Emotion Detection in Customer Feedback
Home » Case Study » Emotion Detection in Customer Feedback
Project Overview:
Objective
The “Emotion Detection in Customer Feedback” project aims to create a dataset for training machine learning models to automatically detect and classify emotions expressed in customer feedback, reviews, and comments. This dataset will enable businesses to gain valuable insights into customer sentiment and improve their products and services accordingly.
Scope
This project involves collecting customer feedback data from various sources, including online reviews, surveys, and social media comments, and annotating them with relevant emotional categories.
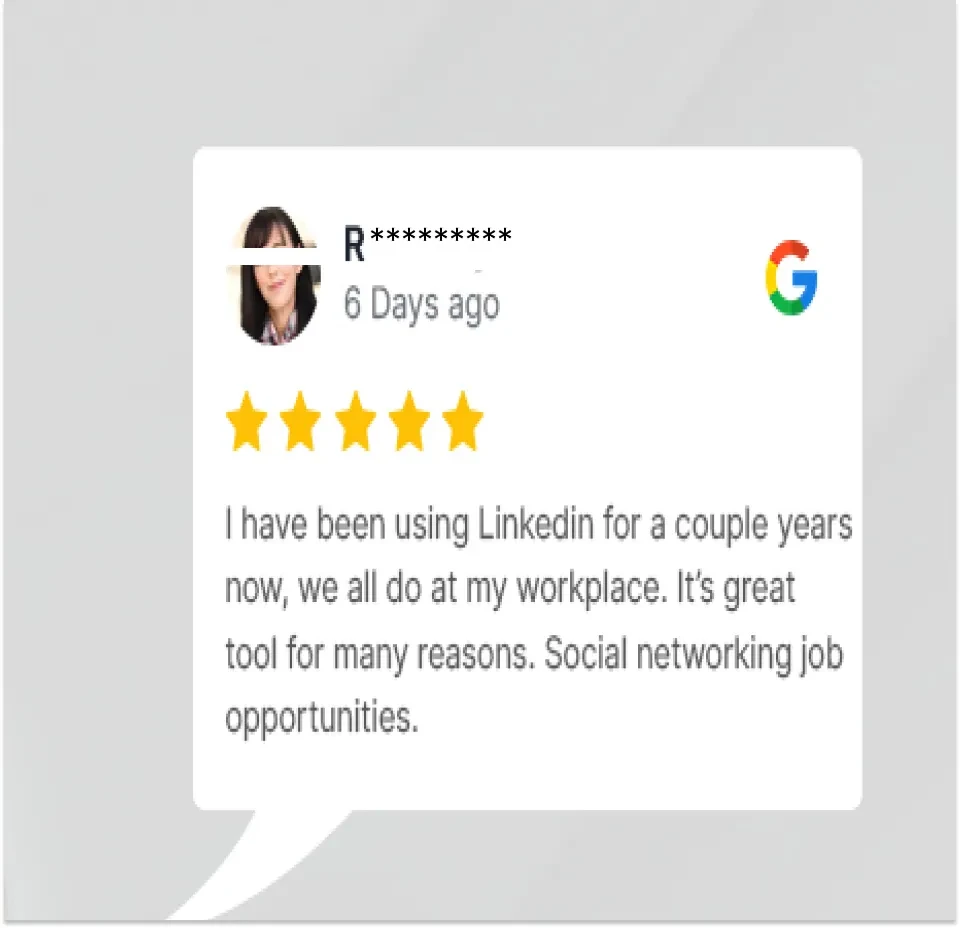
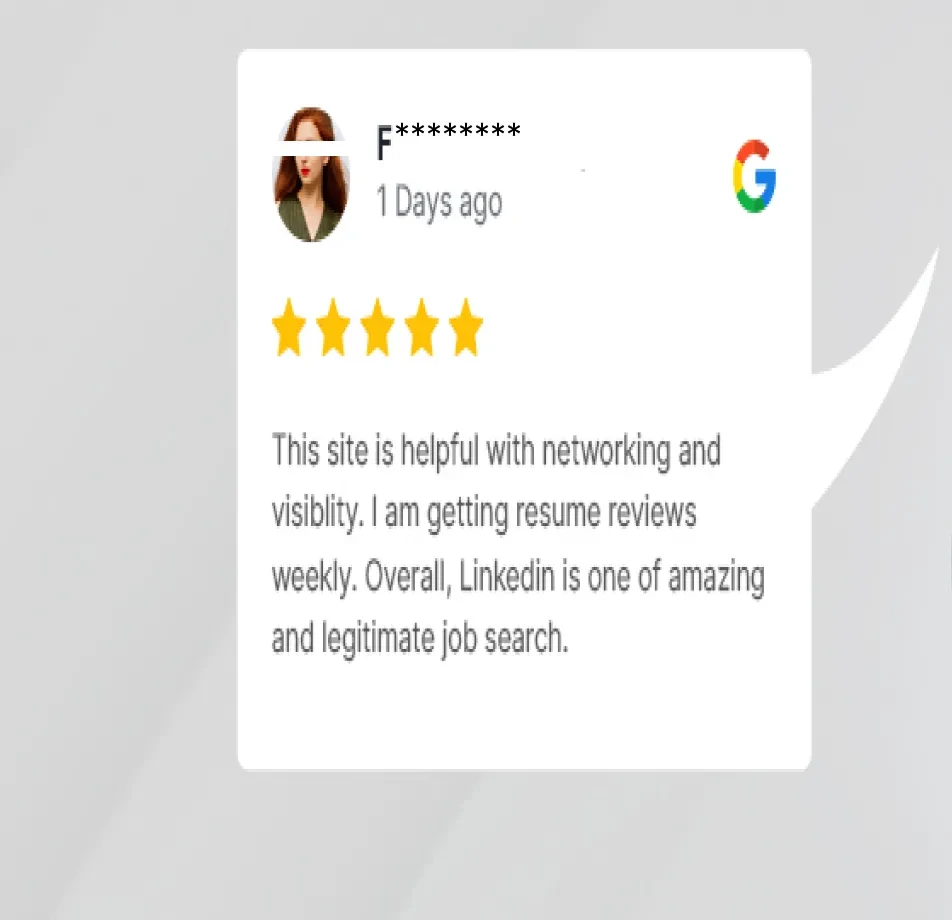
