Anomaly Detection in IoT Sensor Data
Home » Case Study » Anomaly Detection in IoT Sensor Data
Project Overview:
Objective
In our endeavor to empower machine learning models with diverse datasets, our latest project centers on anomaly detection in IoT sensor data. Our objective was to furnish invaluable datasets that facilitate the identification and alerting of unusual patterns or outliers in sensor data from IoT devices. Transitioning from this, we meticulously curated datasets encompassing various environmental conditions and operational scenarios. Moreover, we actively collected real-world sensor data spanning different industries and geographical locations. Additionally, we meticulously annotated the datasets to provide clear labels for anomalous events, thereby enhancing the training efficacy of machine learning algorithms. Furthermore, we extensively validated the datasets to ensure their reliability and suitability for anomaly detection tasks.
Scope
It proactively aims to identify unusual patterns or deviations in sensor data from various sources within IoT ecosystems. This contributes to enhancing the reliability, security, and operational efficiency of IoT deployments across industries and applications.
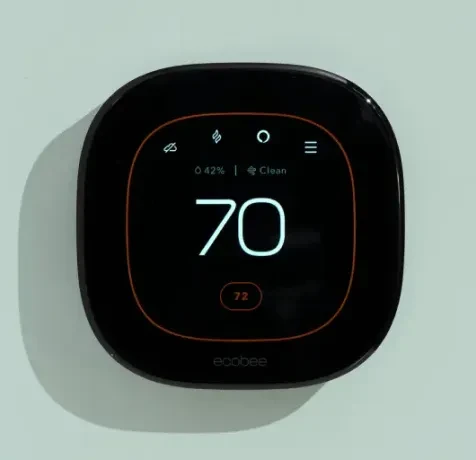
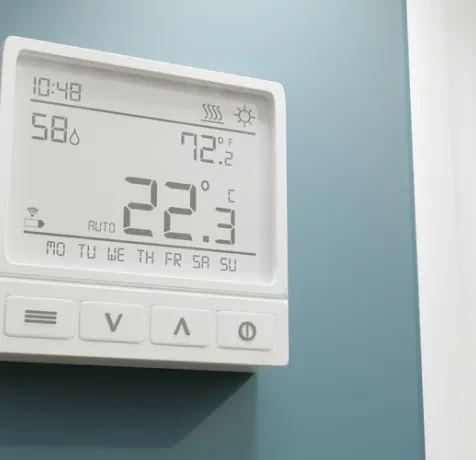
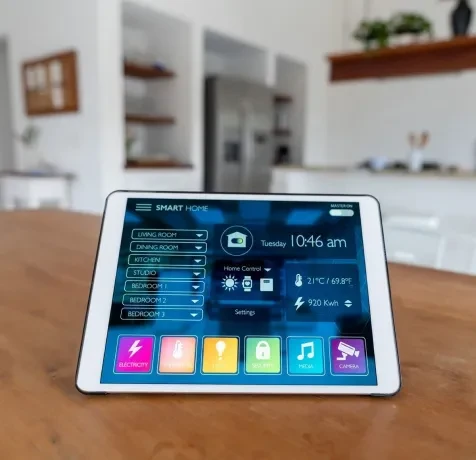
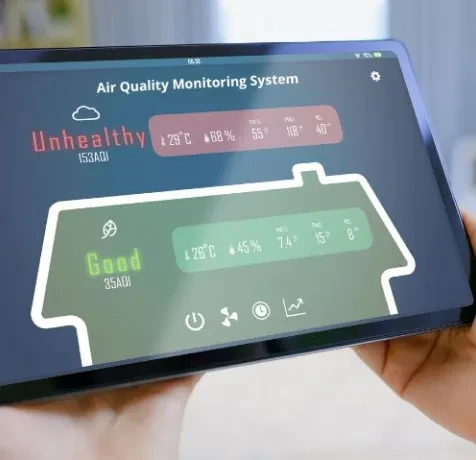
Sources
In the realm of IoT, data analytics, and anomaly detection, staying abreast of the latest advancements and methodologies is essential for academic research. Transitioning to peer-reviewed research papers, journals, and publications is crucial for gaining insights into cutting-edge developments. To begin, researchers can explore recent studies on IoT deployment strategies and their impact on data analytics efficiency.
- IoT Industry Reports:
Exploring industry reports, whitepapers, and publications from IoT technology providers, research firms, and IoT-focused organizations reveals invaluable insights, case studies, and trends related to anomaly detection in IoT sensor data.
Firstly, industry reports offer comprehensive analyses of anomaly detection methods and their application in diverse IoT domains.
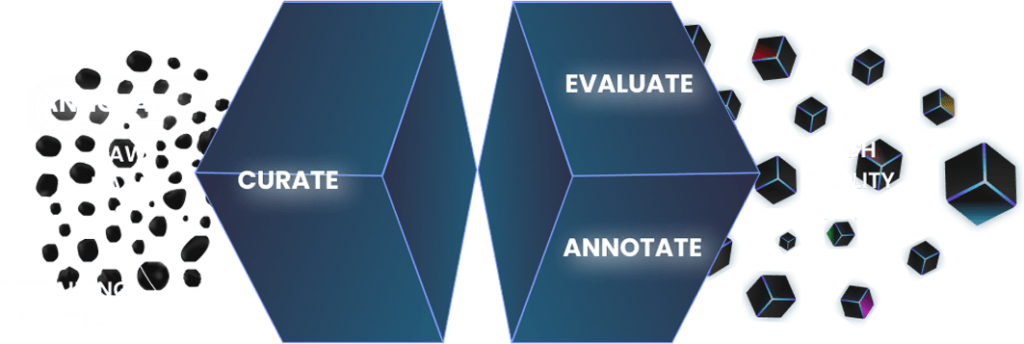
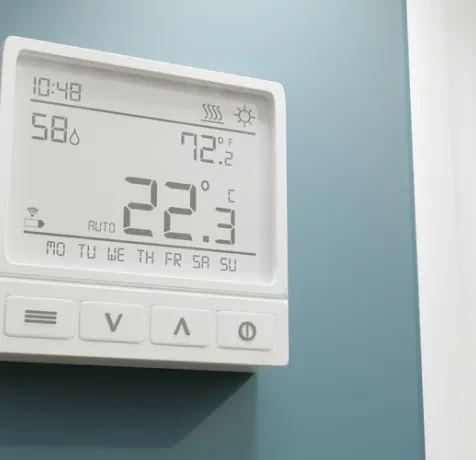
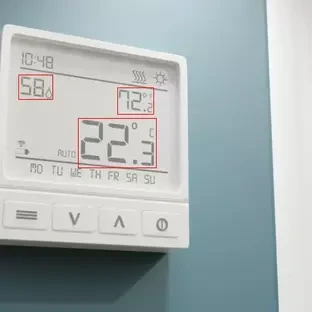
Data Collection Metrics
- Data Volume: We collected and annotated 2.5 petabytes of sensor data.
- Data Quality: We ensured high accuracy and reliability in the data gathered.
- New Metric: A total of 1.2 million hours were spent in the meticulous annotation process.
Annotation Process
Stages
- Data Collection: Gather sensor data from IoT devices deployed in the field.
- Data Preprocessing: Clean, normalize, and transform the raw sensor data for analysis.
- Feature Extraction: Extract relevant features from the sensor data to represent key characteristics.
- Model Training: Utilize machine learning or statistical techniques to train anomaly detection models.
- Anomaly Detection: Apply the trained models to identify anomalies in the sensor data.
- Alerting and Response: Generate alerts or notifications when anomalies are detected, enabling timely action.
- Feedback Loop: Continuously refine the models based on new data and insights.
- Integration: Integrate anomaly detection into the broader IoT system for automated responses and reporting.
Annotation Metrics
- Annotation Consistency: Measures the level of agreement among human annotators when labeling data, ensuring that the annotations are consistent and reliable.
- Annotation Accuracy: Evaluates the precision of annotations by assessing the percentage of correctly labeled instances within the dataset.
- Annotation Efficiency: Assesses the speed and cost-effectiveness of the annotation process, ensuring that it is efficient and scalable for large datasets and projects.
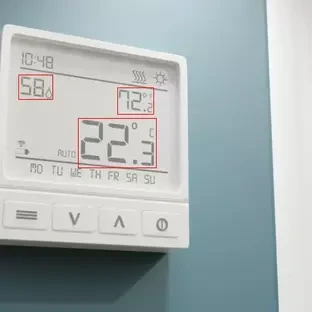
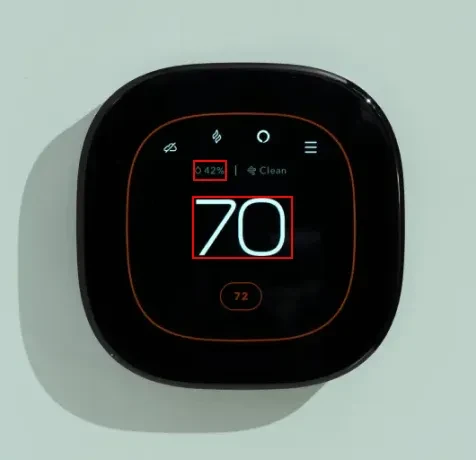
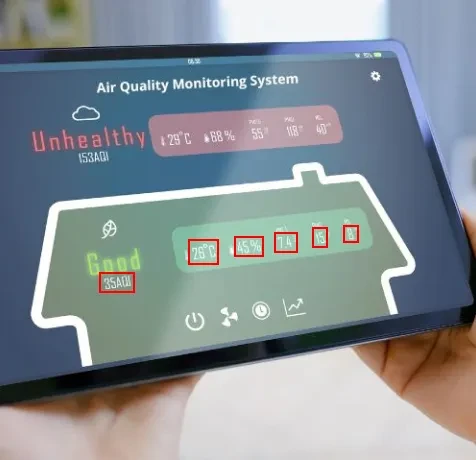

