Autonomous Vehicle Driving Dataset
Home » Case Study » Autonomous Vehicle Driving Dataset
Project Overview:
Objective
Autonomous Vehicle Driving Dataset: The aim is to create a dataset that makes self-driving systems more accurate and efficient by using different types of real driving data.
Scope
The dataset contains various driving situations, environmental conditions, and how vehicles interact to simulate real-life driving accurately.
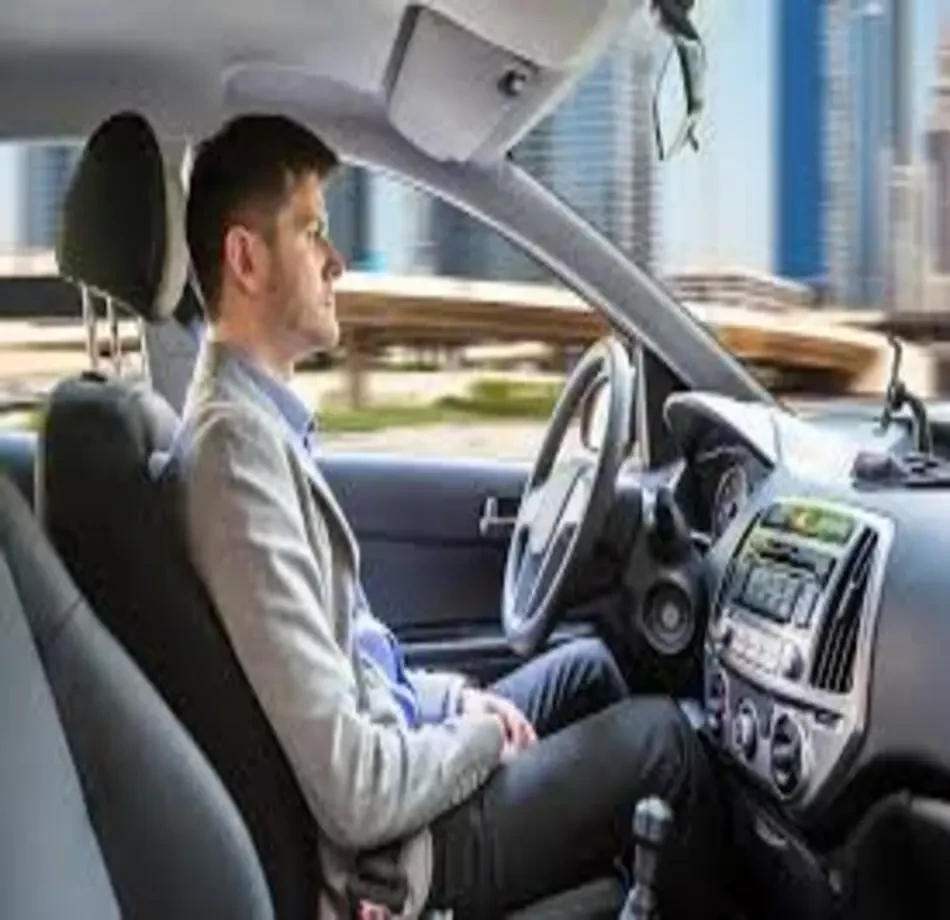
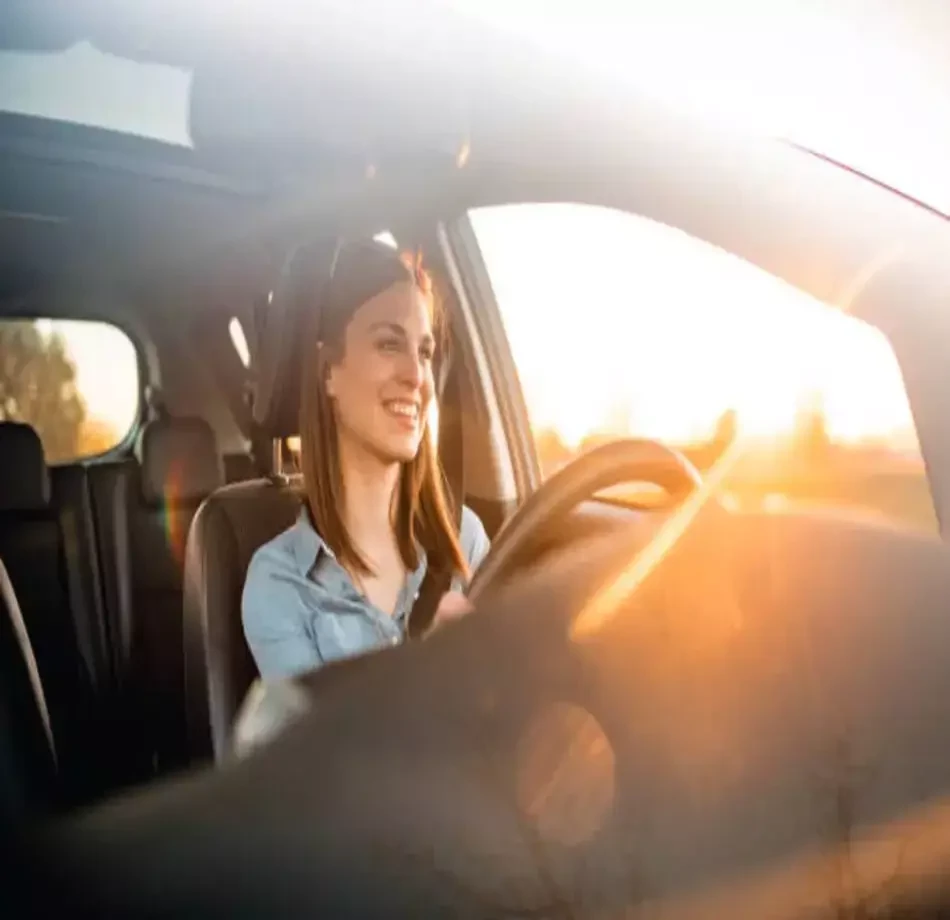
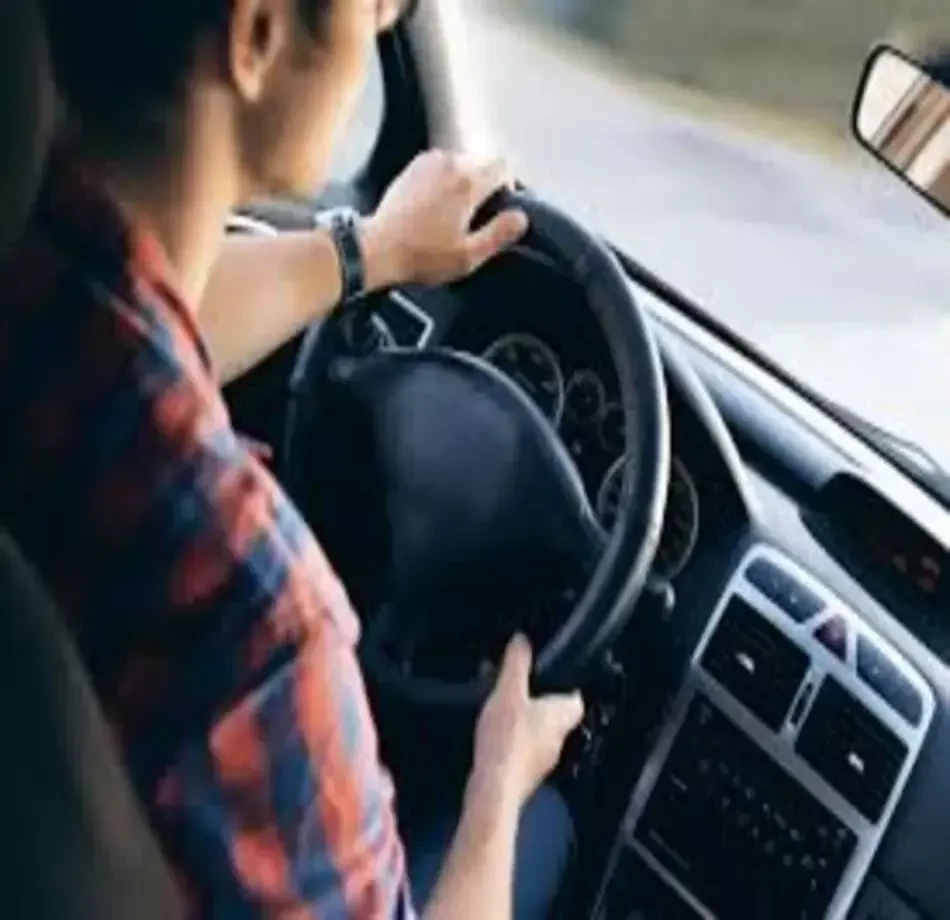
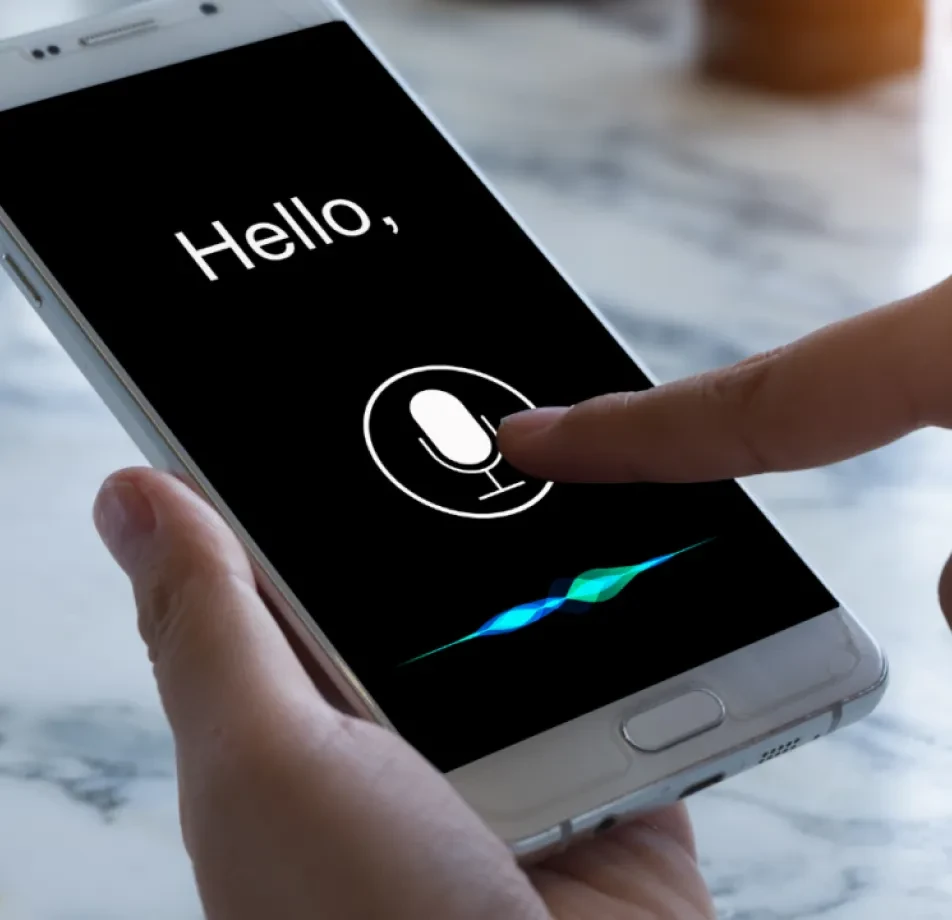
Sources
- Real Driving Sessions: We gathered information from real driving experiences, including different types of weather and various city and countryside locations.
- Simulated Environments: Using simulations, we obtained data on uncommon but important driving situations necessary for thorough testing of autonomous driving systems.
