Recipe Annotation for Food & Beverage LLM
Project Overview:
Objective
The goal was to create a detail dataset that enhances LLMs’ ability to interpret culinary content, including the identification of ingredients and the sequencing of cooking instructions. This would enable more accurate and useful AI-driven applications in the culinary space.
Scope
The dataset encompasses a wide range of recipes from various cuisines and dietary preferences. Each recipe is meticulously annotate to ensure the clear identification of ingredients and cooking steps, facilitating the development of advanced LLM capabilities in the food and beverage domain.
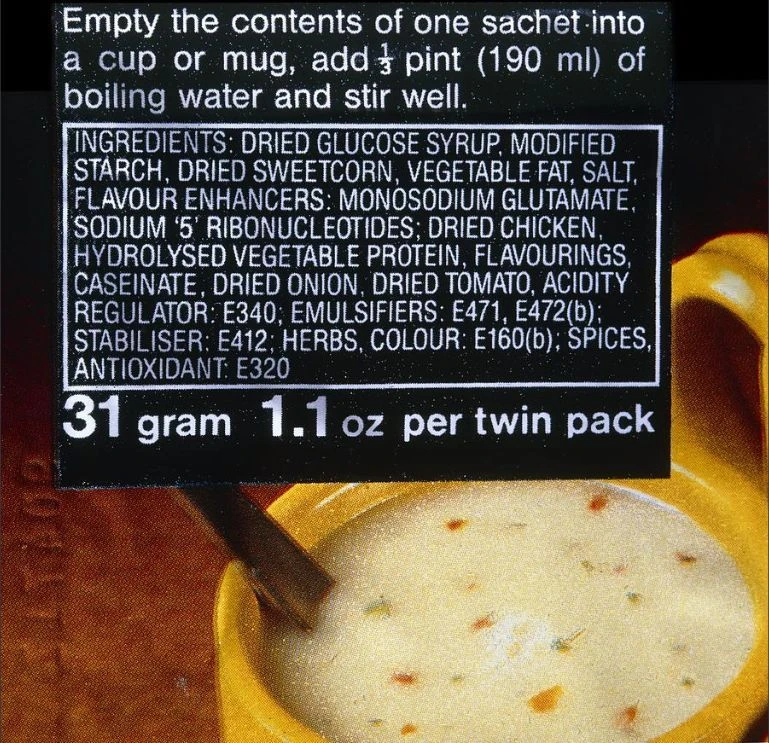
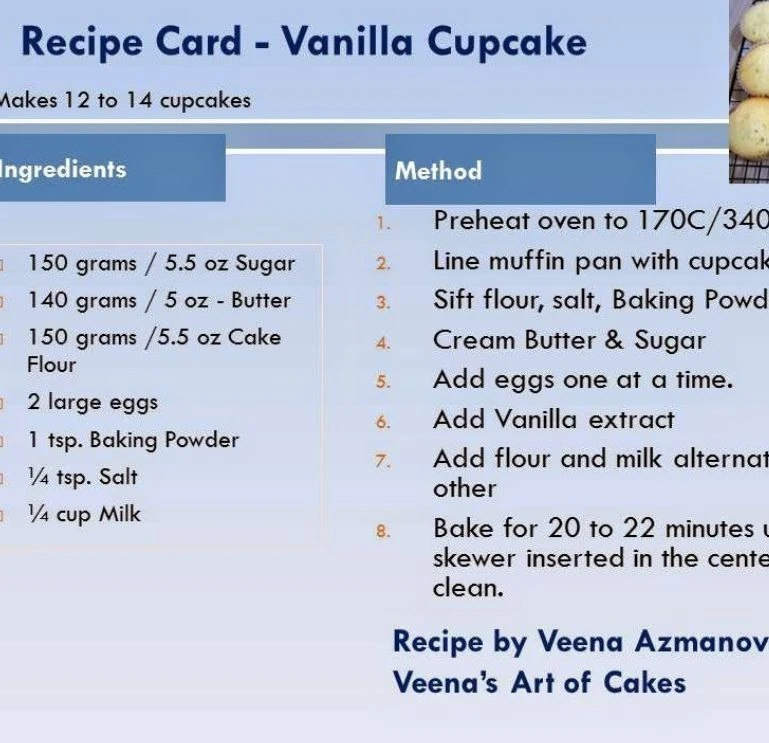
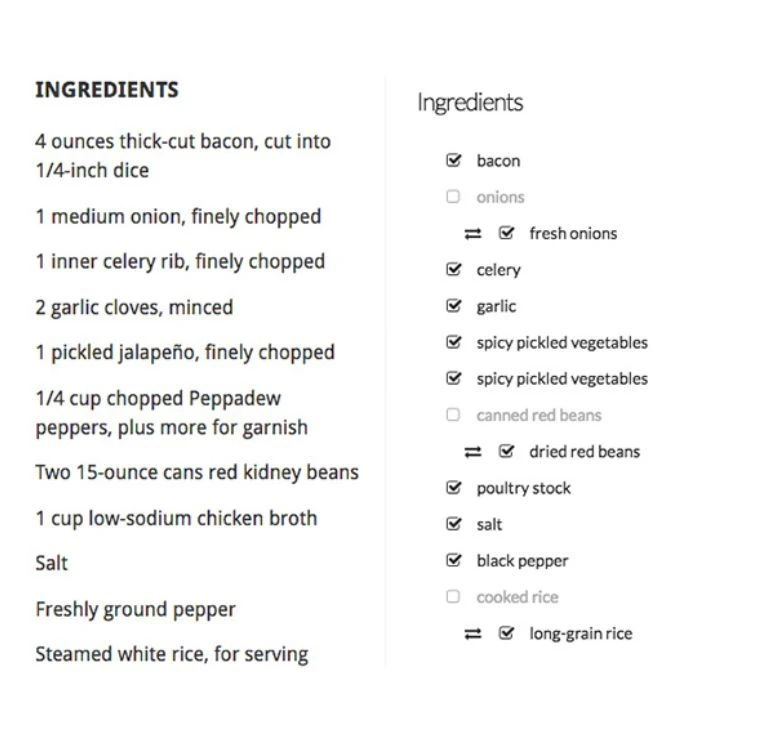
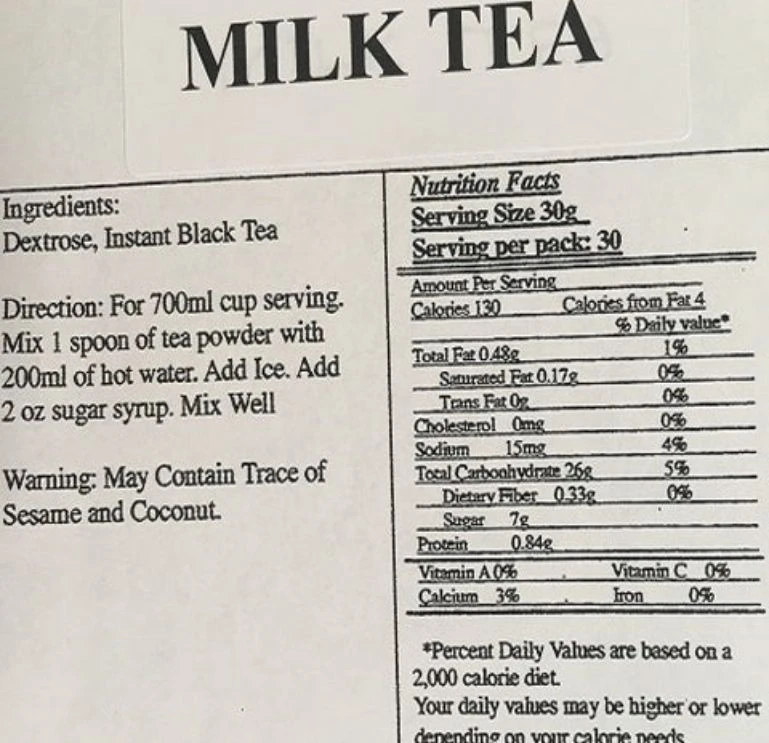
Sources
- Online Recipe Platforms: Data was source from 15,000 recipes collect from diverse online platforms, covering a broad spectrum of cuisines and dietary needs.
