Semantic Annotation
Home » Case Study » Semantic Annotation
Project Overview:
Objective
The Semantic Annotation project, tailored for Semantic Annotation Services, is designed to create a comprehensive dataset for training advanced AI models in understanding and interpreting textual data. This dataset will play a pivotal role in various applications, including natural language processing, content categorization, and sentiment analysis.
Scope
This project encompasses the collection of diverse textual data from multiple sources and its annotation with semantic labels, such as entities, context, sentiment, and other relevant linguistic features. These annotations are integral to Semantic Annotation Services, enhancing the understanding and utility of the collected data.
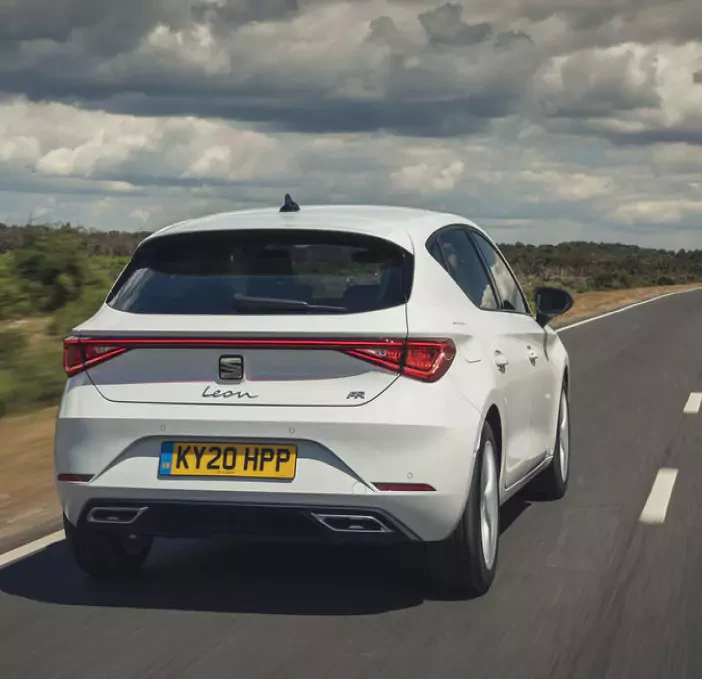
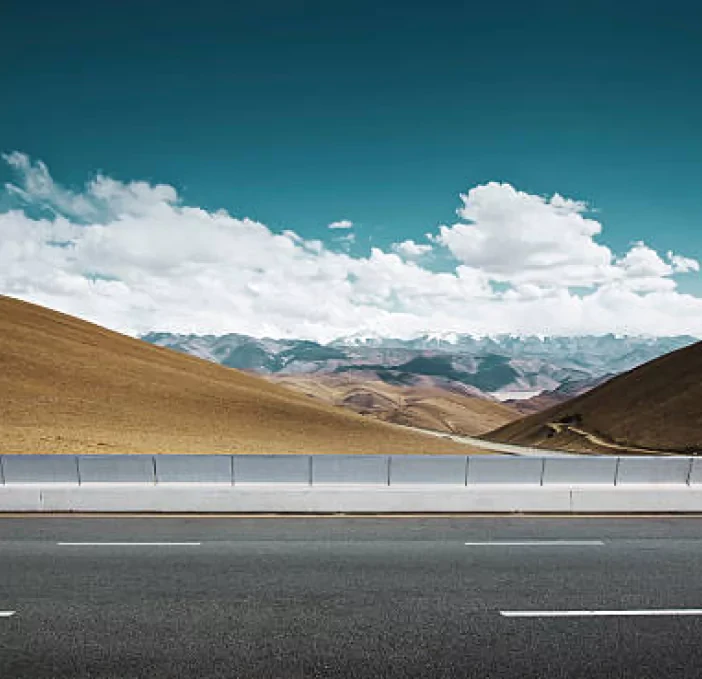
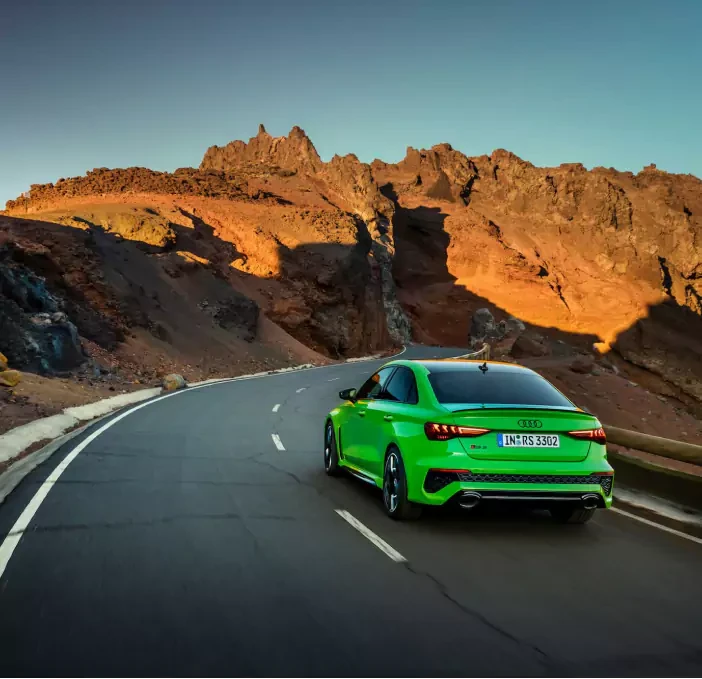
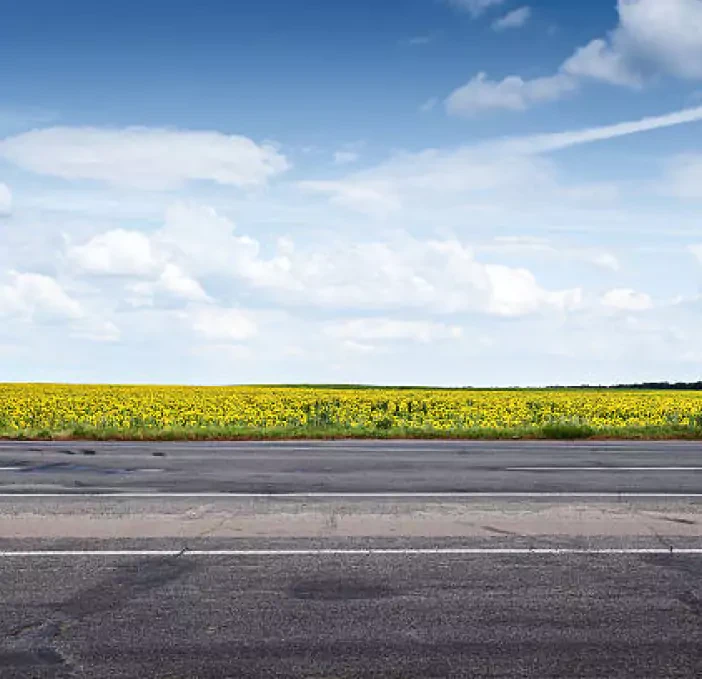
Sources
- Web Scraping: Harvesting data from various websites for a wide range of topics.
- Collaborations: Partnering with educational and research institutions for scholarly articles and papers.
- Public Contributions: Encouraging public submissions of text content for diverse linguistic patterns.
