CCTV Traffic Scene Sematic Segmentation Dataset
Home » Case Study » CCTV Traffic Scene Sematic Segmentation Dataset
Project Overview:
Objective
Our goal was to develop a comprehensive dataset derived from CCTV traffic scenes, specifically designed for semantic segmentation tasks in machine learning. This dataset is unique in its aim to precisely identify and classify each pixel in an image based on its associated object or area.
Scope
Collection of diverse traffic scenes from CCTV cameras and high-quality pixel-wise annotation to classify every segment of the image.
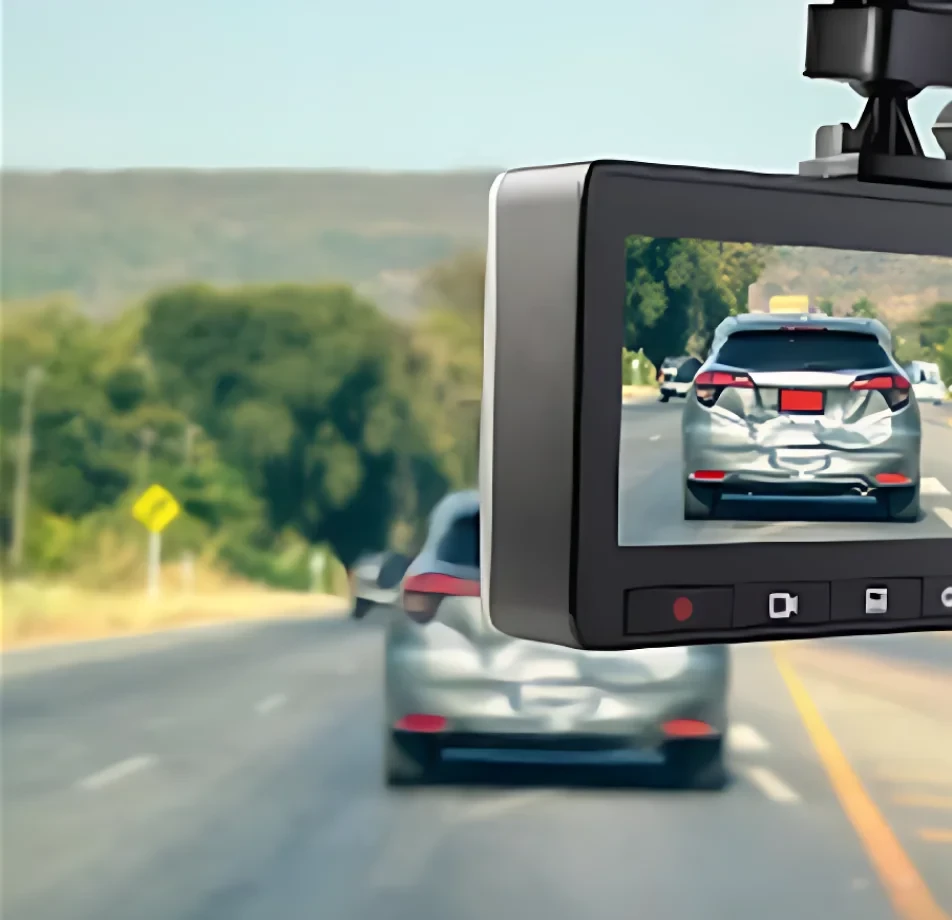
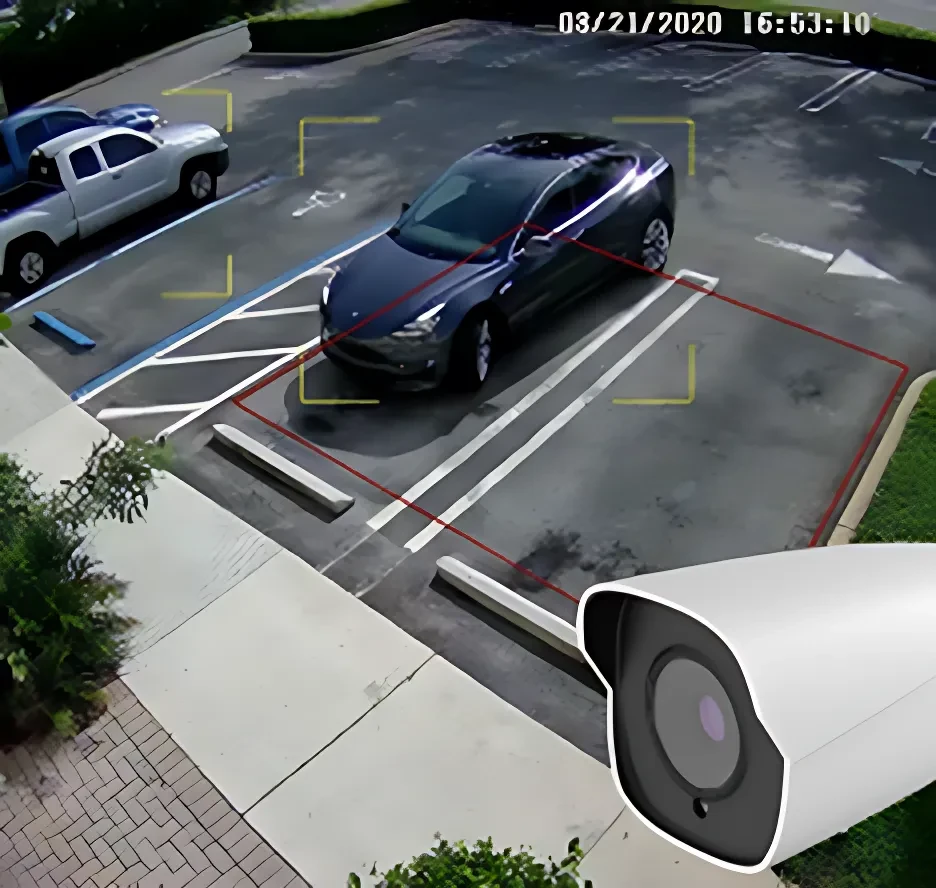
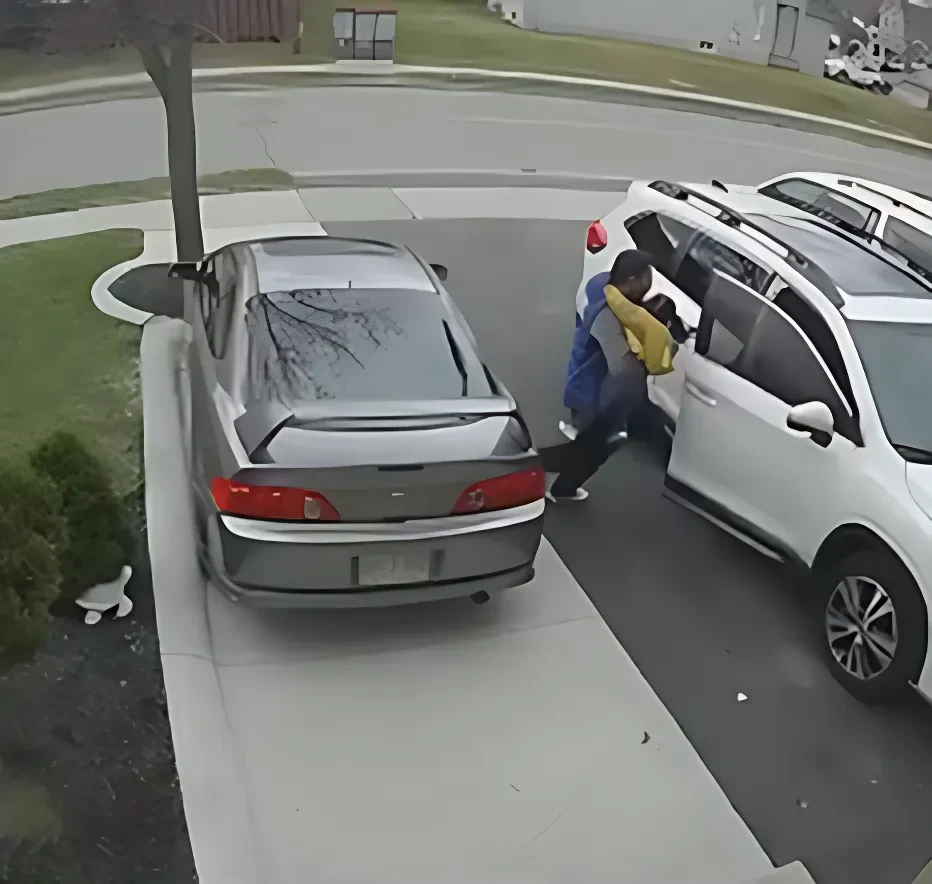
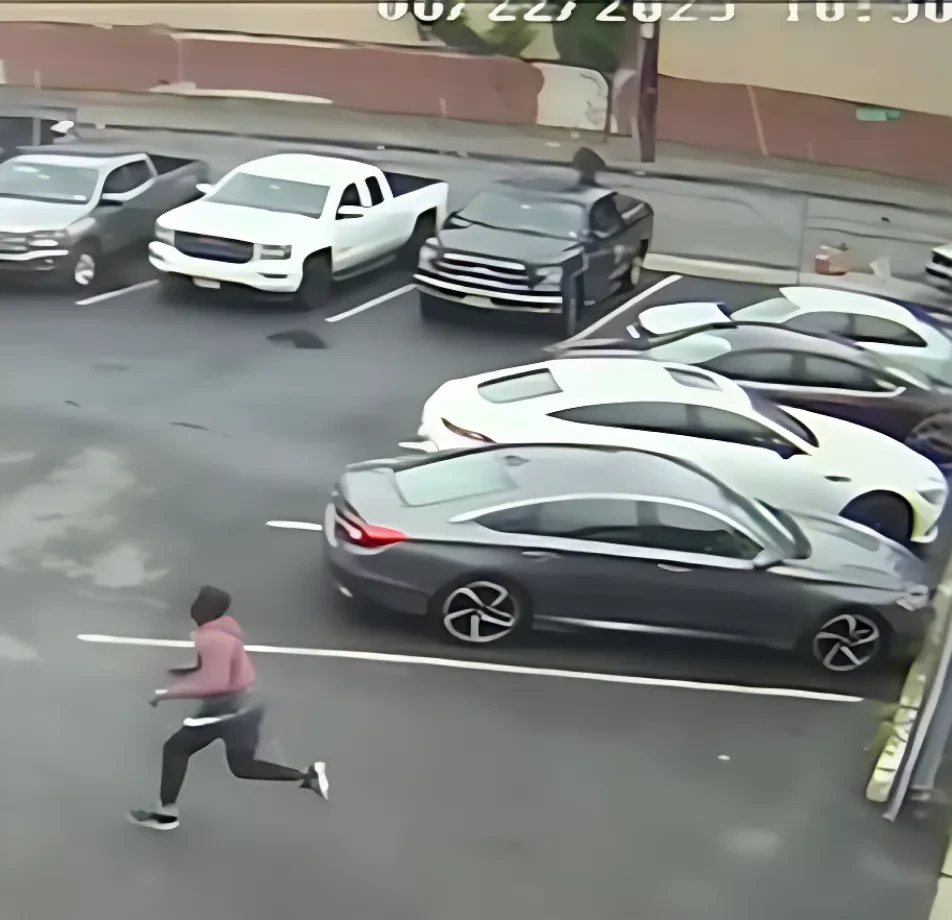
Sources
- Urban intersections and traffic signals.
- Highway traffic scenes.
- Suburban streets.
- Parking lots.
- Pedestrian crossings.