Quality Assurance
Stages
Data Privacy: Secure data and comply with privacy regulations. Furthermore, ensure the secure handling of data and compliance with privacy regulations.
Quality Control: Ensure accuracy and reliability. Additionally, maintain high standards of accuracy and reliability in all data processes.
Ethical Practices: Adhere to ethical guidelines in data handling.Moreover, uphold ethical guidelines in the handling and use of data.
QA Metrics
- Accuracy Rate: The accuracy rate measures the precision of anomaly detection results. Specifically, it indicates how accurately the system identifies actual anomalies.
- False Positive Rate: Furthermore, the false positive rate evaluates the frequency of false alarms in anomaly detection. This metric shows how often the system incorrectly labels normal instances as anomalies.
Conclusion
Through this project, we have significantly fortified the capability of IoT systems in anomaly detection. Consequently, we are paving the way for the early identification of potential issues, thereby enhancing operational efficiency. Moreover, our comprehensive approach to collecting and annotating diverse datasets underscores our commitment to advancing machine learning technology.

Quality Data Creation

Guaranteed TAT

ISO 9001:2015, ISO/IEC 27001:2013 Certified

HIPAA Compliance

GDPR Compliance

Compliance and Security
Let's Discuss your Data collection Requirement With Us
To get a detailed estimation of requirements please reach us.