Audio Datasets for Healthcare & Conversational AI
Home » Case Study » Audio Datasets for Healthcare & Conversational AI
Project Overview:
Objective
To compile a multifaceted audio dataset specifically designed for applications in Healthcare and Conversational AI, thereby facilitating advanced recognition, comprehension, and interaction capabilities.
Scope
To compile a multifaceted audio dataset specifically tailored for applications in Healthcare and Conversational AI, thus enabling enhanced recognition, comprehension, and interaction capabilities.
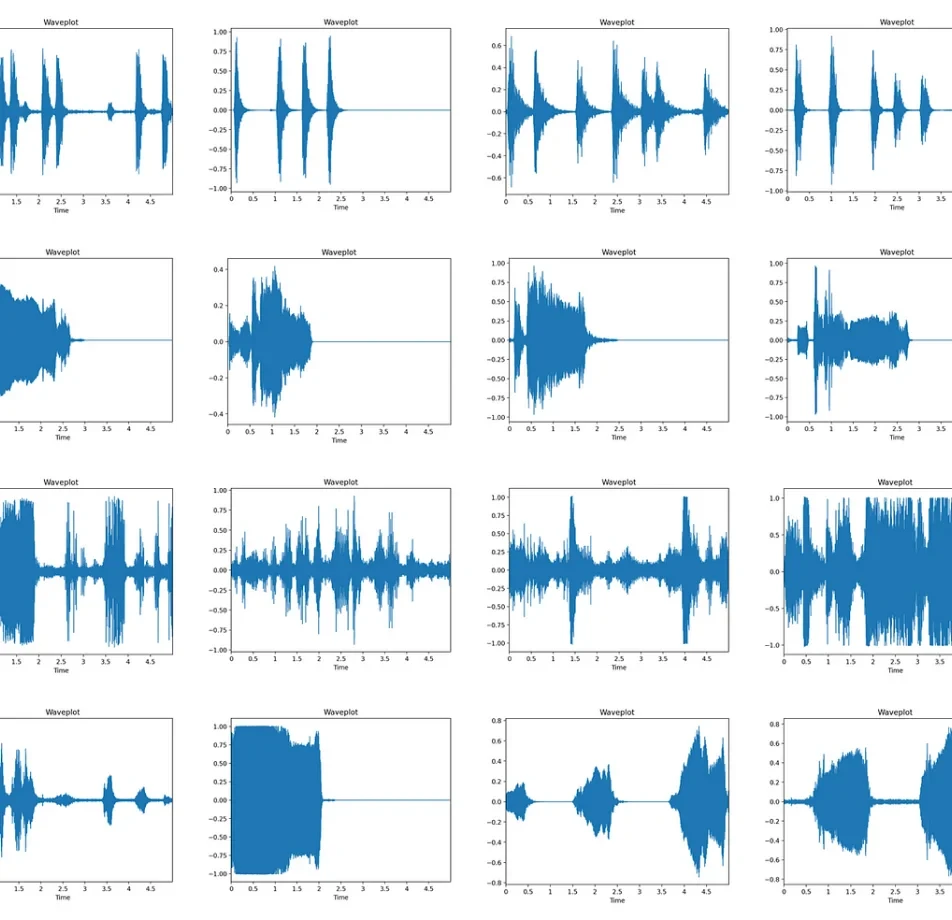
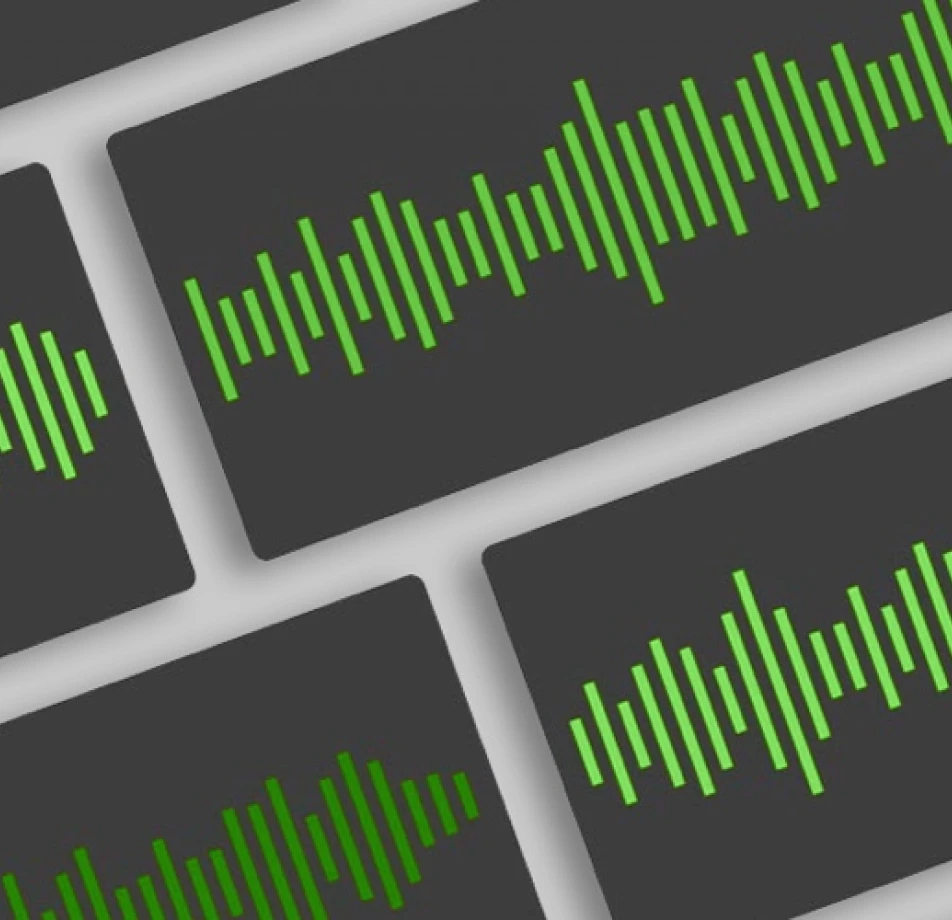
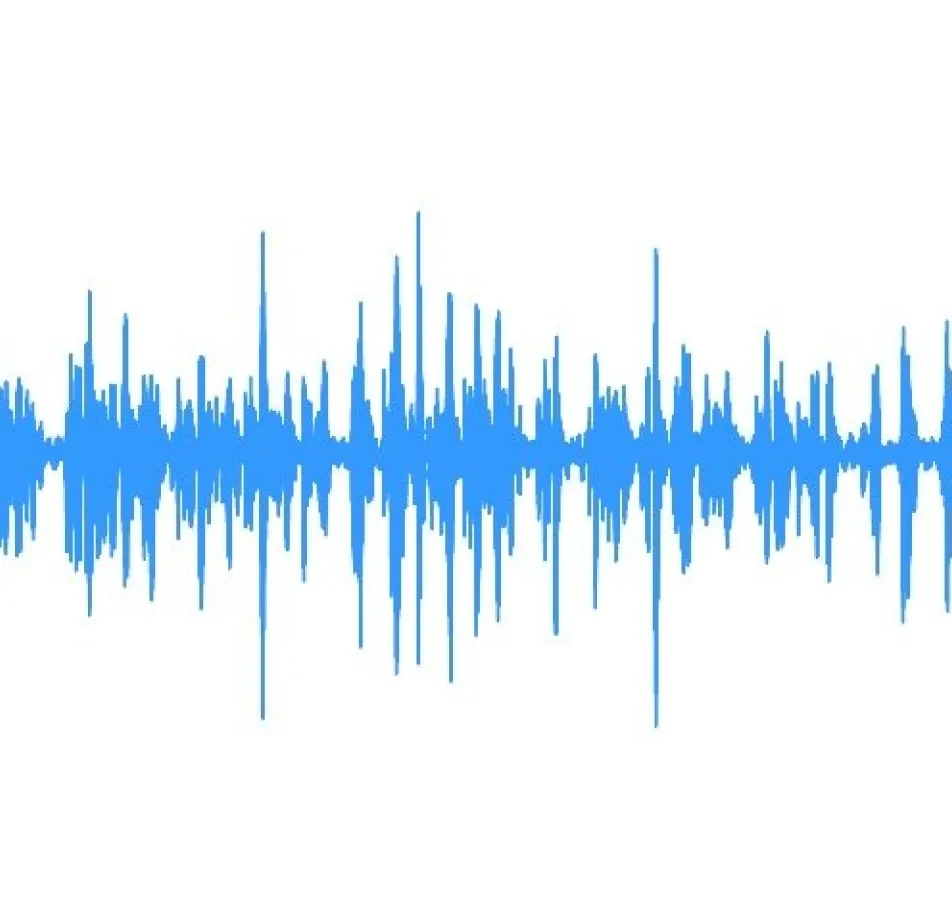
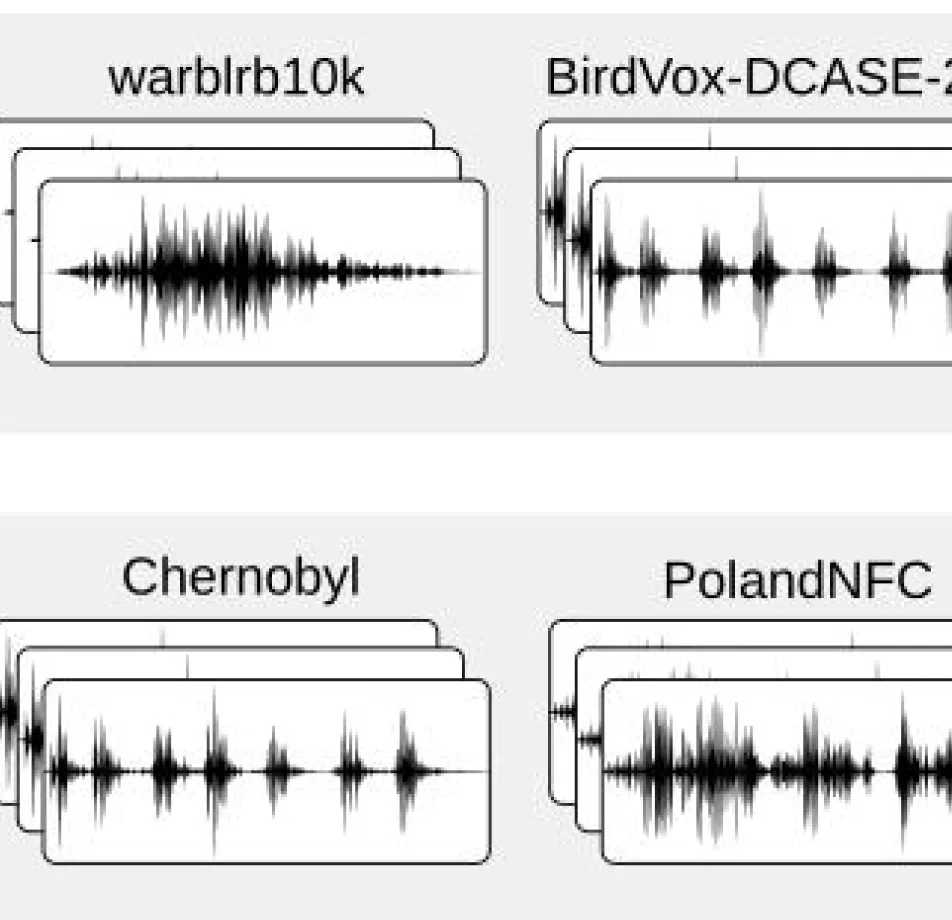
Sources
- Electronic Health Records (EHRs) represent a crucial component, enabling the comprehensive accumulation of patient histories. Furthermore, EHRs facilitate seamless access to patient data, ensuring efficient management and continuity of care.
- Patient Intake Forms: Additionally, these forms captured essential patient-reported information, ensuring that healthcare providers have accurate initial data.
- Laboratory Test Results: Furthermore, these results productively provided key data points from various medical tests, contributing to informed medical decisions.
- Clinical Trial Data: In addition, this data offered insights from controlled medical studies, thereby enhancing the understanding of treatment efficacy.