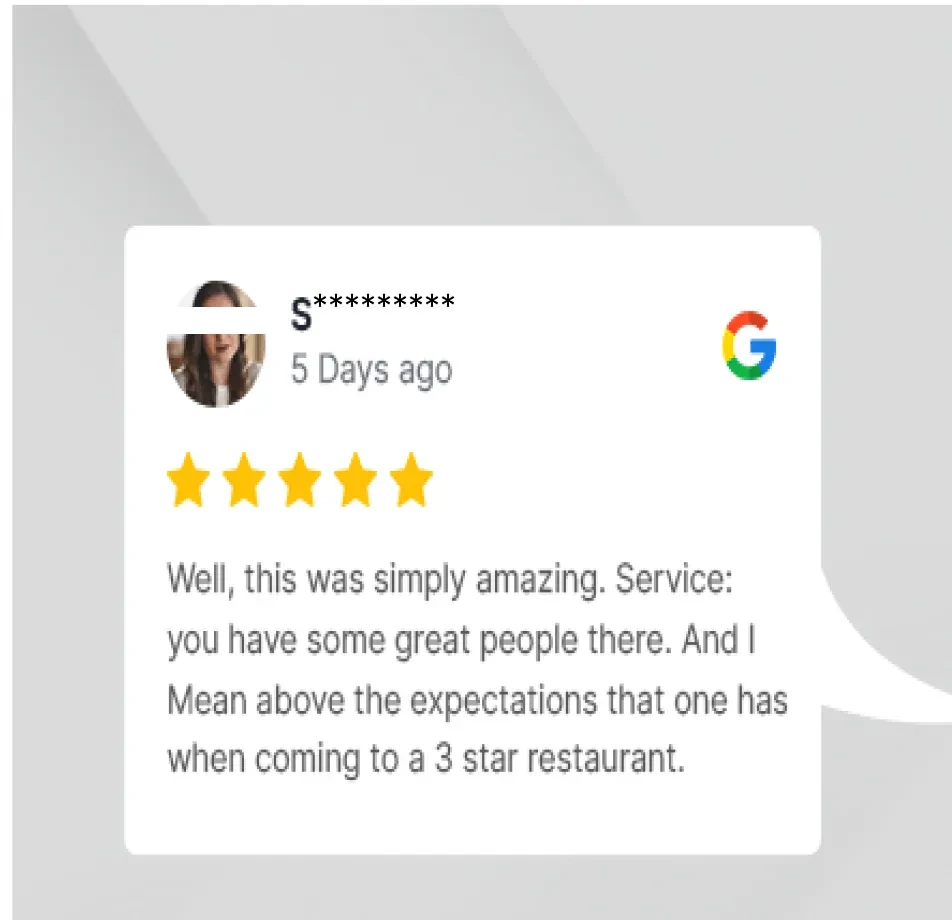
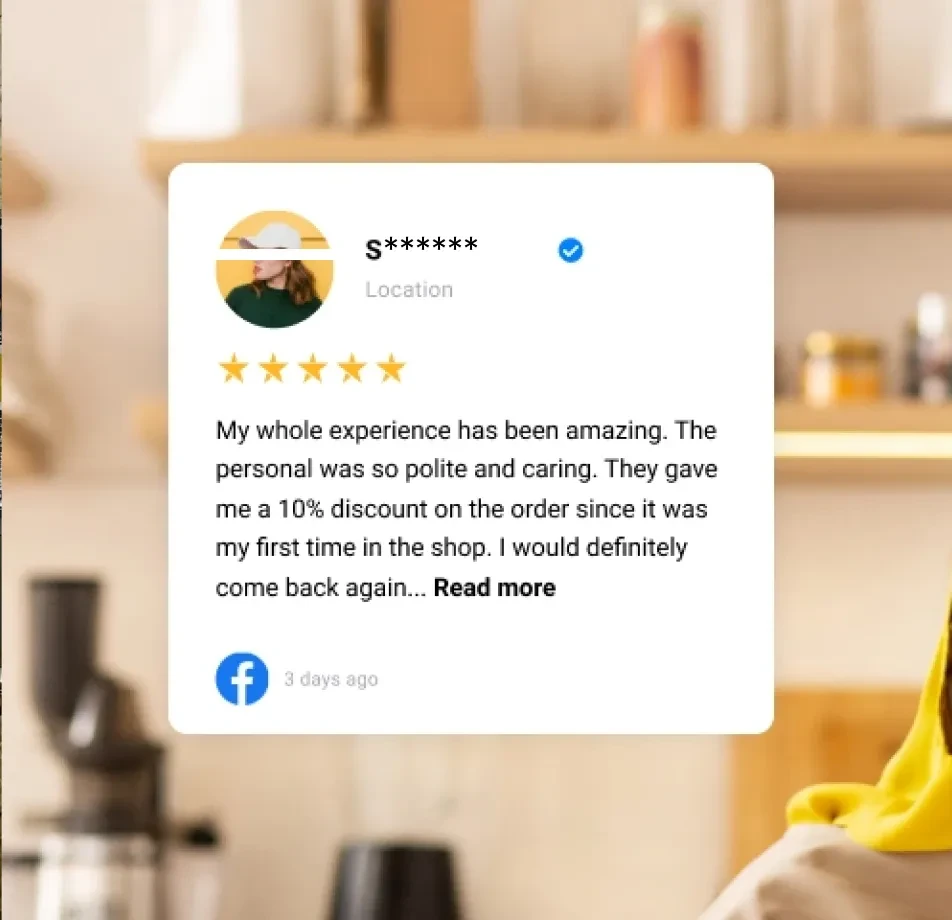
Sources
- Online Reviews: Gather customer reviews from e-commerce websites, online marketplaces, and product review platforms.
- Surveys: Collect feedback data from customer satisfaction surveys conducted by businesses.
- Social Media Comments: Access publicly available comments and mentions on social media platforms.