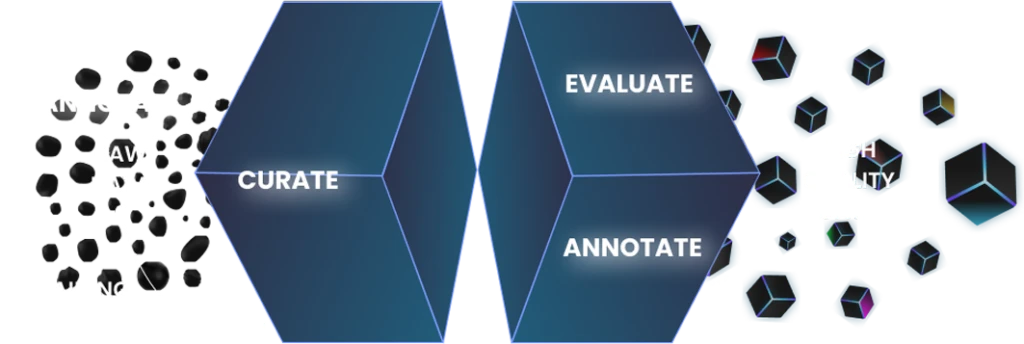
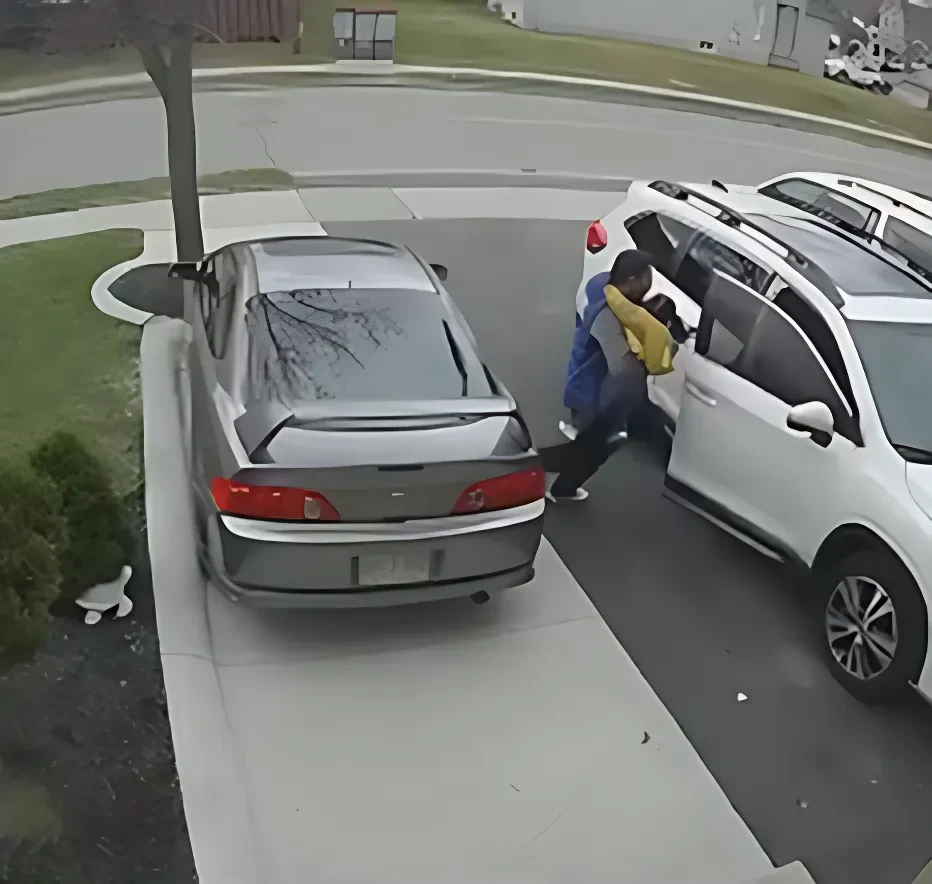
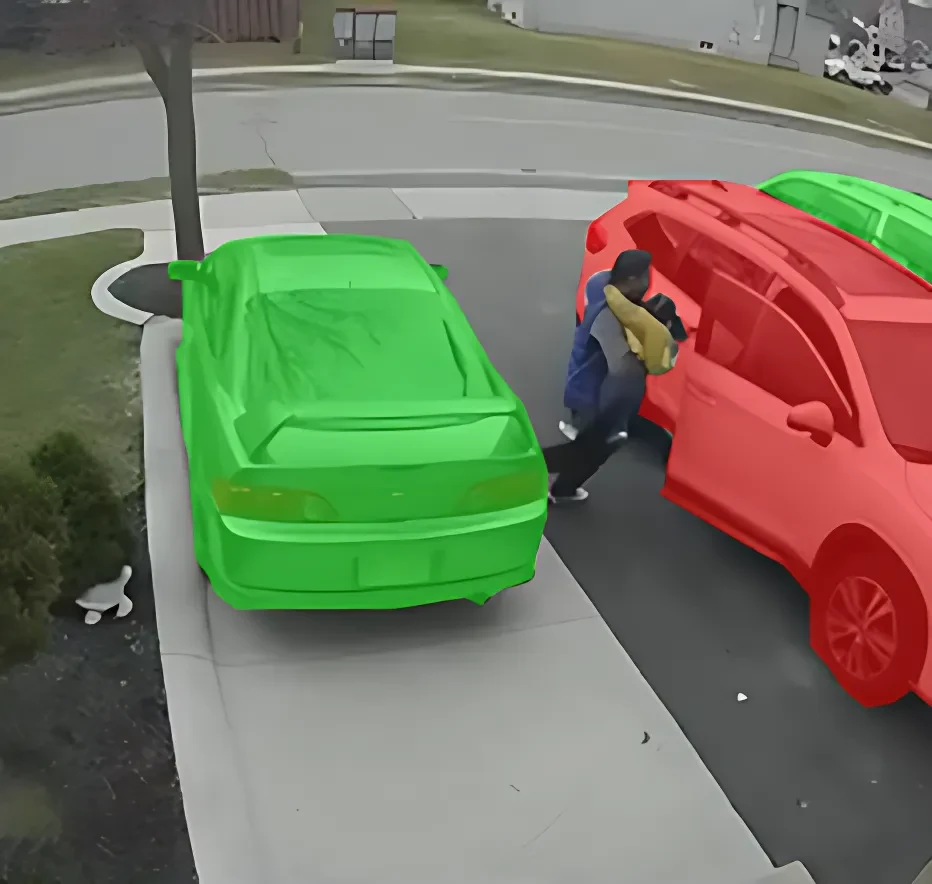
Data Collection Metrics
- Total Images: 100,000
- Urban Intersections: 40,000
- Highway Scenes: 30,000
- Suburban Streets: 15,000
- Parking Lots: 10,000
- Pedestrian Crossings: 5,000
Annotation Process
Stages
- Raw Image Pre-processing:Â Enhancing image quality, contrast, and removing noise.
- Pixel-wise Labeling:Â Manual and semi-automated annotation tools used to mark each pixel.
- Category Assignments: Pixels are categorized into classes like ‘Vehicle’, ‘Pedestrian’, ‘Road’, ‘Building’, ‘Tree’, etc.
- Validation:Â Cross-verification of annotations by separate annotators.