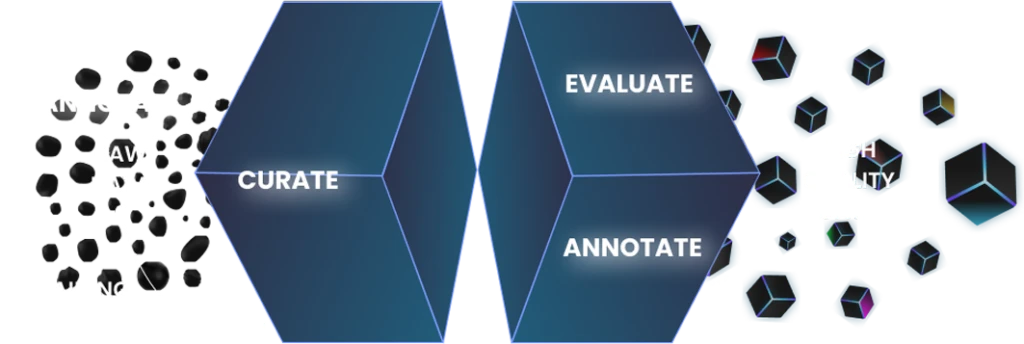
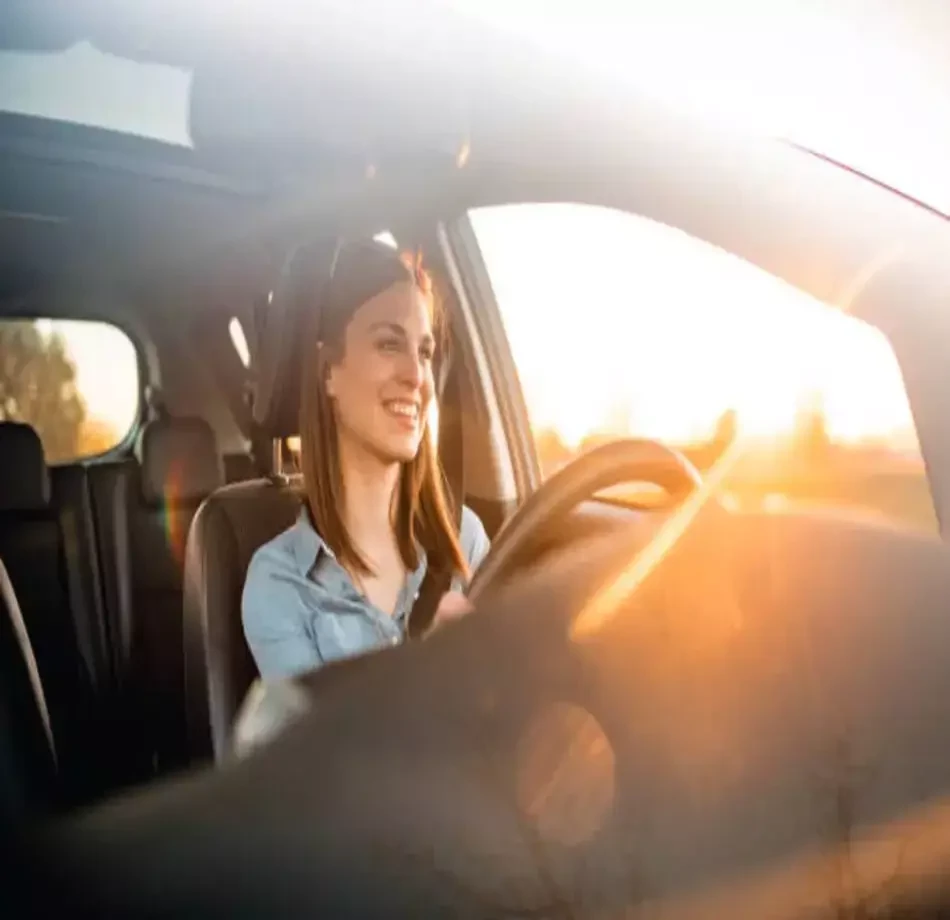
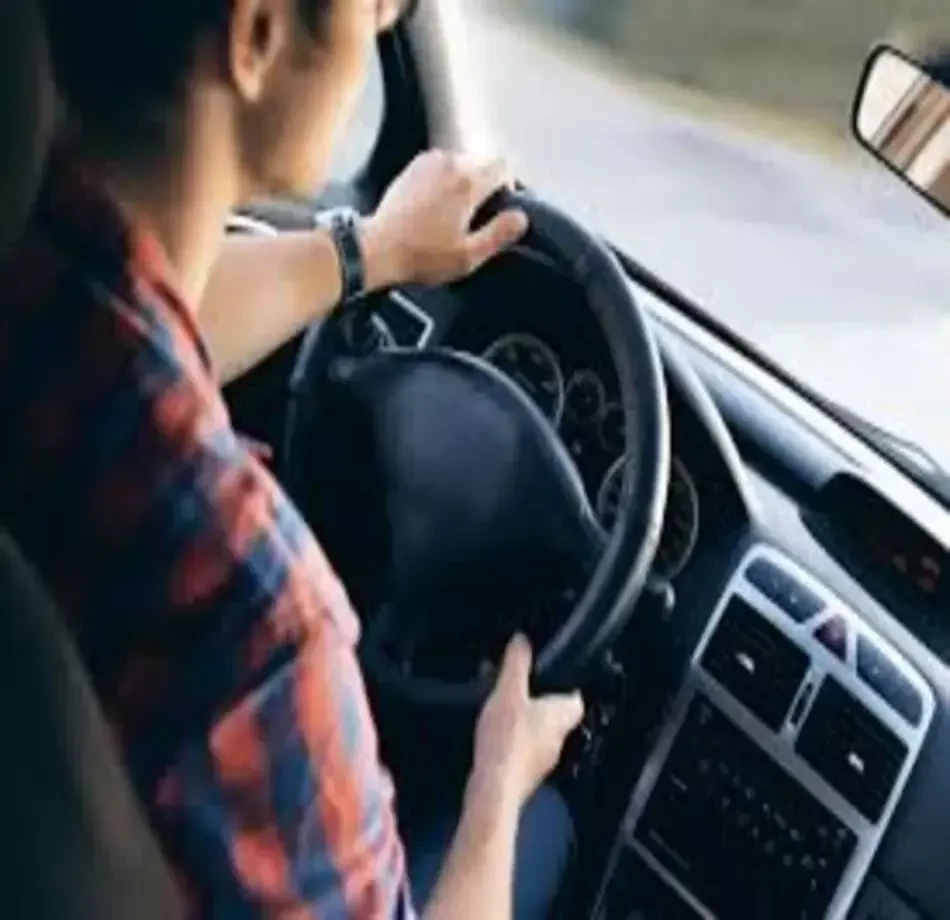
Data Collection Metrics
- Total Data Collected: 100,000 pictures and videos.
- Data Annotated for ML Training: 120,000 pictures and videos with detailed labels added for machine learning use.
Annotation Process
Stages
- Behavioral States: We labeled different actions like changing lanes, stopping, and how fast the vehicle accelerates.
- Object Tracking: We carefully marked every moving and still object in the scene, like other vehicles, people walking, and traffic signals.