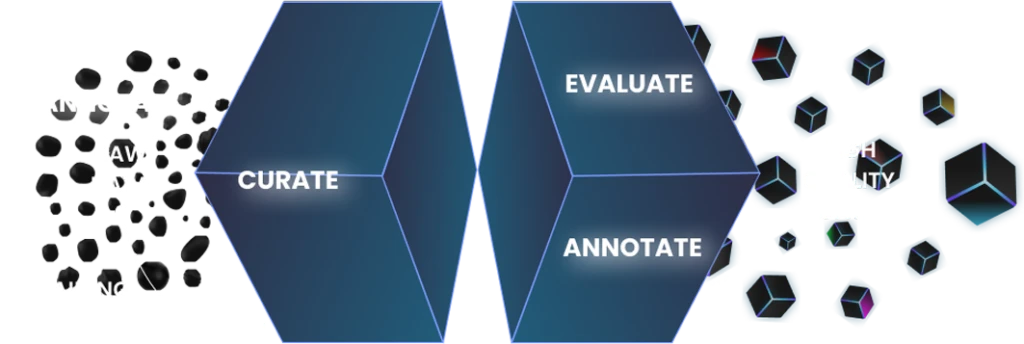
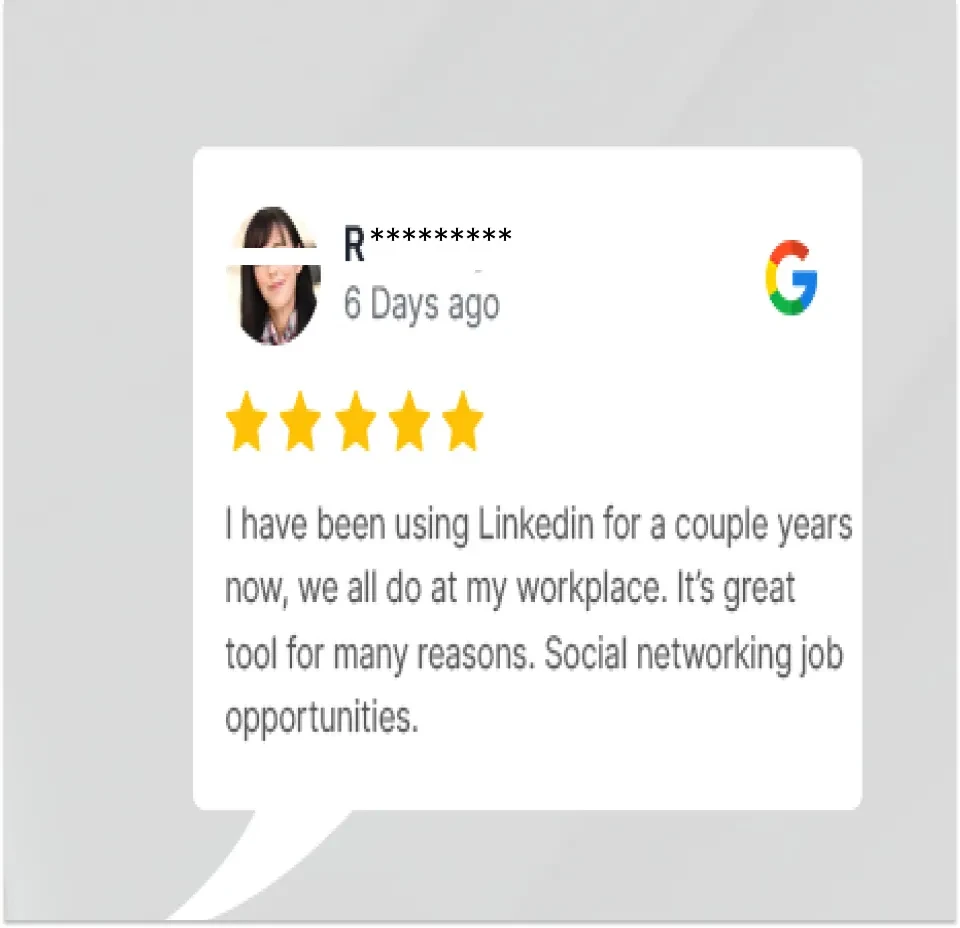
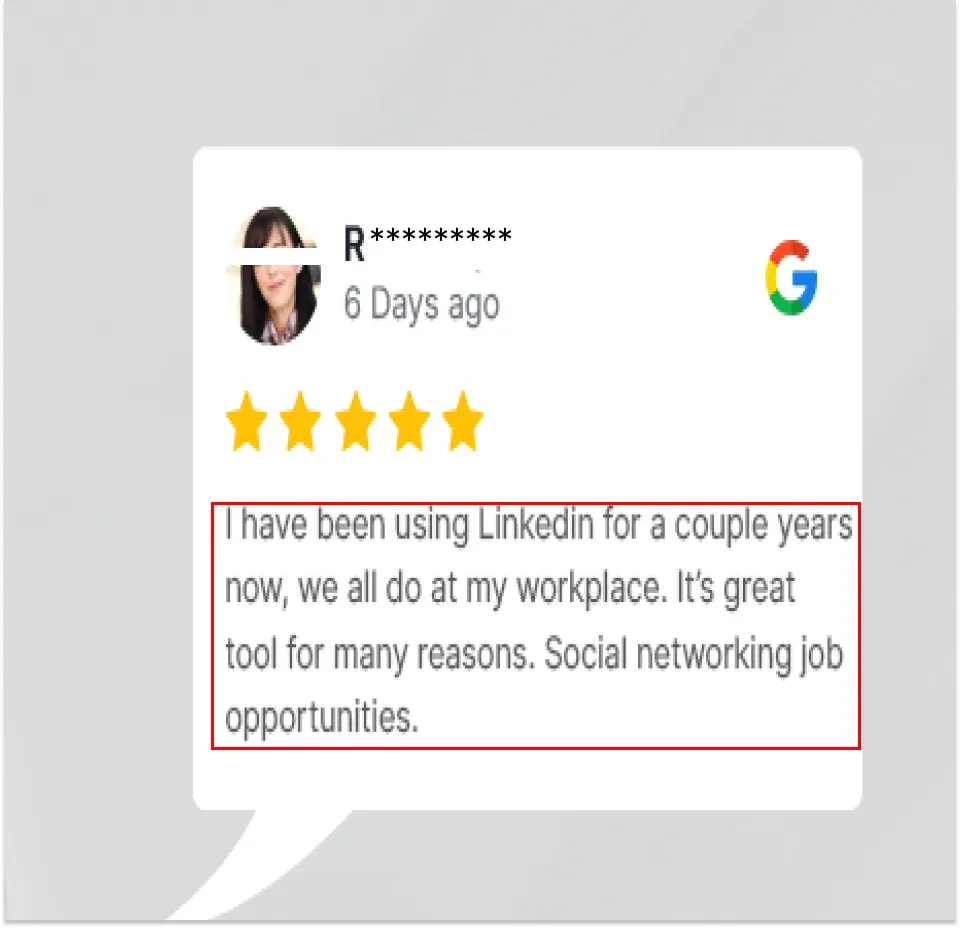
Data Collection Metrics
- Total Customer Feedback Entries: 25,000 entries
- Online Reviews: 15,000
- Surveys: 5,000
- Social Media Comments: 5,000
Annotation Process
Stages
- Emotion Detection: Annotate each customer feedback entry with labels indicating the emotion expressed, such as “Happy,” “Angry,” “Sad,” “Neutral,” etc.
- Metadata Logging: Log metadata, including the source of the feedback, date, product or service mentioned, and any relevant customer demographics.
