Pupils Segmentation Dataset
Home » Case Study » Pupils Segmentation Dataset
Project Overview:
Objective
Scope
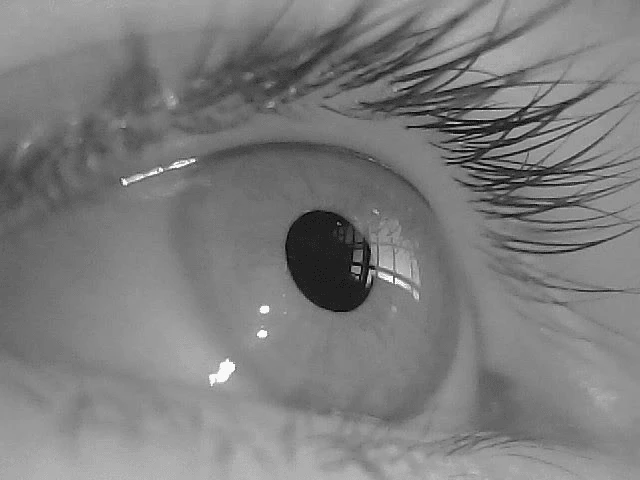
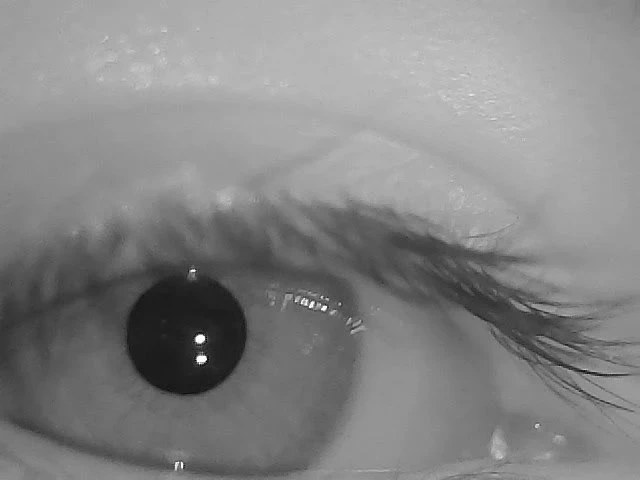
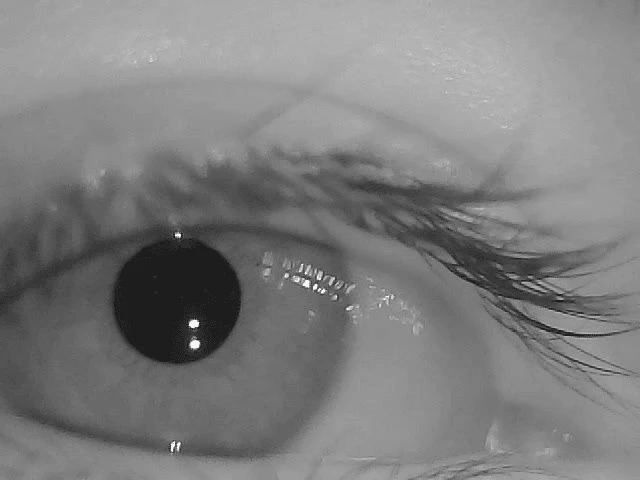
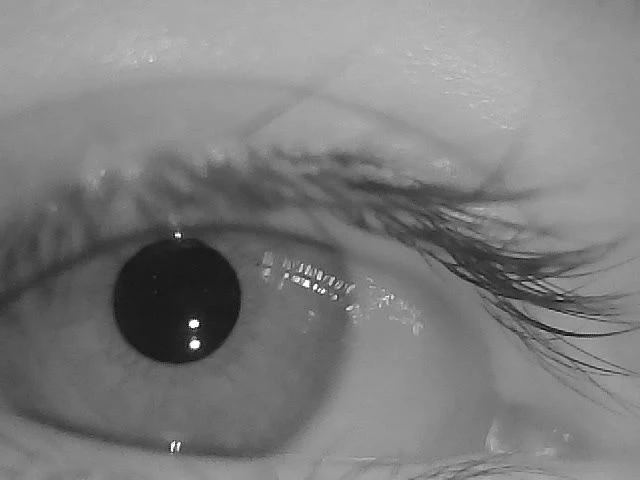
Sources
- Collaborations with ophthalmology clinics and hospitals.
- Additionally, dataset augmentation from publicly available eye databases will be essential.
- Moreover, contributions from volunteers through a dedicated app will ensure diversity in lighting and angles.
- Furthermore, special sessions for capturing images of individuals with ocular conditions will be arranged.
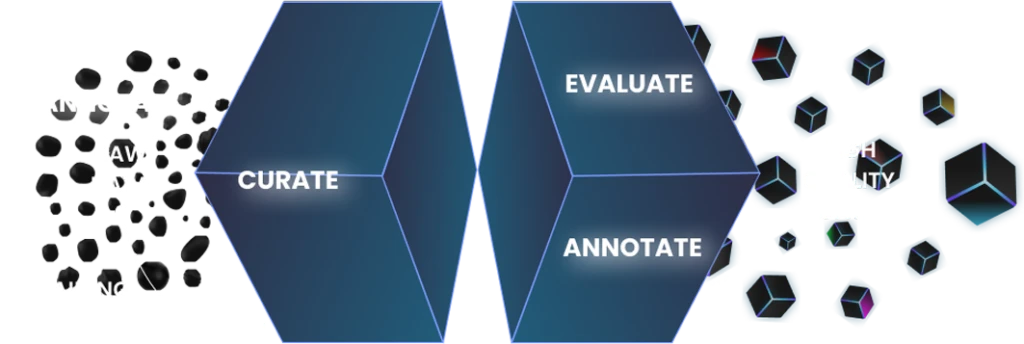
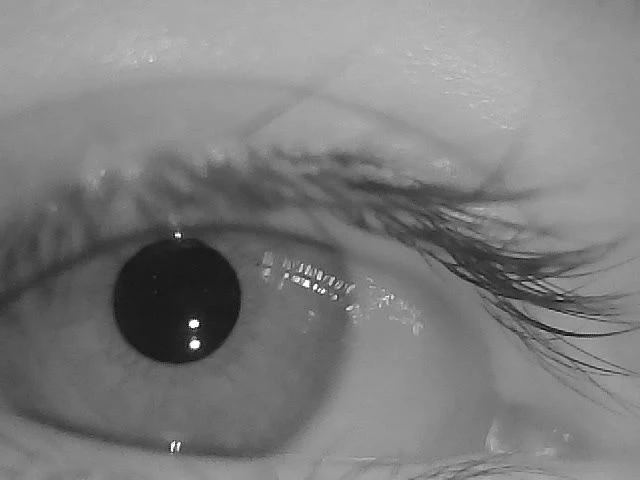
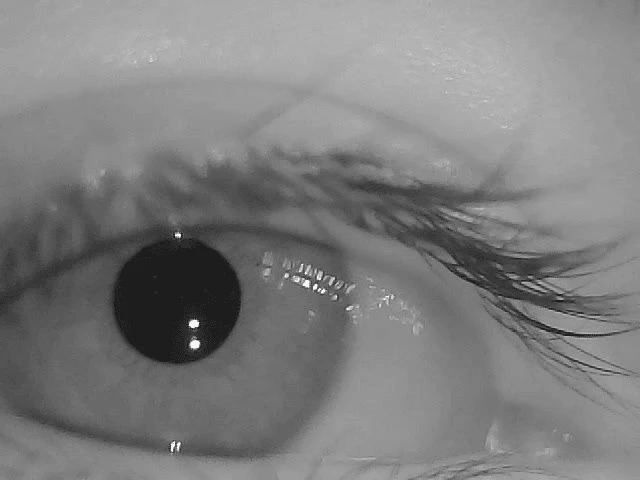
Data Collection Metrics
- Total Eye Images: 100,000
- Natural Lighting: 30,000
- Artificial Lighting: 20,000
- Mixed Lighting: 25,000
- Ocular Conditions: 15,000
- Varied Ethnicities: Covered across all categories
Annotation Process
Stages
- Image Pre-processing: Involves sharpening, contrast enhancement, and normalization for optimal clarity.