Annotation Metrics
- Total Annotations: 10 billion pixels (approximation)
- Vehicle Pixels: 3 billion
- Pedestrian Pixels: 1 billion
- Road Pixels: 2.5 billion
- Building Pixels: 2 billion
- Tree Pixels: 1.5 billion
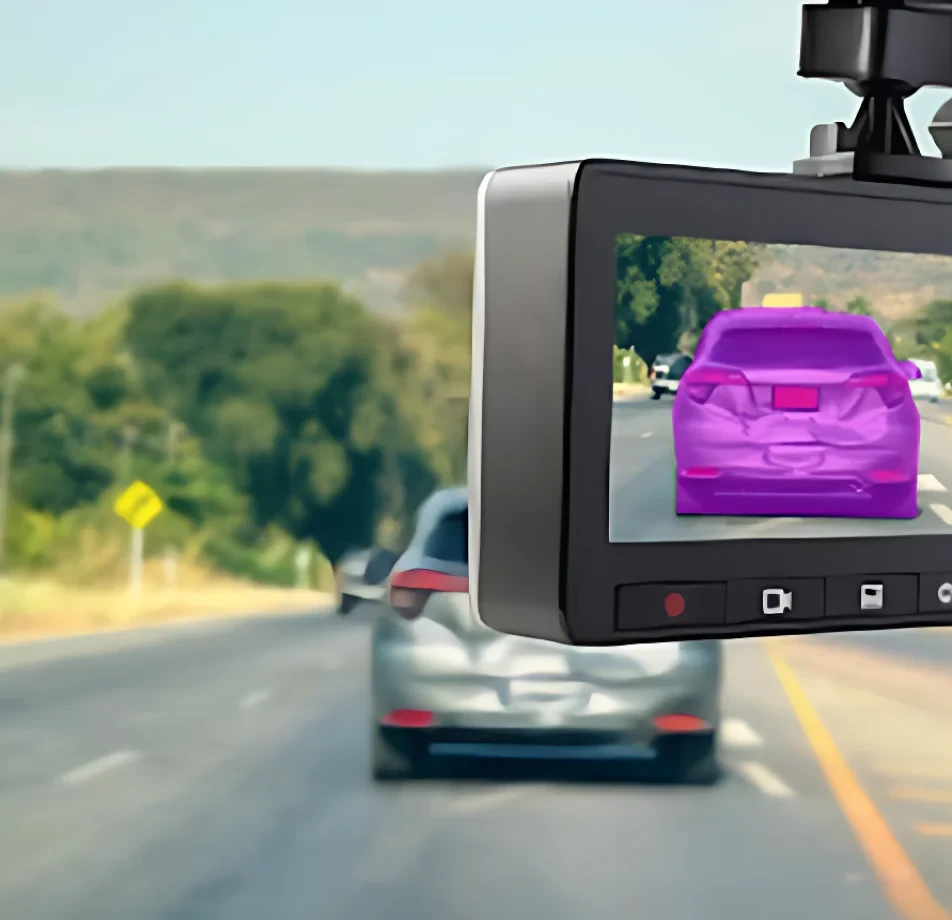
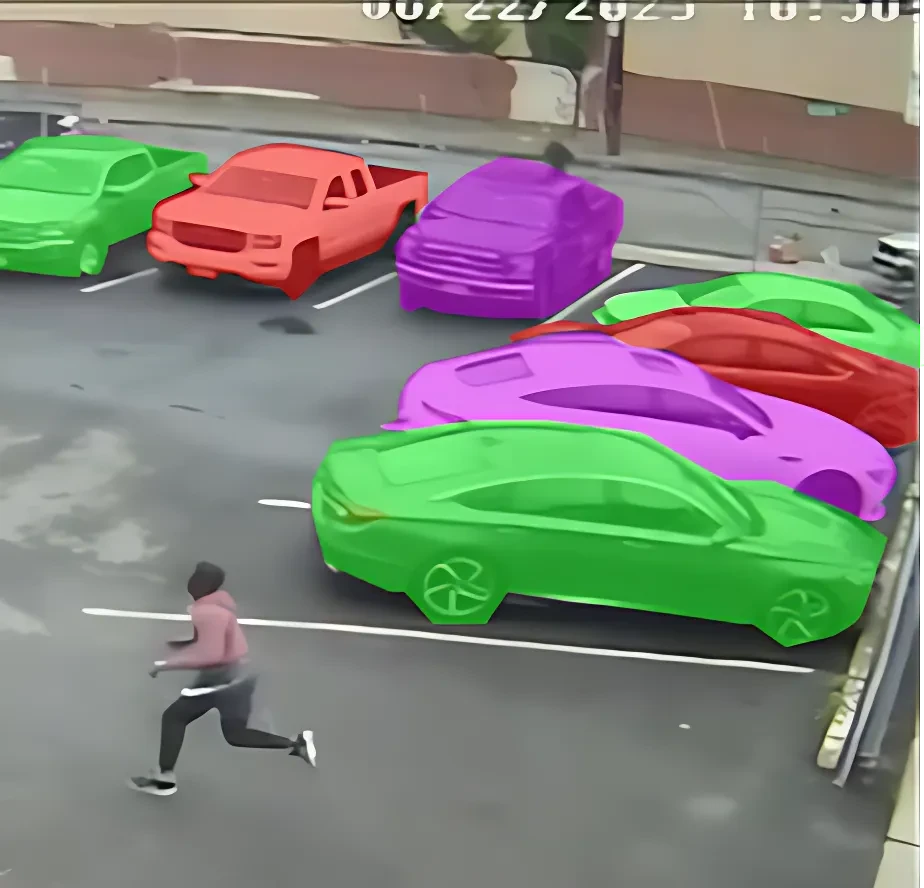
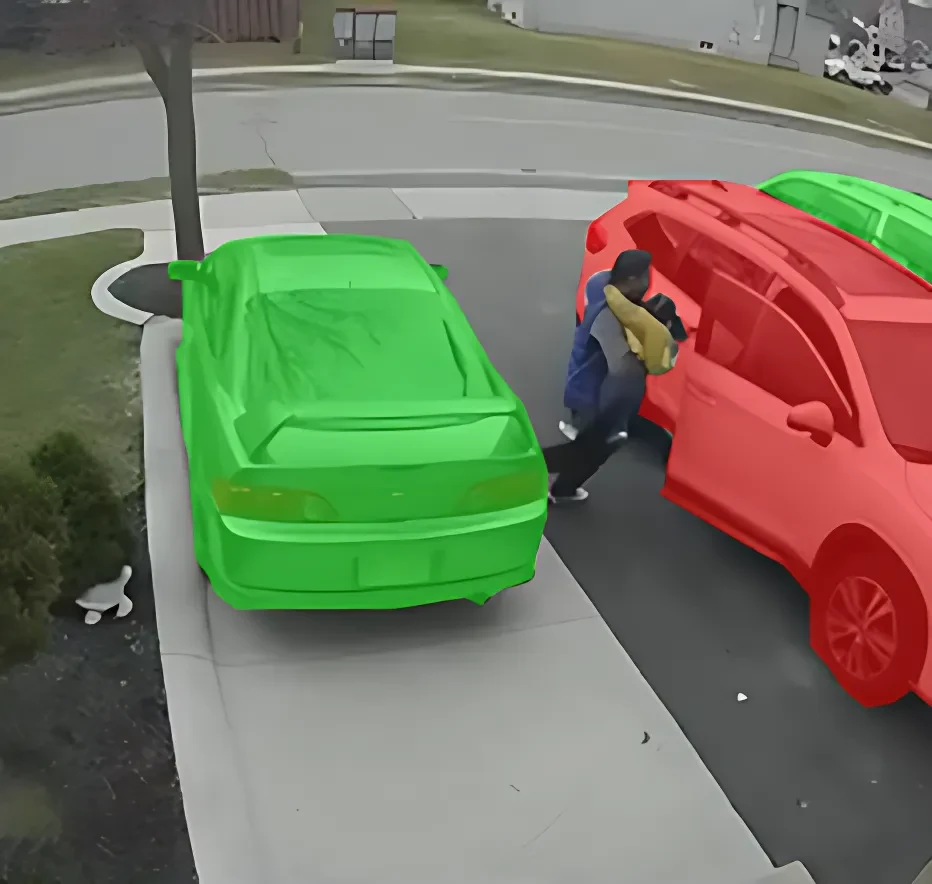
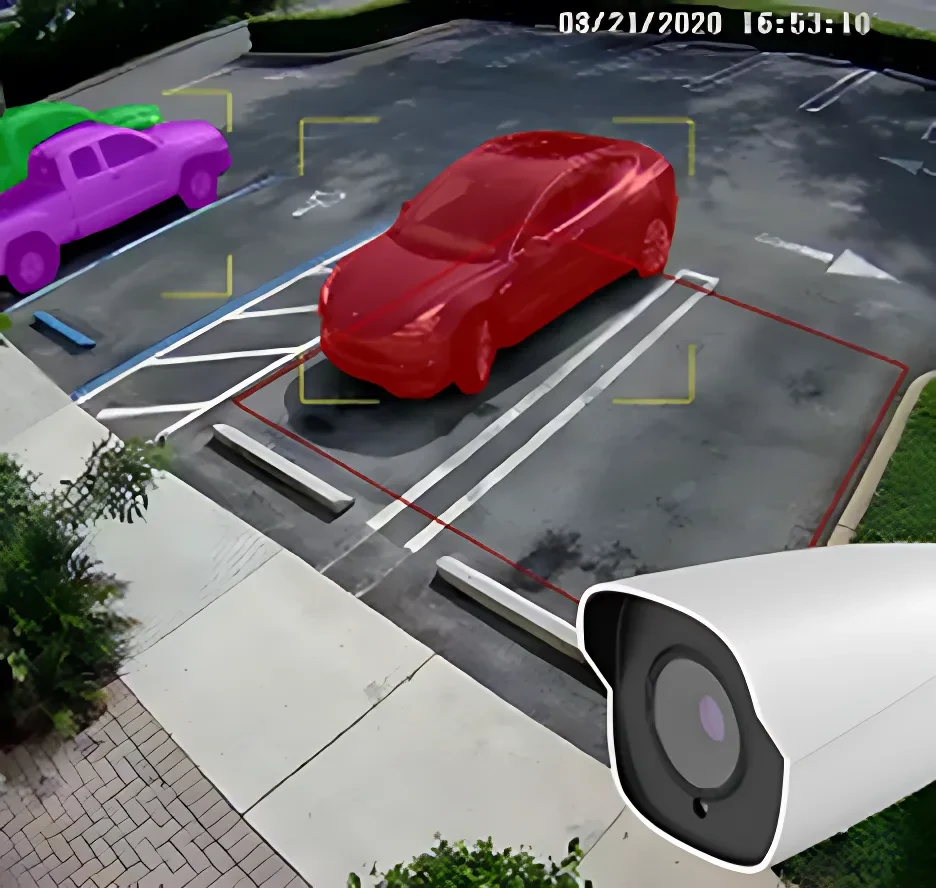
Quality Assurance
Stages
Automated Segmentation Models:Â Used pre-trained models to cross-verify manual annotations.
Expert Review:Â A team of experts reviewed challenging segments for accuracy.
Inter-annotator Agreement:Â Several annotators reviewed the same image, ensuring pixel-wise consistency.
QA Metrics
- Annotations Reviewed by Experts: 20 million pixels (0.2% of total annotations)
- Inconsistencies Identified and Rectified: 5 million pixels (0.05% of total annotations)
Conclusion
The CCTV Traffic Scene Semantic Segmentation Dataset has been meticulously crafted to ensure high precision in every pixel. It offers a comprehensive view of various traffic scenes, ensuring versatility and depth for researchers and developers in the field of computer vision.

Quality Data Creation

Guaranteed TAT

ISO 9001:2015, ISO/IEC 27001:2013 Certified

HIPAA Compliance

GDPR Compliance

Compliance and Security
Let's Discuss your Data collection Requirement With Us
To get a detailed estimation of requirements please reach us.