Annotation Metrics
- Customer Feedback Entries with Emotion Labels: 25,000
- Metadata Logging: 25,000
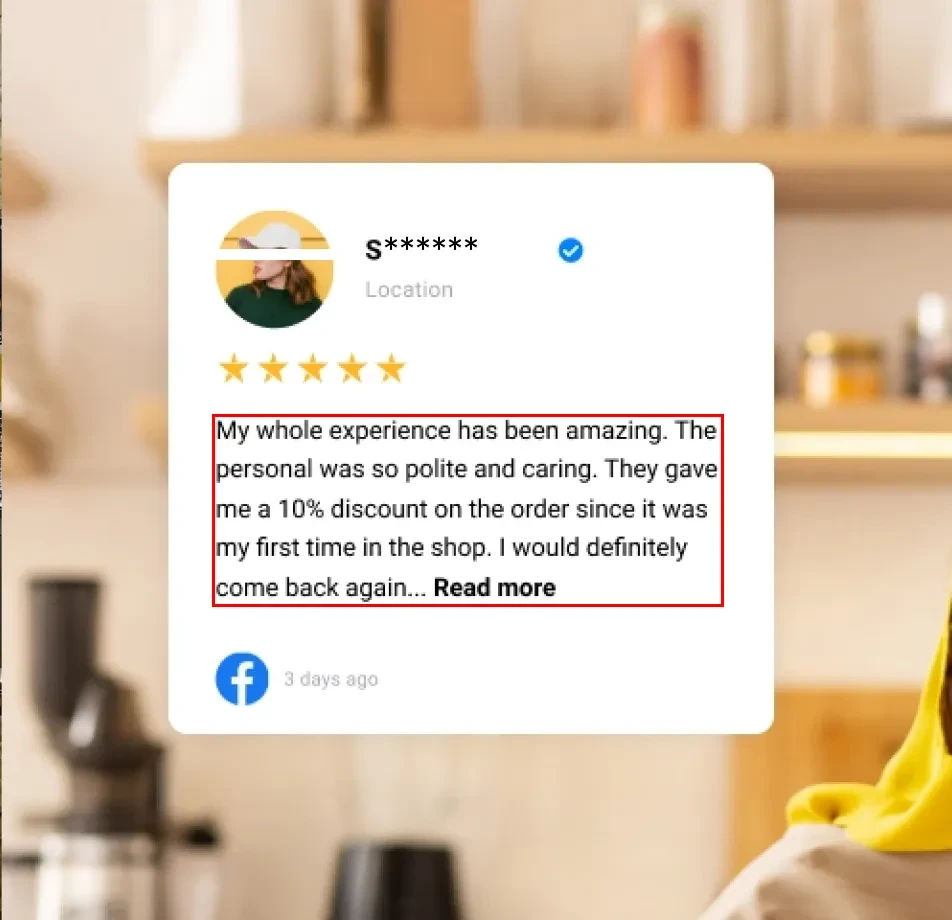
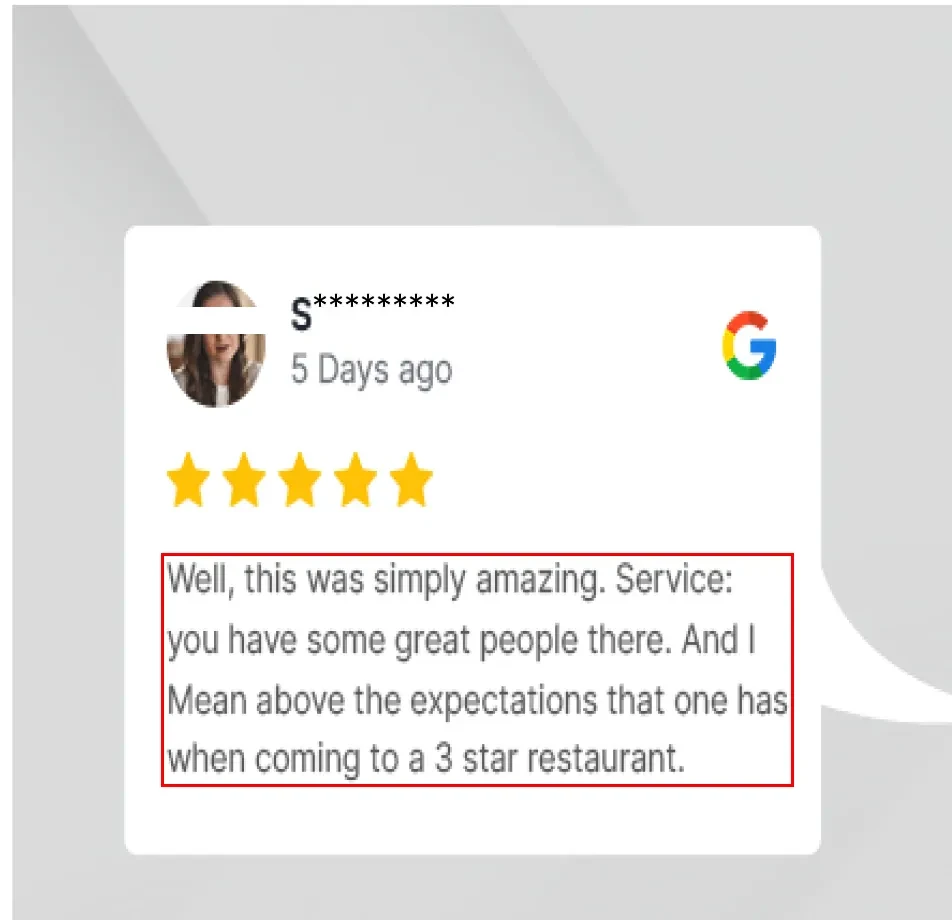
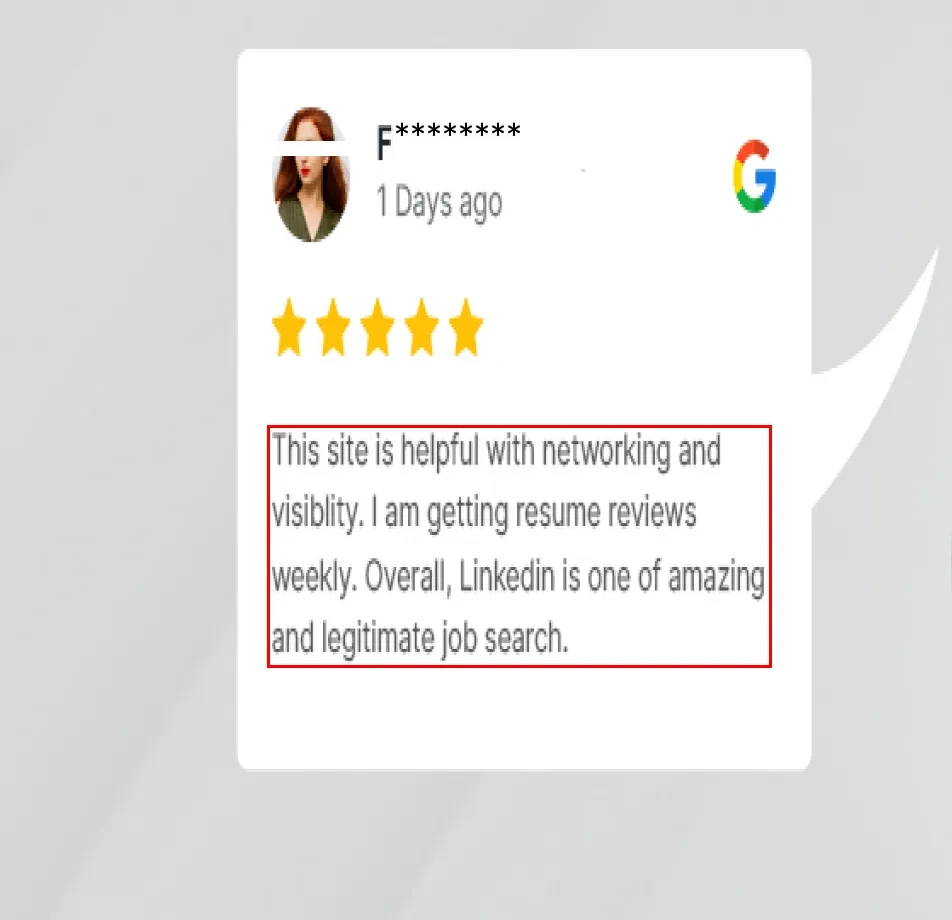
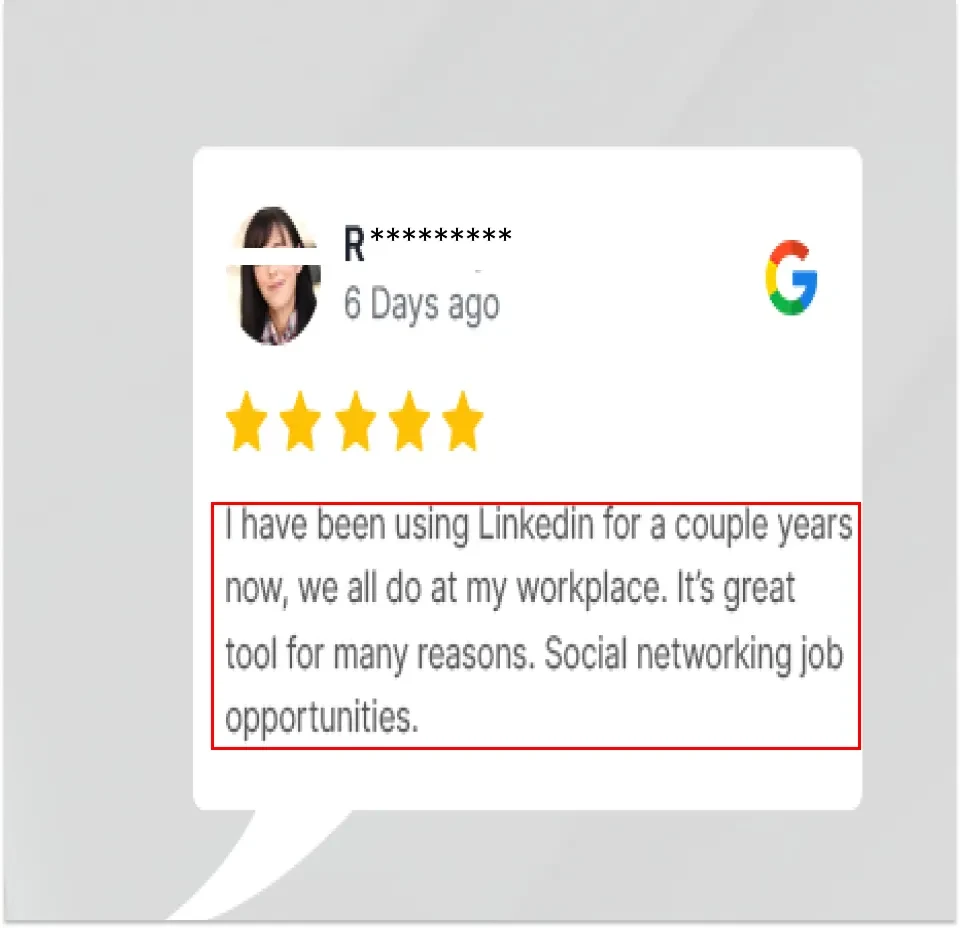
Quality Assurance
Stages
Annotation Verification: Implement a validation process involving sentiment analysis experts to review and verify the accuracy of emotion labels assigned to customer feedback.
Data Quality Control: Ensure the removal of spam or irrelevant entries from the dataset.
Data Security: Protect sensitive customer information and maintain data privacy.
QA Metrics
- Annotation Validation Cases: 2,500 (10% of total)
- Data Cleansing: Remove spam or irrelevant entries
Conclusion
The “Emotion Detection in Customer Feedback” dataset is a valuable resource for businesses seeking to understand customer sentiment and improve their products and services. With a diverse collection of accurately labeled customer feedback entries, along with comprehensive metadata, this dataset empowers companies to analyze customer emotions, identify trends, and make data-driven decisions to enhance customer satisfaction and loyalty. It provides a solid foundation for developing advanced sentiment analysis models and tools that can revolutionize customer relationship management and product development strategies.

Quality Data Creation

Guaranteed TAT

ISO 9001:2015, ISO/IEC 27001:2013 Certified

HIPAA Compliance

GDPR Compliance

Compliance and Security
Let's Discuss your Data collection Requirement With Us
To get a detailed estimation of requirements please reach us.