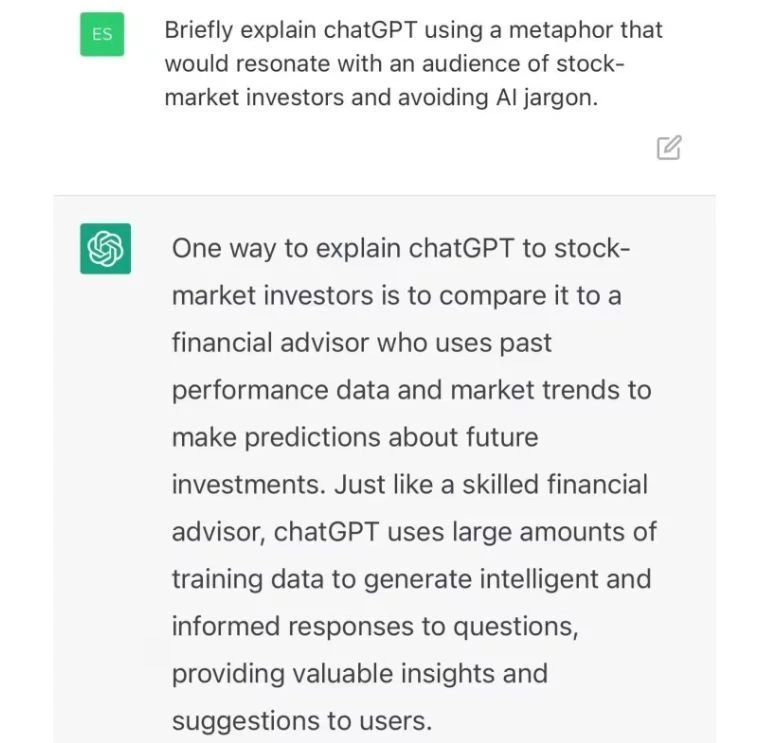
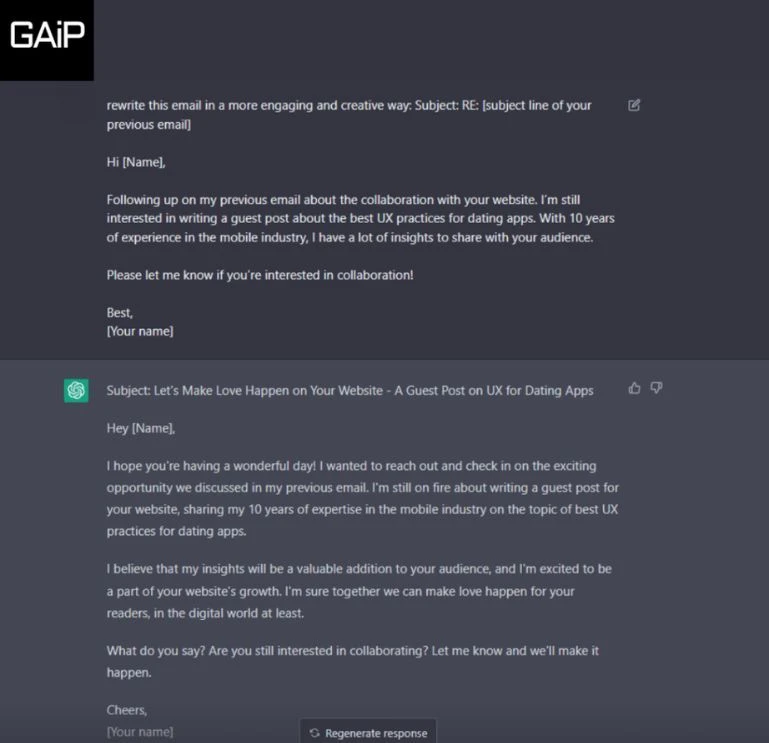
Data Collection Metrics
- Total Conversations Generate: 50,000 synthetic multi-turn conversations.
- Context Points Identify: 150,000 context points were identify and annotate (averaging 3 per conversation).
Annotation Process
Stages
- Contextual Analysis: Annotators with expertise in linguistics and conversational AI analyze each conversation to identify key context points, conversational shifts, and relevance.
- Context Retention: The annotations were design to help the LLM understand and retain context, ensuring more coherent and contextually appropriate responses.
Annotation Metrics
- Team Involvement: A team of 40 annotators, specializing in linguistics and conversational AI, work over a period of 3 months to complete the project.
