Human Pose Estimation Dataset
Home » Case Study » Human Pose Estimation Dataset
Project Overview:
Objective
We wanted to create a Human Pose Estimation Dataset to enhance technology for analyzing human body movements. This dataset aims to improve the accuracy and speed of motion analysis apps. Additionally, by evaluating the precision and correctness of annotations, we can ensure that the dataset effectively identifies the type, location, and boundaries of each movement.
Scope
We worked hard to gather lots of data and mark it with details. We made sure to include many different kinds of movements from people of all ages and backgrounds. This makes the dataset strong and useful for real-life situations.
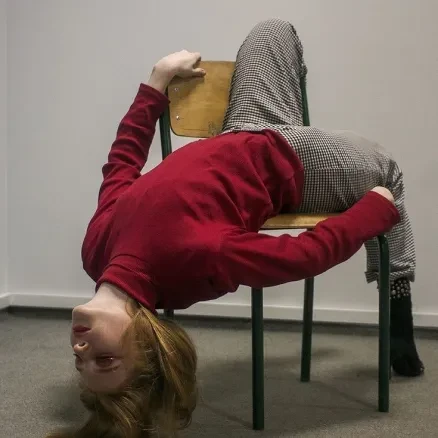
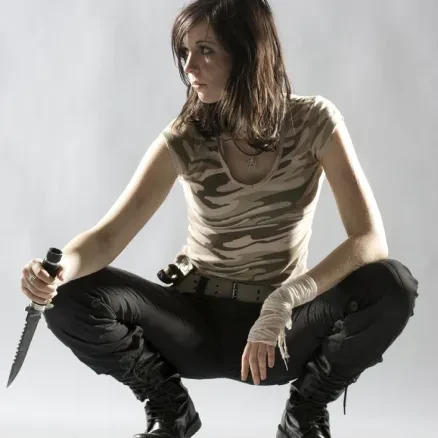
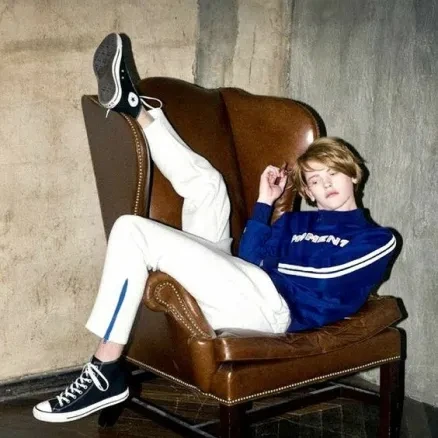
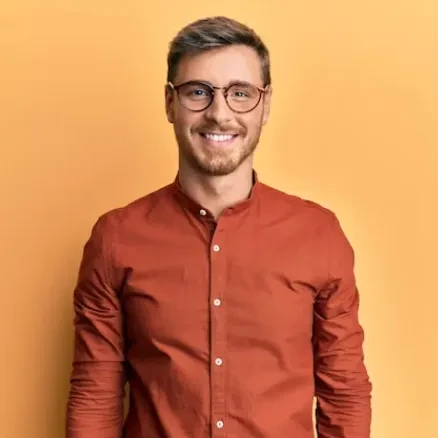
Sources
- Real Human Activities: We took pictures and videos of people doing regular things like walking, playing sports, and performing art.
- Staged Interactions: We also filmed planned situations to get poses that aren’t usually seen in public datasets.
- Enhancement with Existing Data: We included extra pose information from other public sources to make our dataset even more varied.
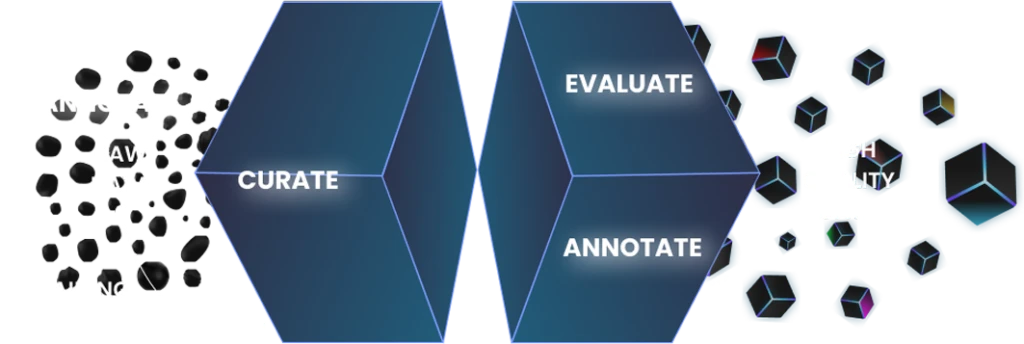
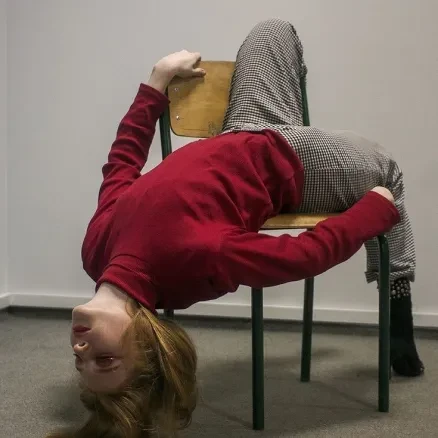
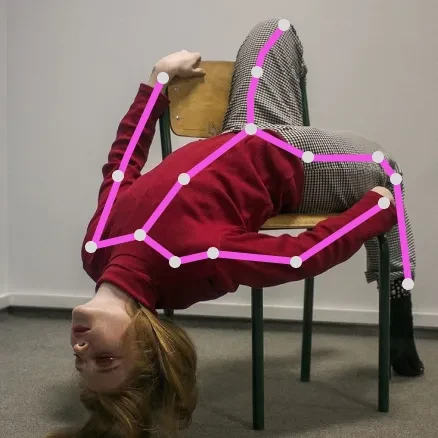
Data Collection Metrics
- Total Data Collected: We gathered 120,000 pictures and frames from videos showing different human poses.
- Data Annotated for ML Training: We marked 180,000 instances of poses to teach computer programs.
Annotation Process