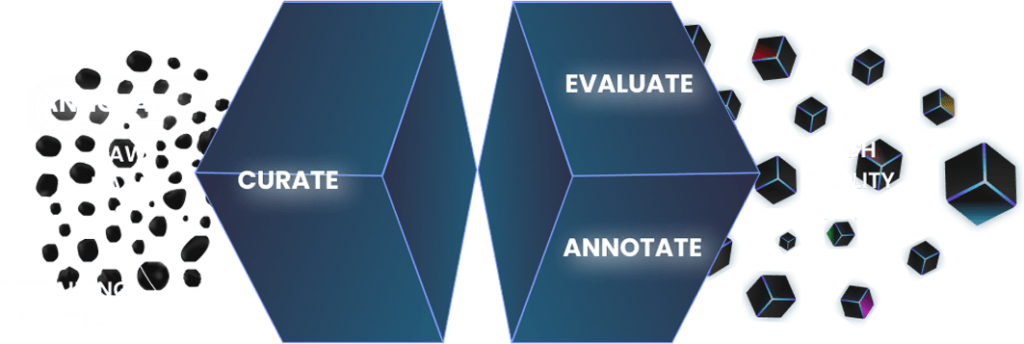
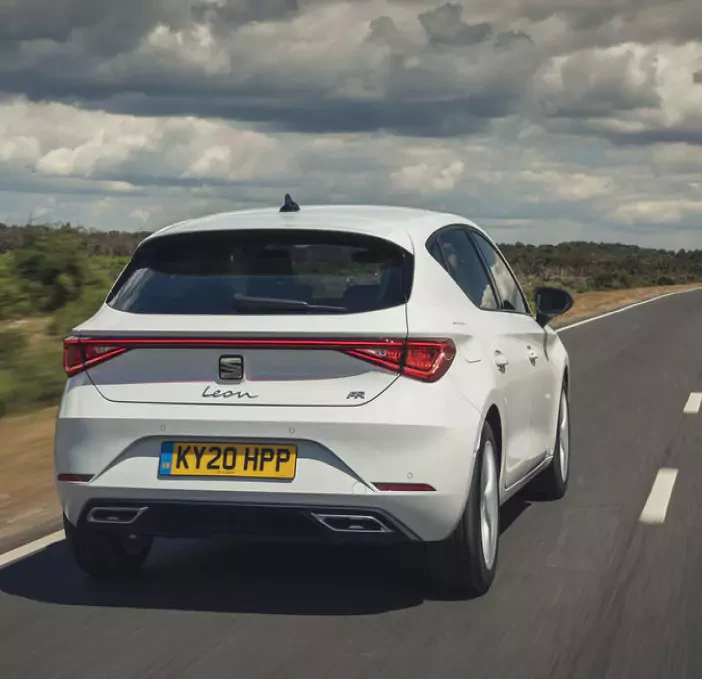
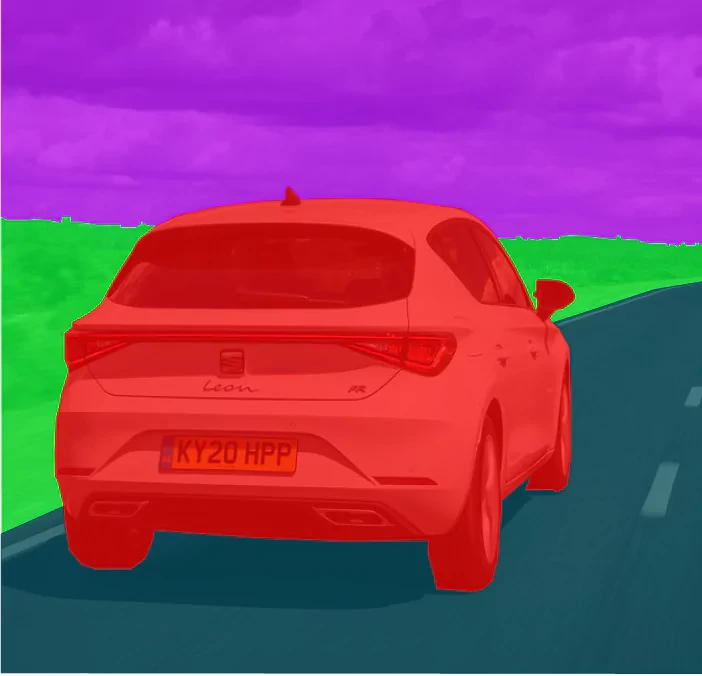
Data Collection Metrics
- Total Text Entries Collected: 50,000
- Web Scraping: 30,000 entries
- Collaborations with Institutions: 10,000 entries
- Public Contributions: 10,000 entries
Annotation Process
Stages
- Semantic Labeling: Each text entry is annotated with semantic labels identifying entities, sentiments, and contextual meanings.