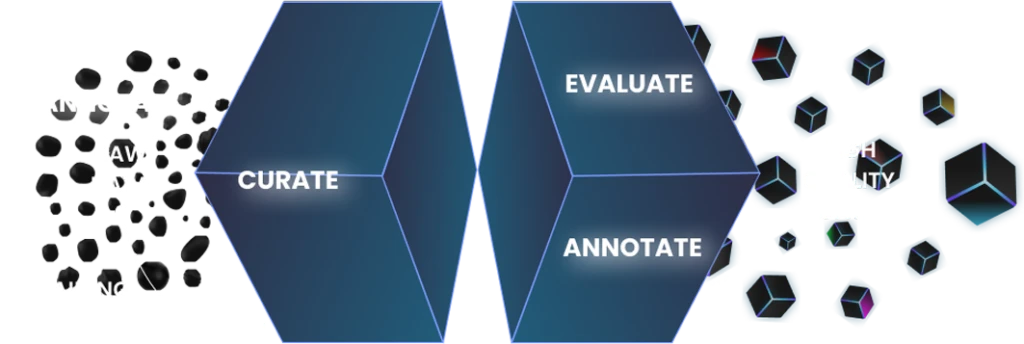
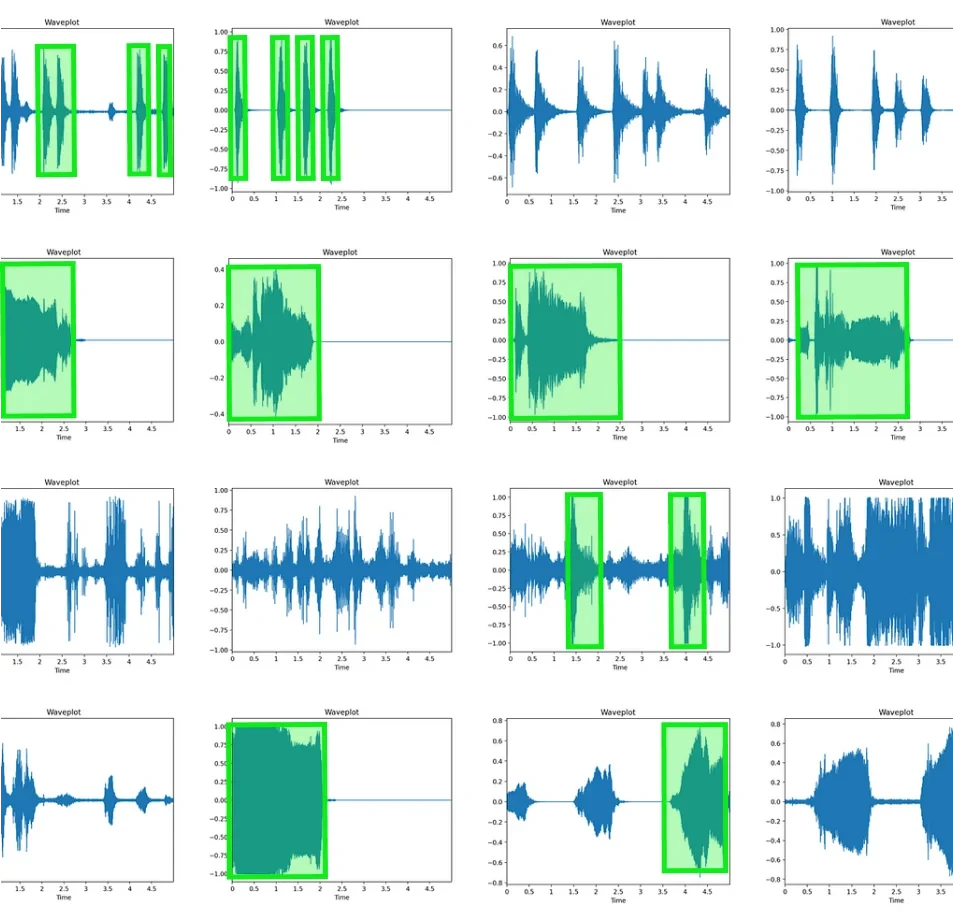
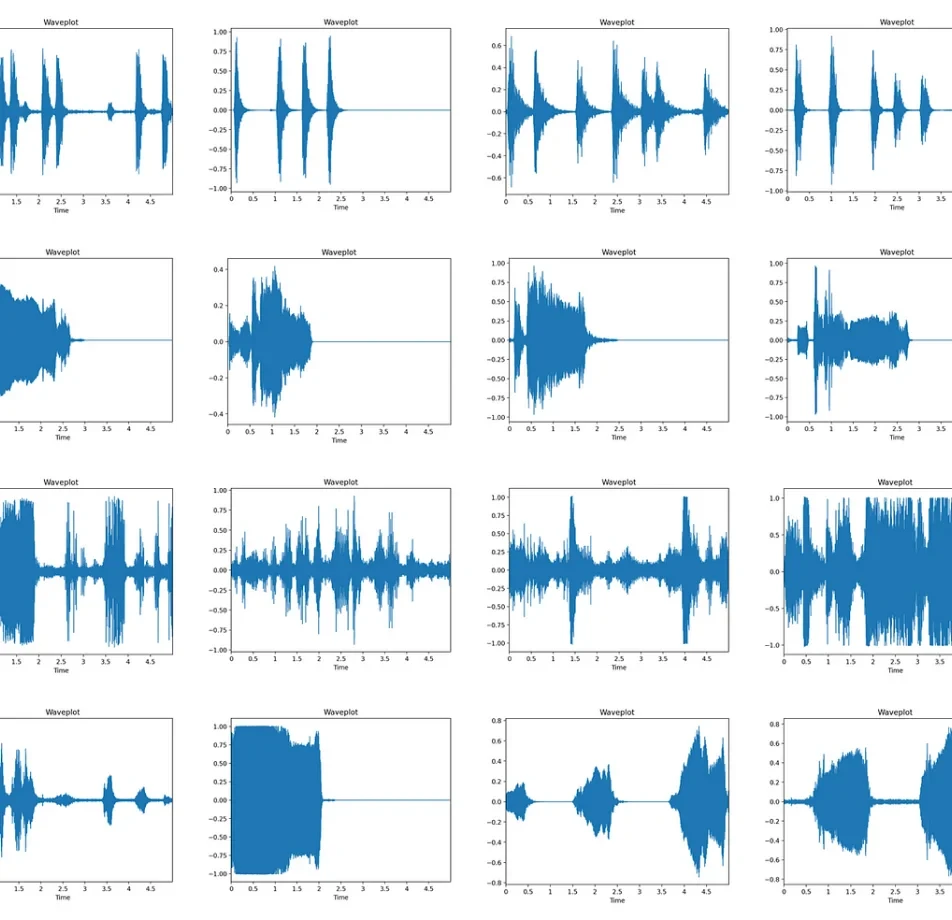
Data Collection Metrics
- Total Audio Samples: 200,000
- Patient-Doctor Consultations: 70,000
- Health Helpline Recordings: 30,000
- Medical Seminars and Lectures: 40,000
- AI-User Interactions: 40,000
- General Conversational Samples: 20,000
Annotation Process
Stages
- Noise Reduction: Filtering out background noise and enhancing voice clarity are crucial for achieving high-quality audio recordings.
- Segmentation: Breaking down long recordings into meaningful chunks can significantly enhance the listening experience and improve comprehension.
- Transcription: Converting audio data into textual representation can be a complex process.
- Entity Recognition: Highlighting medical terms, drug names, conditions, etc.
- Intent Recognition: Categorizing the purpose behind each conversational interaction.