- Scene Segmentation: We divided the scene into parts like roads, lanes, footpaths, and barriers to help understand how the car moves.
Annotation Metrics
- Annotated Behaviors: We’ve noted down 120,000 driving behaviors in detail.
- Object Labels: There are 110,000 labels tracking all objects in each scene.
- Segmentation Maps: We’ve created 100,000 maps showing the layout of different driving areas in detail.
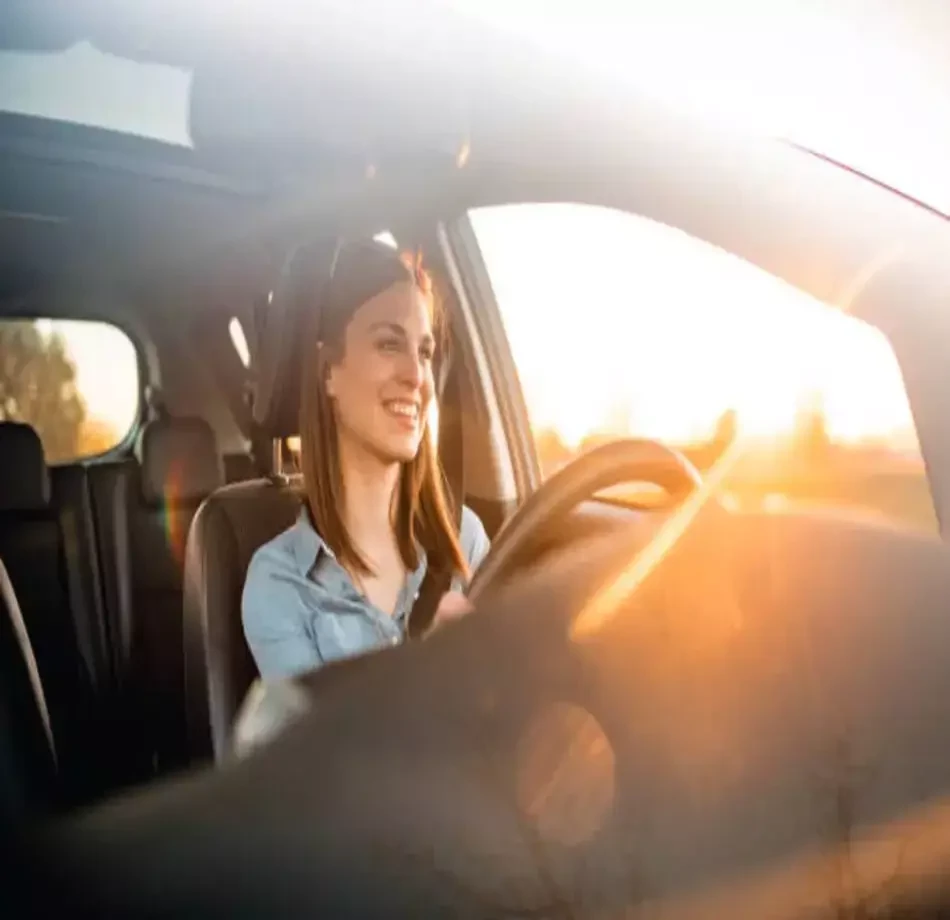
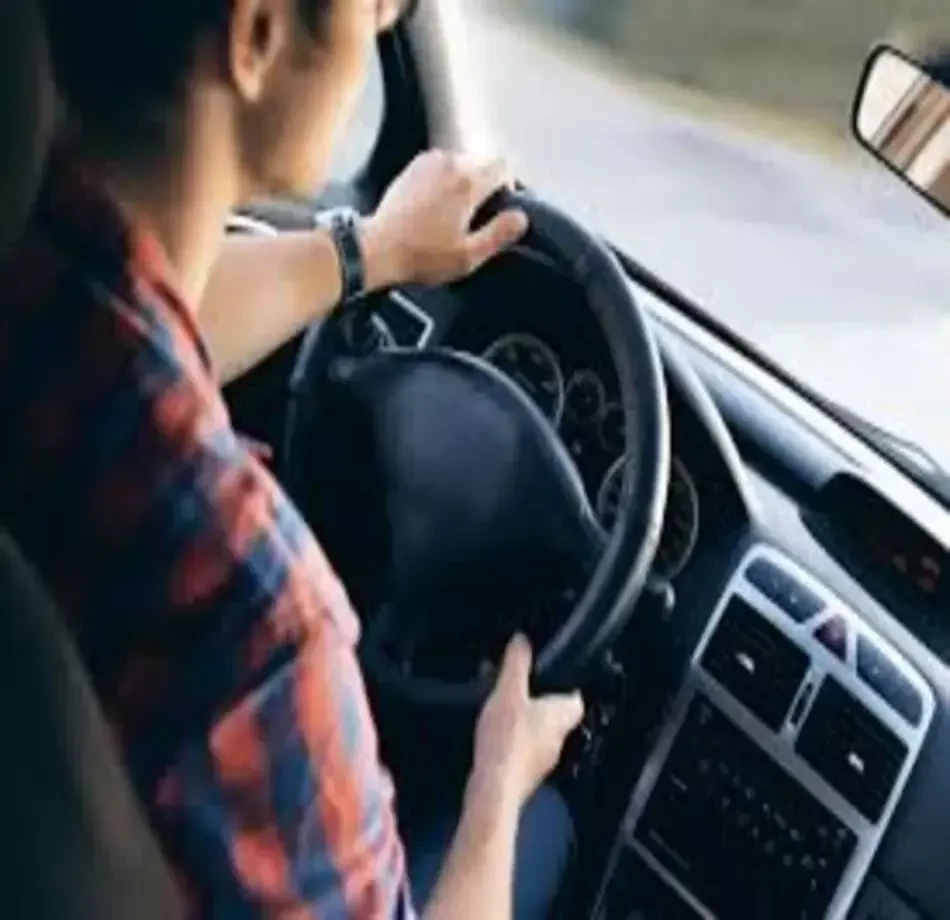
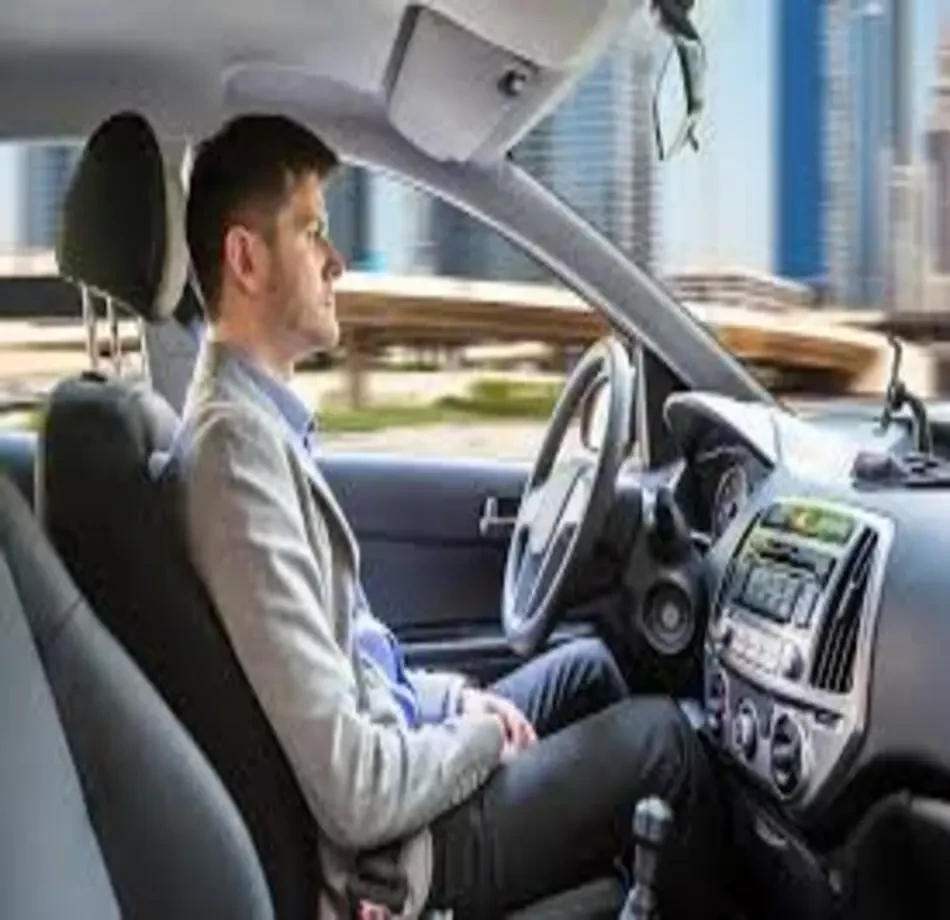
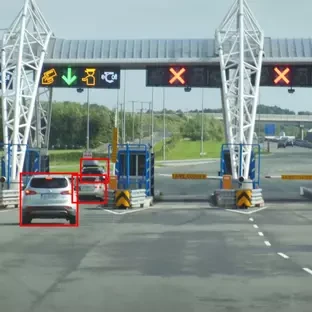
Quality Assurance
Stages
- Continuous Model Testing: We regularly test our dataset to make sure it’s accurate and useful for real-life driving situations.
- Privacy and Security: We follow strict privacy laws to make sure all the data we collect is anonymous and collected responsibly.
- Improvement Process: We listen to feedback from how well our dataset works and use it to make our data collection and labeling better.
QA Metrics
- Behavior Recognition Accuracy: We correctly identified detailed driver behaviors with a high accuracy of 97%.
- Object Detection Accuracy: We successfully detected and tracked different objects with an accuracy of 95%.
- Privacy Compliance: We followed all international rules about data protection and privacy, achieving 100% compliance.
Conclusion
The creation of the KITTI Vision Benchmark dataset is a big step forward in self-driving car technology. It gives a thorough and very accurate view of different driving situations, which is important for teaching advanced and safe self-driving systems.

Quality Data Creation

Guaranteed TAT

ISO 9001:2015, ISO/IEC 27001:2013 Certified

HIPAA Compliance

GDPR Compliance

Compliance and Security
Let's Discuss your Data collection Requirement With Us
To get a detailed estimation of requirements please reach us.