Fake-Vs-Real-Faces (Hard)
Fake-Vs-Real-Faces (Hard)
Datasets
Fake-Vs-Real-Faces (Hard)
File
Fake-Vs-Real-Faces (Hard)
Use Case
Fake-Vs-Real-Faces (Hard)
Description
Explore the Real vs. Fake Human Faces Dataset with 1,288 high-quality images (300x300 resolution). Includes StyleGAN2-generated fake faces and diverse real faces, ideal for AI research, deep learning, and face classification tasks
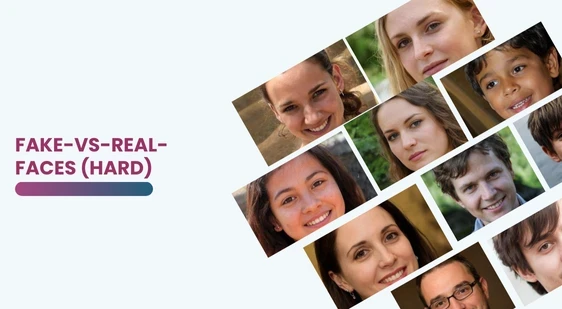
Description:
A challenging dataset of 1,288 images (700 fake, 589 real) for AI research. Fake faces are generated using StyleGAN2, while real faces feature diverse attributes like age, sex, and ethnicity. All images are 300×300 JPEGs, perfect for training and testing face classification models.
Download Dataset
This dataset is designed to challenge and advance the capabilities of machine learning models in classifying real and fake human faces. Unlike most available datasets that lack variance, this dataset ensures a diverse range of features for real faces and uses StyleGAN2-generated fake faces, creating a more difficult classification task, even for the human eye.
Key Features
✅ Challenging Classification Task: Includes high-quality, realistic fake faces generated by StyleGAN2, raising the difficulty for accurate classification.
✅ Diverse Real Faces: Real human faces are gathered with attention to age, sex, ethnicity, makeup, and other features, ensuring a realistic and fair representation for production scenarios.
✅ Uniform Image Format: All images are in JPEG format with a consistent resolution of 300×300 pixels for seamless AI training.
✅ Balanced Dataset: Contains a total of 1,288 images:
- Fake Faces: 700 images (collected from thispersondoesnotexist.com).
- Real Faces: 589 images (extracted via the Unsplash API and processed using OpenCV).
Dataset Contents
- Images: 1,288 labeled JPEG images.
- Labels: A
data.csv
file mapping image IDs to their respective labels (Fake or Real).
Applications
- Deep Learning Model Development: Train neural networks for face classification tasks.
- AI for Fraud Detection: Detect GAN-generated fake faces in real-world applications.
- Facial Recognition Research: Enhance the robustness of AI systems in handling diverse features and synthetic face detection.
This dataset is sourced from Kaggle.
Contact Us

Quality Data Creation

Guaranteed TAT

ISO 9001:2015, ISO/IEC 27001:2013 Certified

HIPAA Compliance

GDPR Compliance

Compliance and Security
Let's Discuss your Data collection Requirement With Us
To get a detailed estimation of requirements please reach us.