Annotation Metrics
- Total Annotations: 600,000 (3 annotations per audio sample on average)
- Noise-reduced Samples: 180,000
- Segmented Chunks: 550,000
- Transcribed Samples: 200,000
- Entity Tags: 150,000
- Intent Tags: 100,000
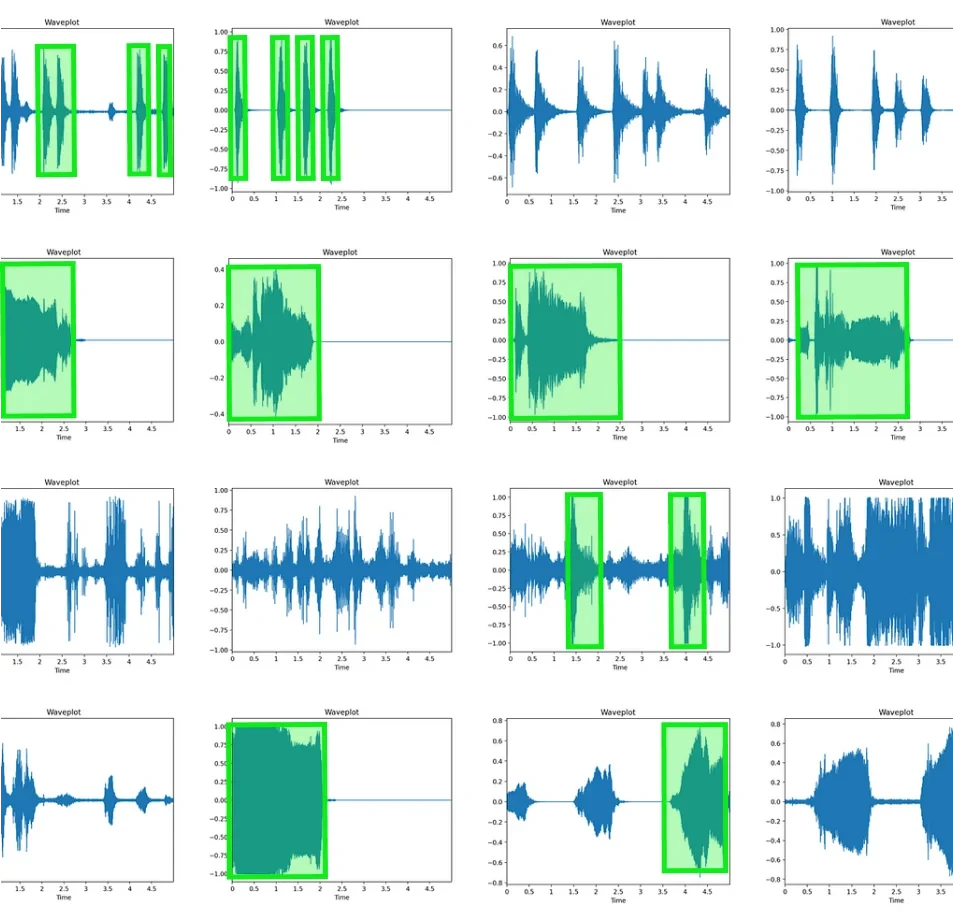
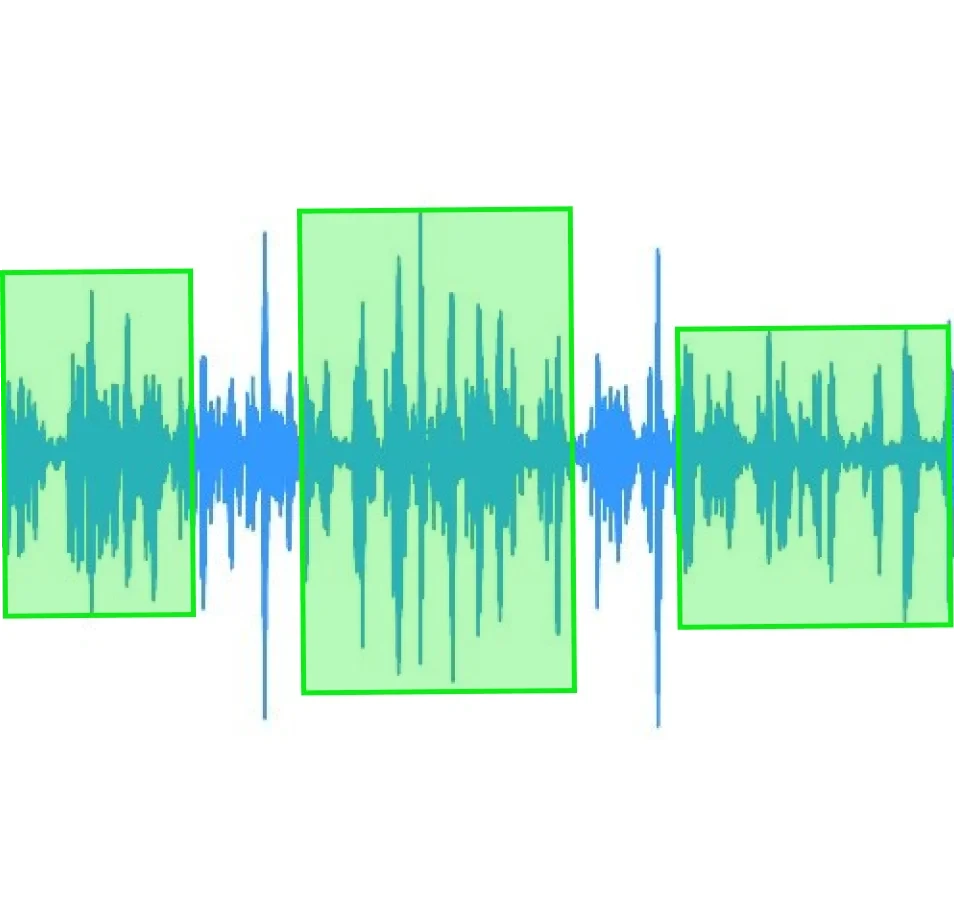
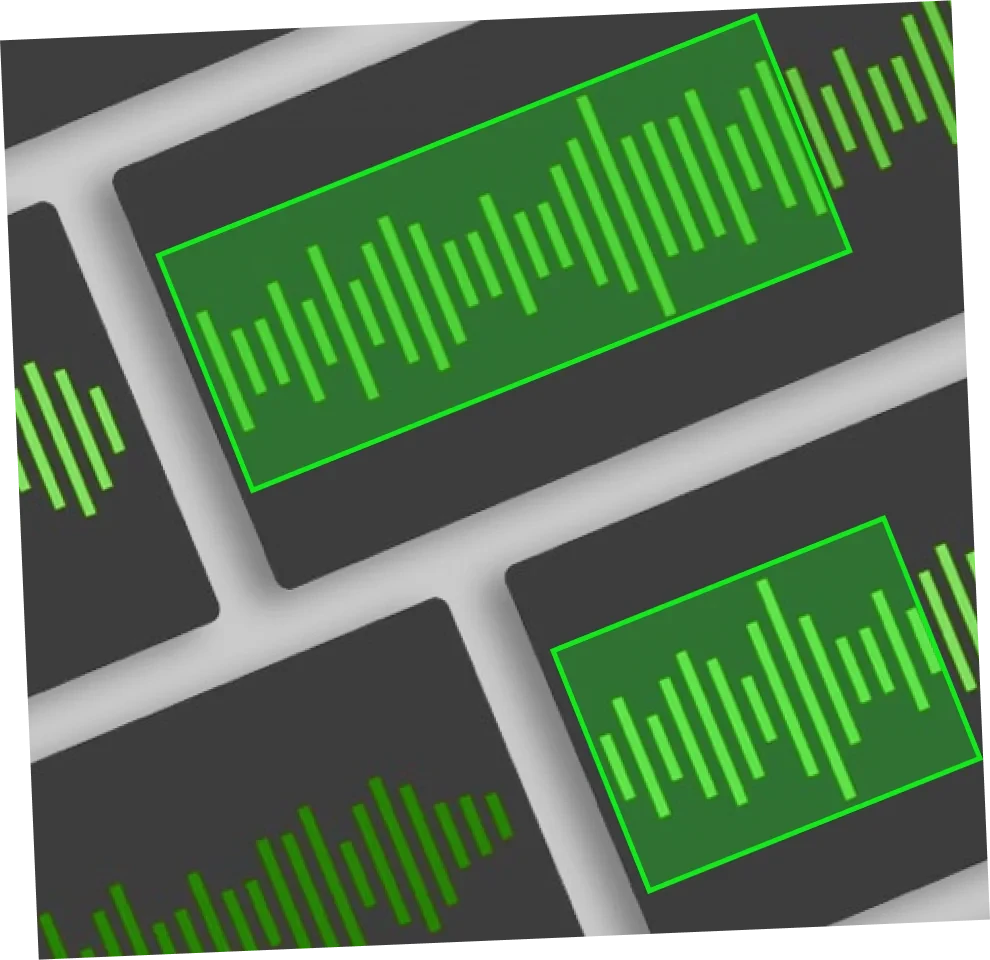
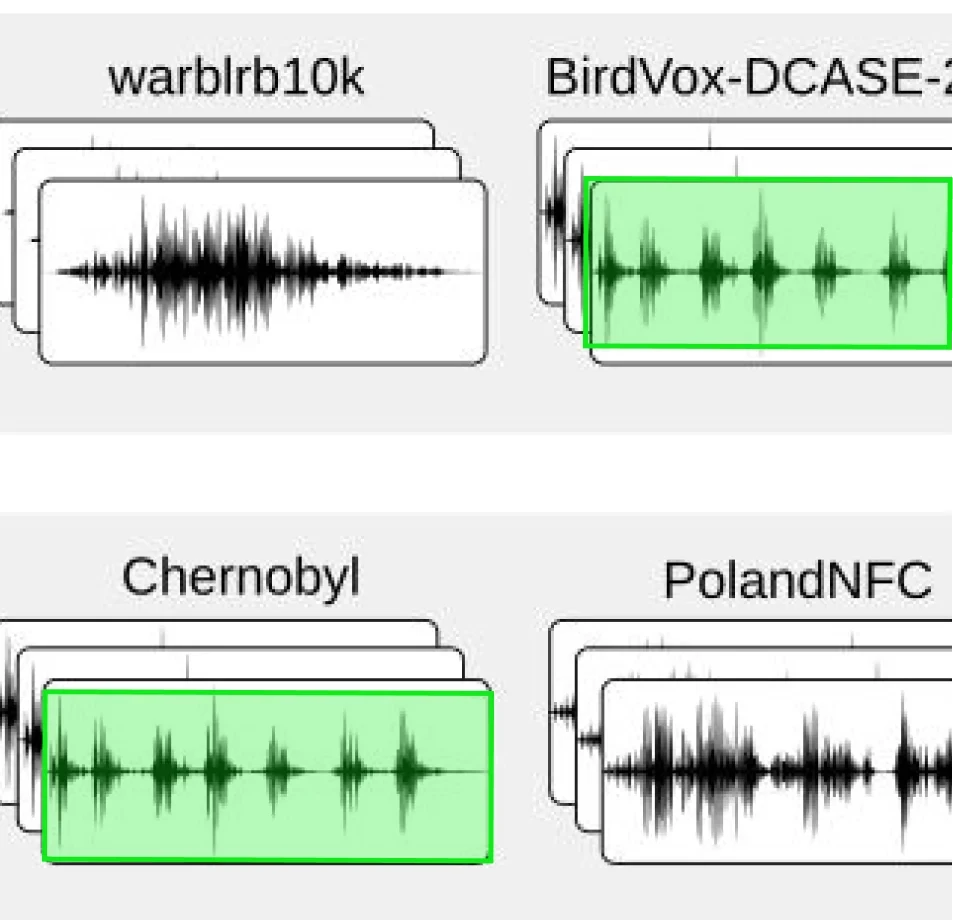
Quality Assurance
Expert Review:Medical professionals and linguistic experts meticulously reviewed the annotations for accuracy. Furthermore, they conducted thorough assessments to ensure the precision of the annotations.
Moreover, Consistency Checks: Automated checks to identify anomalies or inconsistencies in transcriptions are essential for ensuring data accuracy and reliability.
Additionally, Inter-annotator Agreement: Multiple annotators assessed a portion of the data to ensure consistent and reliable annotations.
QA Metrics
- Annotations Reviewed by Experts: 60,000 (10% of total annotations)
- Inconsistencies Identified and Corrected: 12,000 (2% of total annotations)
Conclusion
This ambitious undertaking, consequently, has yielded a robust audio dataset meticulously curated specifically for healthcare and conversational AI applications. Through meticulous collection and annotation, therefore, the dataset is poised to significantly bolster advancements in AI-driven healthcare solutions and conversational platforms alike.

Quality Data Creation

Guaranteed TAT

ISO 9001:2015, ISO/IEC 27001:2013 Certified

HIPAA Compliance

GDPR Compliance

Compliance and Security
Let's Discuss your Data collection Requirement With Us
To get a detailed estimation of requirements please reach us.