- Pixel-wise Segmentation: Where annotators use specialized tools to mark every pixel belonging to the pupil region.
- Validation: Is conducted through cross-verification by a secondary annotator and preliminary segmentation models.Â
Annotation Metrics
- Firstly, the total pixel-wise annotations amount to 100,000, with one for each image.
- Secondly, the average annotation time per image stands at 10 minutes, owing to the intricate nature of the task.
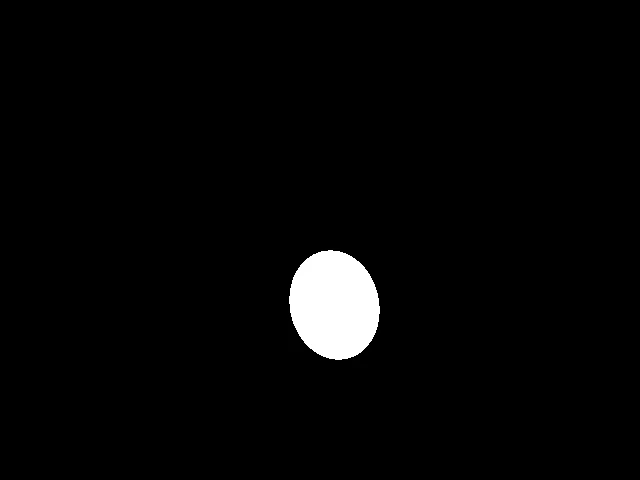
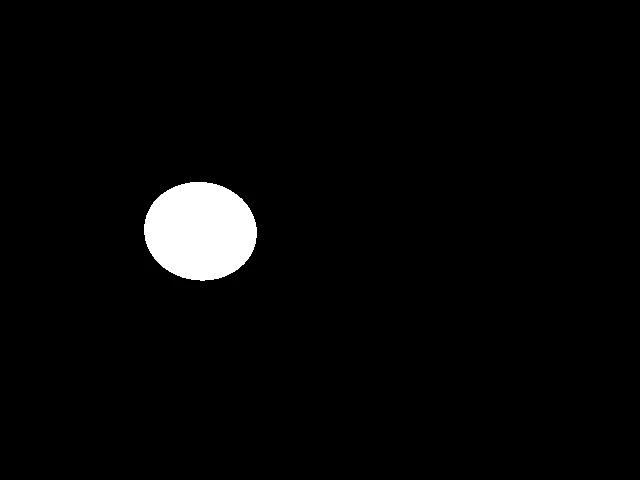
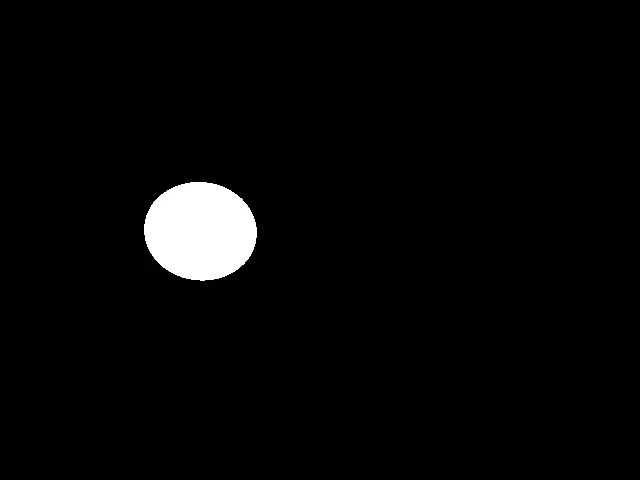
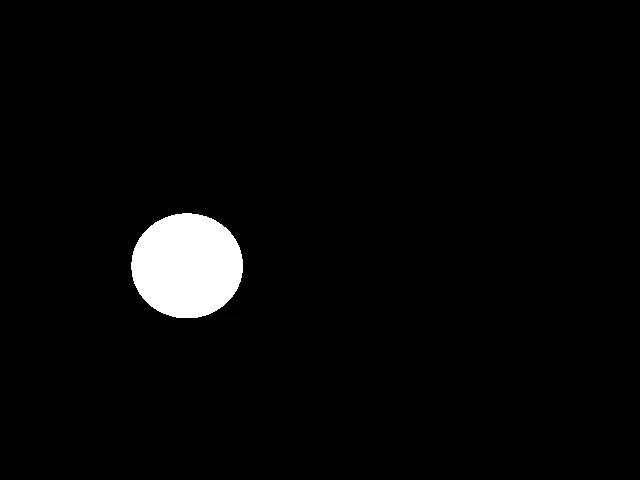
Quality Assurance
Stages
Automated Model Validation: In the initial phase, segmentation models evaluate the quality of annotations by comparing them to their own segmentations.
Peer Review: Furthermore, each annotation is cross-verified by another experienced annotator to check for discrepancies.
Inter-annotator Agreement: Furthermore, randomly selected images are annotated by multiple individuals to ensure consistent labeling quality and maintain a standard.
QA Metrics
- Firstly, Annotations Validated using Segmentation Models reached 50,000, accounting for 50% of total images.
- Additionally, Peer Reviewed Annotations amounted to 30,000, representing 30% of total images.
- Moreover, Inconsistencies Identified and Rectified numbered 3,000, making up 3% of total images.
Conclusion
The Pupils Segmentation Dataset represents a groundbreaking initiative aimed at equipping computer vision researchers with a comprehensive toolset for capturing the nuanced characteristics of the human pupil. With its diversity and precision, this dataset stands poised to catalyze substantial progress in the realms of gaze detection, health diagnostics, and biometric systems. Consequently, it guarantees both precise and efficient pupil segmentation, heralding a new era of advancement in these critical fields.

Quality Data Creation

Guaranteed TAT

ISO 9001:2015, ISO/IEC 27001:2013 Certified

HIPAA Compliance

GDPR Compliance

Compliance and Security
Let's Discuss your Data collection Requirement With Us
To get a detailed estimation of requirements please reach us.