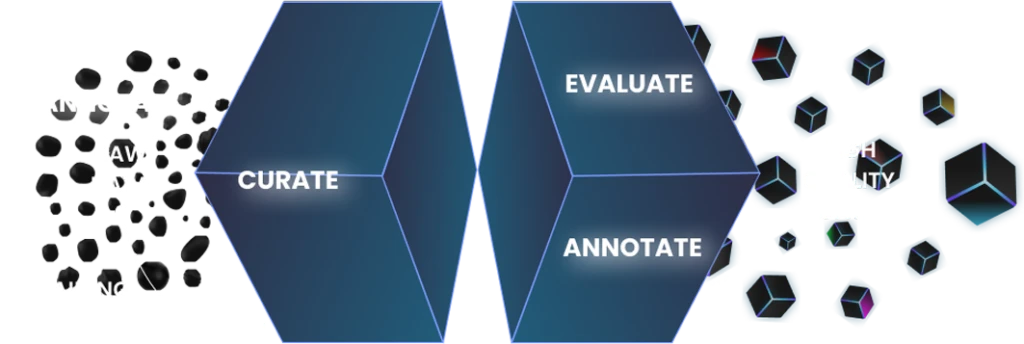
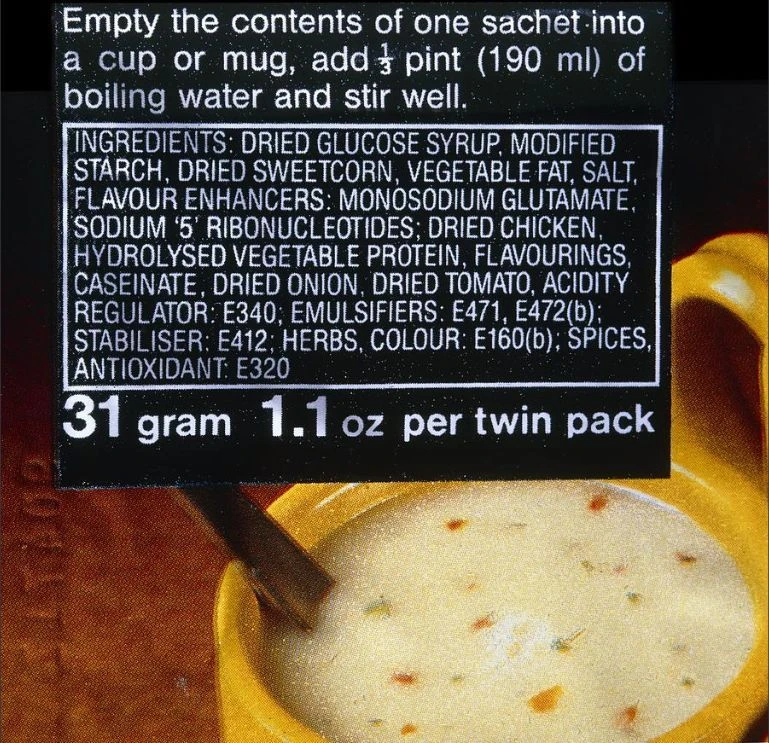
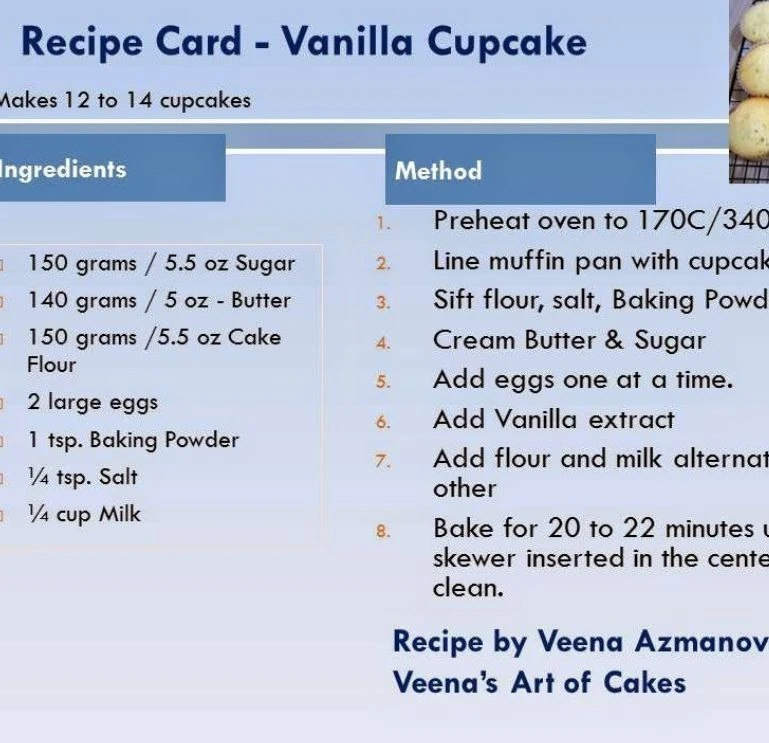
Data Collection Metrics
- Total Recipes Collect: 15,000 recipes.
- Ingredients Tagged: 75,000 ingredients identify and tag (averaging 5 per recipe).
- Instructions Annotate: 75,000 cooking instructions were annotate to ensure clarity and proper sequence (averaging 5 per recipe).
Annotation Process
Stages
- Ingredient Identification: Annotators, including culinary experts, meticulously tagged each ingredient within the recipes to ensure precise identification.