Stages
- Key Points Annotation: We marked important parts of the body like joints, hands, feet, and facial features.
- Pose Categorization: We sorted each pose based on the activity being done.
- Demographic Information: We also added details about the people’s characteristics to make the dataset useful for different purposes.
Annotation Metrics
- Annotated Poses: 180,000
- Pose Categories: 100+ unique activity types.
- Demographic Tags: Included for all data points.
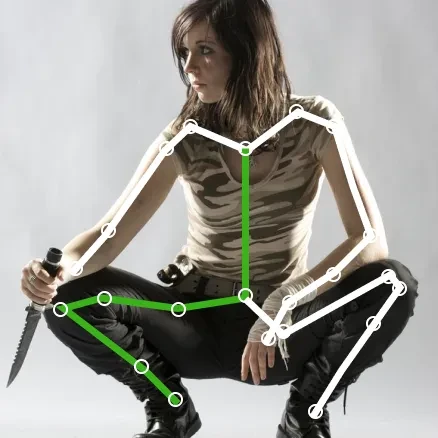
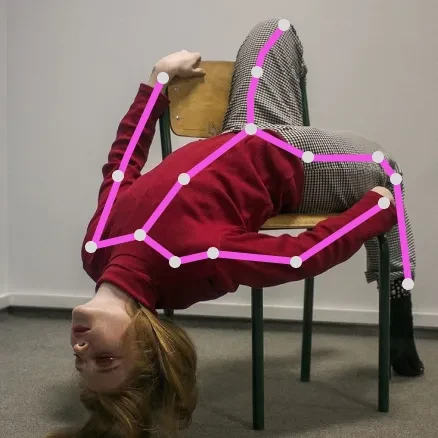
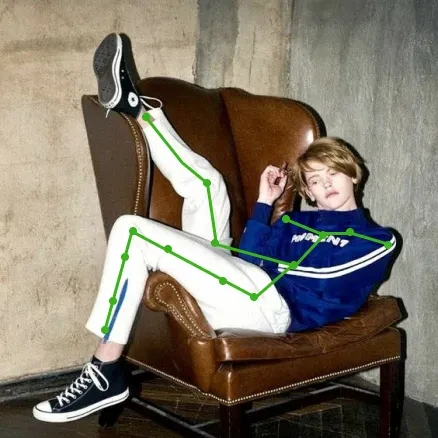
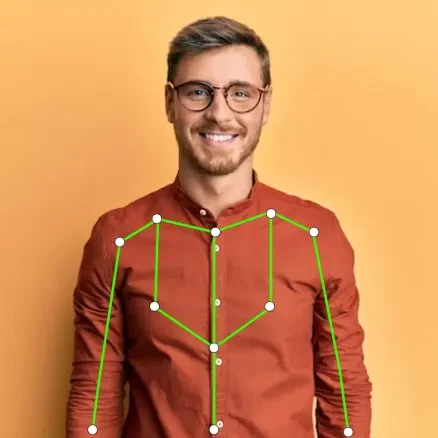
Quality Assurance
Stages
Model Validation: We kept checking how good our pose estimation models were with new data to make sure they worked well.
Privacy Compliance: We followed strict privacy rules during data collection, making sure everyone agreed to their data being used.
Iterative Improvement: We used feedback from early model tests to improve how we gathered and marked the data.
QA Metrics
- Pose Recognition Accuracy: 92%
- Coverage Accuracy: 95%
- Privacy Compliance: 100%
Conclusion
The Human Pose Estimation Dataset – COCO has really improved how computers recognize and understand different human movements. It’s very accurate and helps developers and researchers make AI systems that can respond better to what people are doing.

Quality Data Creation

Guaranteed TAT

ISO 9001:2015, ISO/IEC 27001:2013 Certified

HIPAA Compliance

GDPR Compliance

Compliance and Security
Let's Discuss your Data collection Requirement With Us
To get a detailed estimation of requirements please reach us.