- Metadata Documentation: Capturing metadata such as the source, author, and date of each text entry for comprehensive understanding and traceability.
Annotation Metrics
- Text Entries with Semantic Labels: 50,000
- Metadata Documentation: 50,000 entries
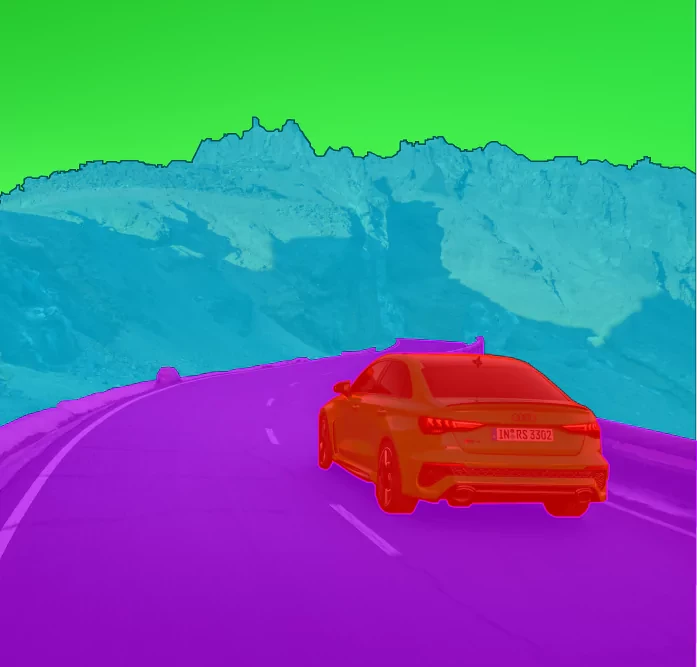
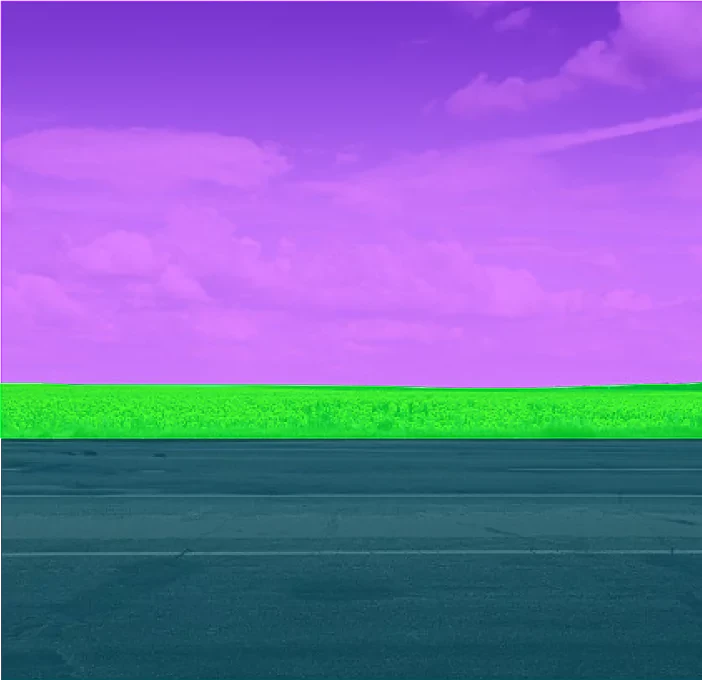
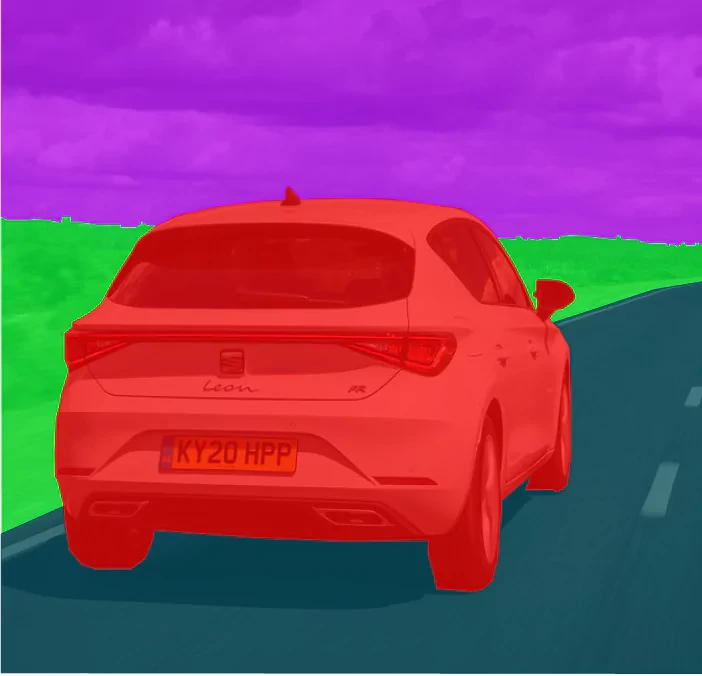
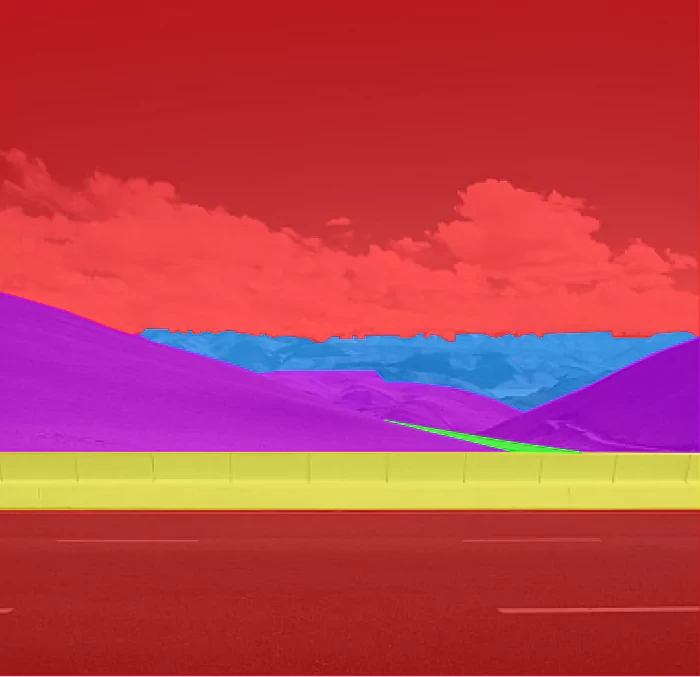
Quality Assurance
Stages
Annotation Verification:Â Implementing rigorous validation processes to ensure the accuracy of semantic labels.
Data Quality Control: Removing irrelevant or low-quality text entries.
Data Security:Â Ensuring the privacy and security of the collected data, adhering to data protection regulations.
QA Metrics
• Annotation Validation Cases: 5,000 (10% of total)
• Data Cleansing: Systematic removal of irrelevant or low-quality entries
Conclusion
The Semantic Annotation project represents a significant stride in the realm of data understanding and AI training. By meticulously collecting and annotating a diverse range of text entries, this dataset stands as a cornerstone for developing more nuanced and sophisticated AI models capable of interpreting complex linguistic constructs. This project not only enhances the capabilities of AI in language processing but also contributes to the broader field of machine learning by providing a rich, well-annotated dataset.

Quality Data Creation

Guaranteed TAT

ISO 9001:2015, ISO/IEC 27001:2013 Certified

HIPAA Compliance

GDPR Compliance

Compliance and Security
Let's Discuss your Data collection Requirement With Us
To get a detailed estimation of requirements please reach us.