- Instruction Sequencing: Cooking steps were annotated to maintain the correct sequence, aiding in accurate recipe interpretation and execution by LLMs.
Annotation Metrics
- Team Involvement: A team of 30 annotators, including culinary experts, work over a span of 2 months to complete the project.
- Total Annotations: 150,000 annotations were made, encompassing both ingredients and cooking instructions.
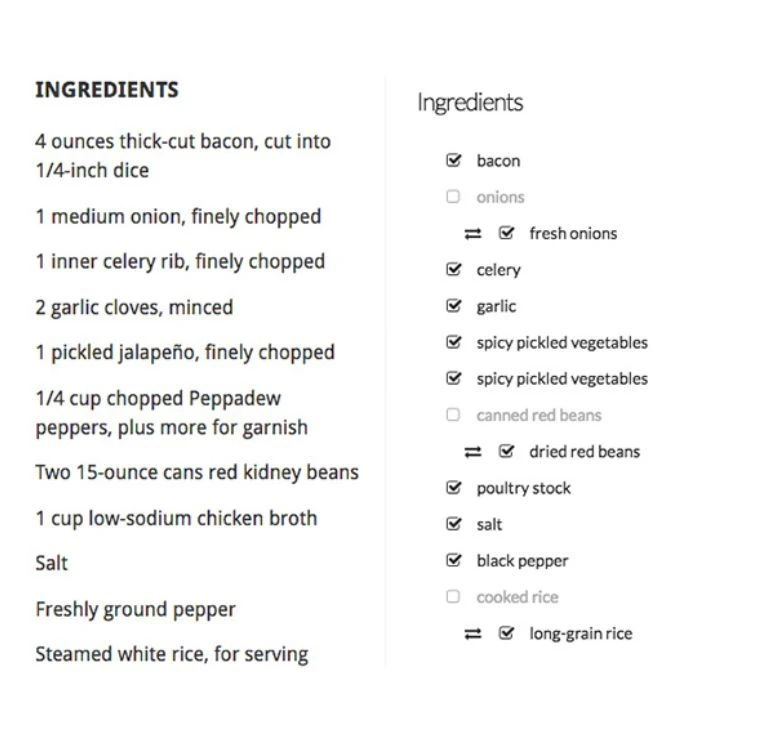
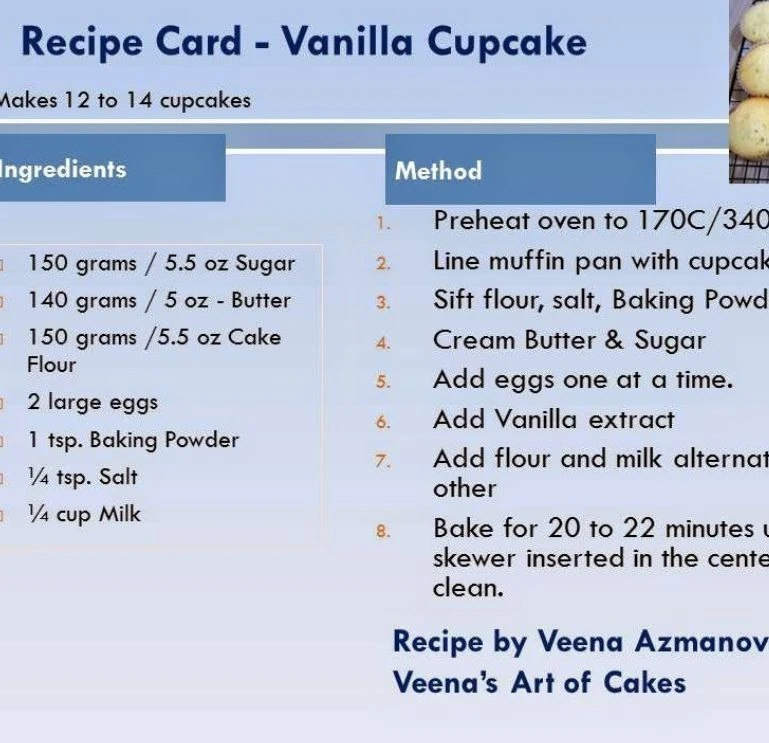
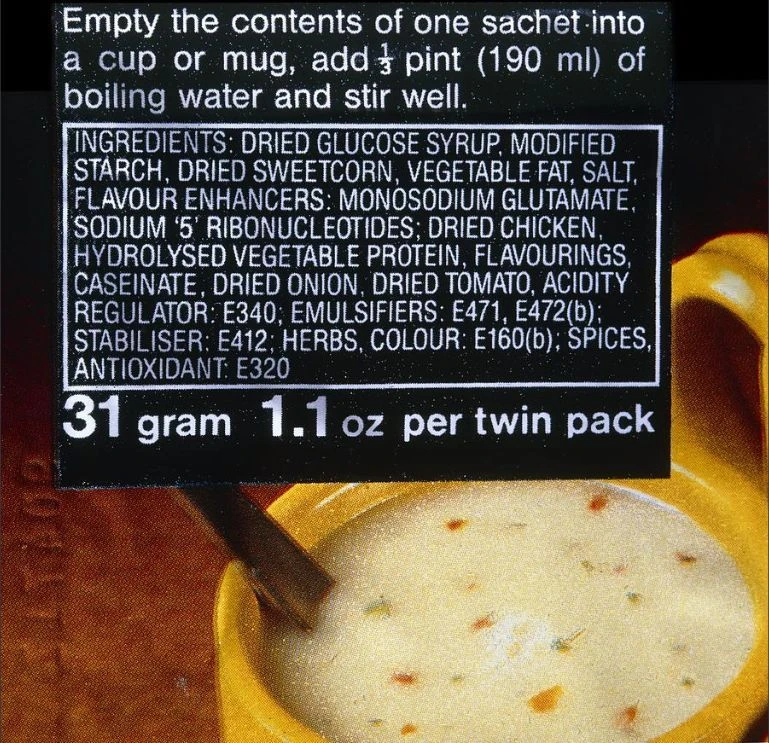
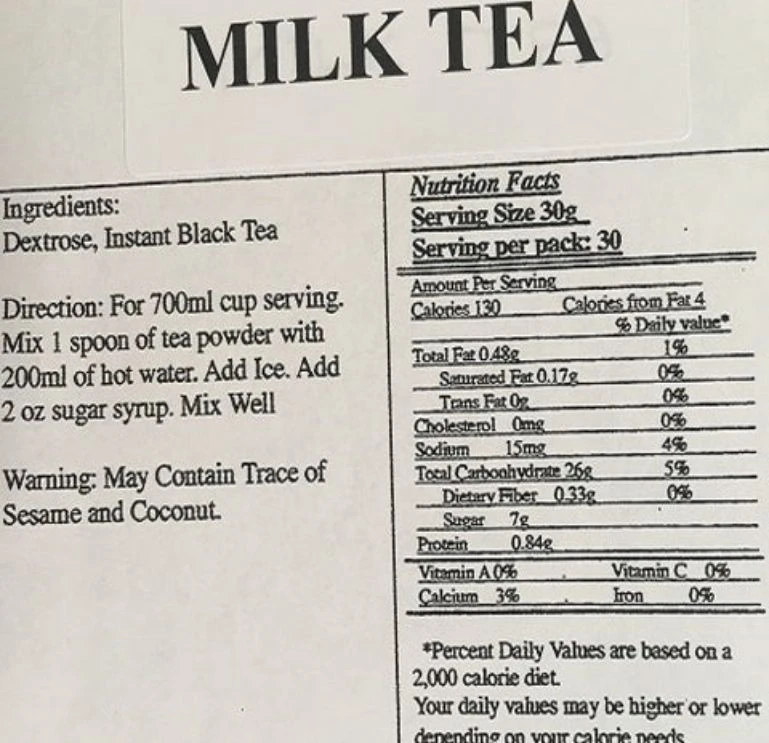
Quality Assurance
Stages
- Annotation Accuracy: Rigorous checks were implement to ensure that ingredients and instructions were correctly annotate and that the sequence of steps was logical and consistent.
- Culinary Expertise: Involvement of culinary experts ensure that the annotations were not only accurate but also contextually appropriate for various cuisines.
QA Metrics
- Ingredient Identification Accuracy: High accuracy was achieved in correctly tagging ingredients across all recipes.
- Instruction Sequencing Accuracy: The cooking steps were accurately annotate and sequenced, contributing to the dataset’s overall quality and utility.
Conclusion
The creation of this recipe annotation dataset significantly improve the capabilities of LLMs in understanding and processing culinary information. The dataset has proven to be a valuable resource in the development of AI-driven cooking assistants and tools for dietary analysis, enhancing the user experience in the food and beverage industry.

Quality Data Creation

Guaranteed TAT

ISO 9001:2015, ISO/IEC 27001:2013 Certified

HIPAA Compliance

GDPR Compliance

Compliance and Security
Let's Discuss your Data collection Requirement With Us
To get a detailed estimation of requirements please reach us.