- Total Annotations: 150,000 annotations were made, focusing on context points and conversational flow.
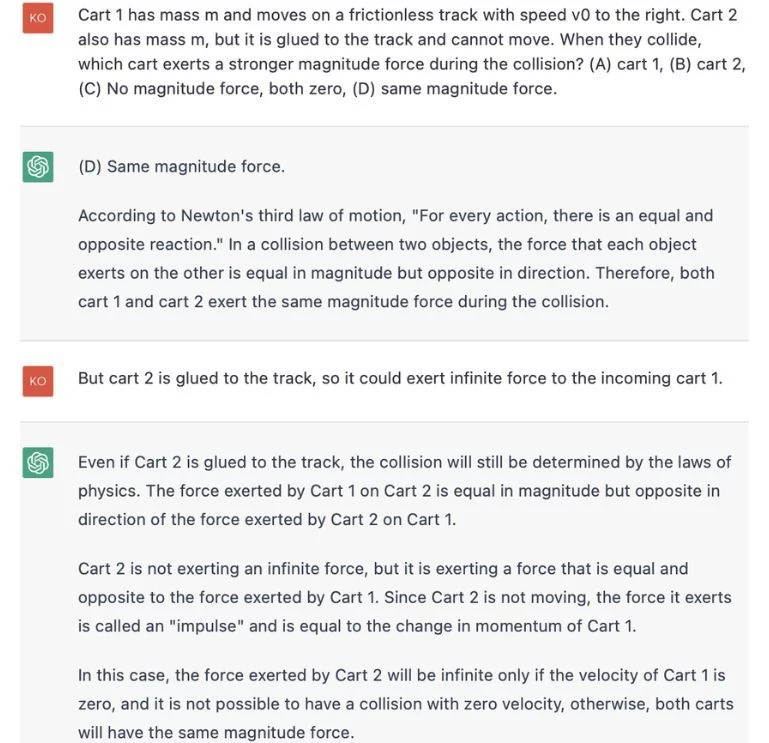
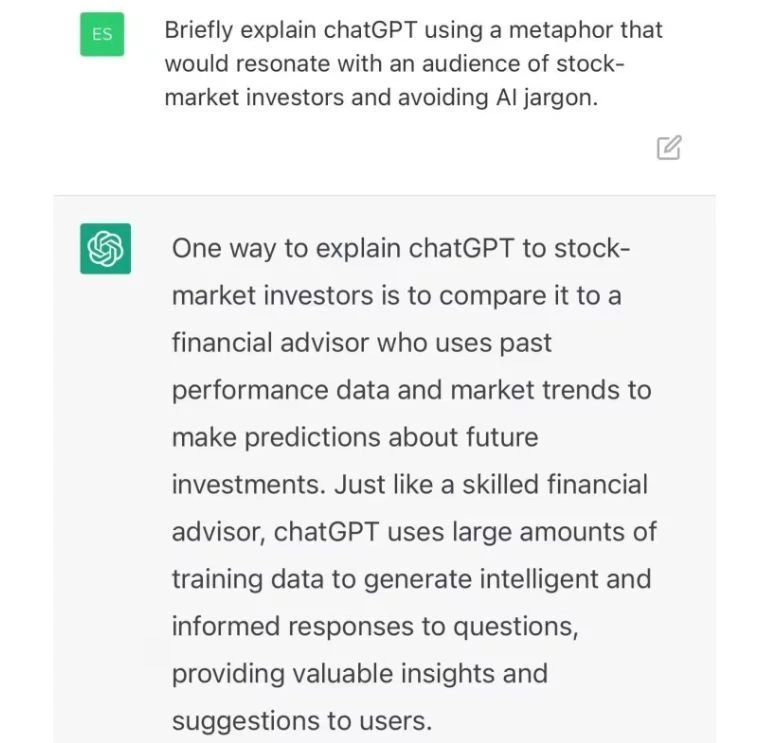
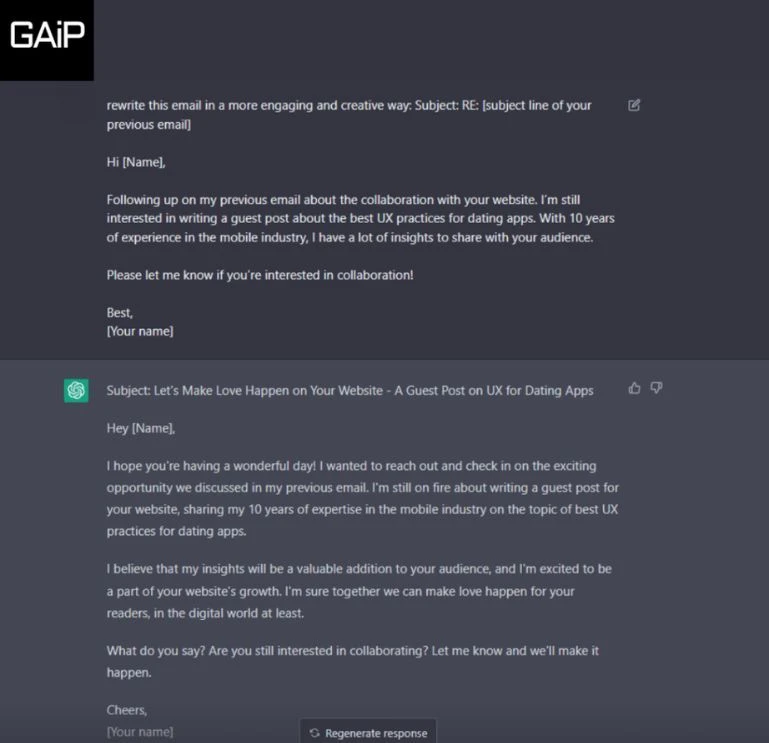
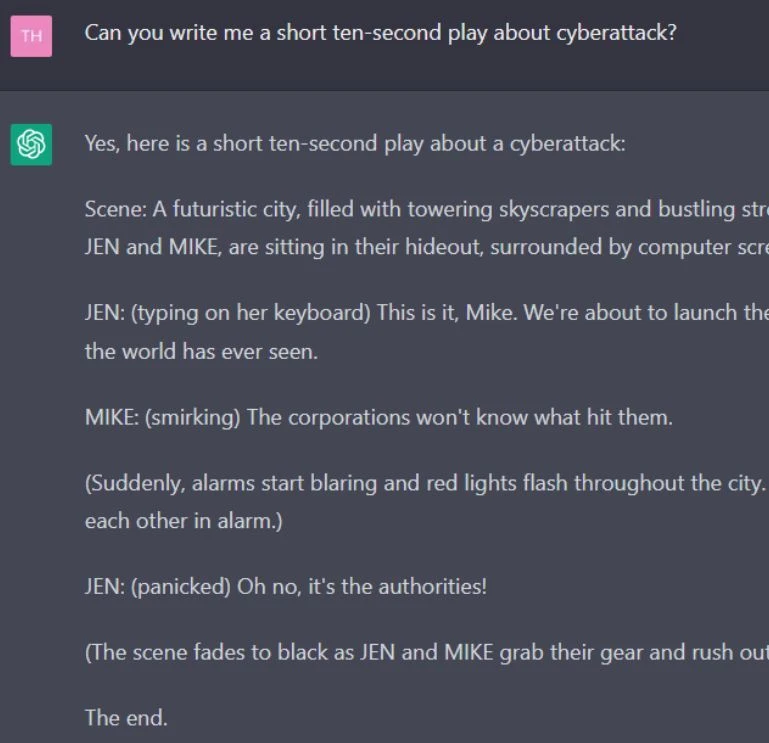
Quality Assurance
Stages
- Annotation Accuracy: Rigorous checks were perform to ensure that context points were accurately identify and annotate, and that conversational shifts were clearly marked.
- Consistency Reviews: Regular reviews were conduct to maintain consistency across all annotated conversations.
QA Metrics
- Context Retention Accuracy: The dataset achieved high accuracy in correctly identifying and maintaining context across extend conversations.
- Conversational Flow Accuracy: Annotations accurately reflect the conversational flow, enhancing the LLM’s ability to provide natural and engaging responses.
Conclusion
The development of this conversational context dataset significantly improve the ability of LLM-based chatbots to handle extend dialogues. As a result, the chatbots now offer more coherent and contextually relevant interactions, enhancing user experience across various applications.

Quality Data Creation

Guaranteed TAT

ISO 9001:2015, ISO/IEC 27001:2013 Certified

HIPAA Compliance

GDPR Compliance

Compliance and Security
Let's Discuss your Data collection Requirement With Us
To get a detailed estimation of requirements please reach us.